Introduction: Why AI is No Longer Optional for Business Growth
Artificial Intelligence (AI) has shifted from being a futuristic concept to an indispensable business tool. As industries embrace digital transformation, AI plays a pivotal role in automating processes, delivering predictive insights, and personalizing user experiences. From chatbots to fraud detection, AI applications are revolutionizing sectors like finance, healthcare, retail, and logistics. However, while off-the-shelf solutions offer plug-and-play functionality, custom AI development cost unlocks deeper value and long-term strategic advantages.
Custom AI vs. Off-the-Shelf AI Solutions
Off-the-shelf AI solutions are pre-built, generic tools tailored to common problems like sentiment analysis or image recognition. They offer quick deployment but limited flexibility. In contrast, custom AI solutions are tailored to specific business workflows, data sets, and objectives. For instance, a bank may need a fraud detection system tailored to its transaction patterns—something a generic tool can’t effectively address.
Custom AI provides:
- Full integration with legacy systems
- Ownership of proprietary models
- Competitive advantage through unique capabilities
- Scalability with evolving business needs
Breaking Down AI Development Costs
Understanding the investment needed for custom AI is critical. Here’s a breakdown of core cost components:
- Data Collection and Preprocessing: High-quality data is the foundation of any AI system. Costs include data acquisition, cleaning, labeling, and storage.
- Model Development: This includes algorithm selection, experimentation, and tuning. Costs vary based on the complexity of the problem.
- Infrastructure: Training AI models requires powerful computing resources—GPUs, cloud infrastructure, or on-premise clusters.
- Talent: AI projects demand expertise in data science, software engineering, DevOps, and domain knowledge.
- Maintenance: Continuous model retraining, monitoring, and updating are essential to ensure consistent performance.
Estimated ranges (2025):
- MVP-level AI: $30,000 – $80,000
- Mid-range complexity: $100,000 – $250,000
- Enterprise-grade solutions: $300,000 and above
Understanding the ROI of Custom AI Development
AI investments can yield significant returns when aligned with business goals. ROI comes from:
- Automation: Reducing manual work (e.g., invoice processing)
- Predictive Analytics: Better forecasting (e.g., inventory management)
- Customer Engagement: Personalized recommendations and faster service
For example, an eCommerce business deploying AI-powered search and product recommendations may see a 15–25% increase in conversion rates.
Metrics to Evaluate ROI:
- Cost savings (labor hours, error reduction)
- Increased revenue (personalization, upselling)
- Productivity gain (faster decision-making)
Building a Business Case: How to Justify AI Investment to Stakeholders
To get buy-in from leadership, you need a compelling business case that links AI development costs with strategic goals.
Steps:
- Identify a high-impact use case (e.g., churn prediction, smart routing)
- Forecast potential gains and cost savings
- Present a clear timeline: development, deployment, scaling
- Highlight risks and how they’ll be mitigated
- Propose a phased approach for ROI visibility
Tools: Use case-specific ROI calculators, proof of concept demos, and competitor benchmarking to strengthen the case.
Competitive Edge Through Custom AI Capabilities
Custom AI is not just about efficiency—it’s about differentiation. With unique models and domain-specific insights, businesses can outpace competitors.
Examples:
- Manufacturing: Predictive maintenance models reduce downtime
- Retail: Dynamic pricing engines tailored to real-time demand
- Healthcare: AI for personalized treatment recommendations
Moreover, custom AI ensures that your data—and the intelligence derived from it—remains proprietary, fueling future innovation.
Cost-Effective Strategies for Custom AI Development
Custom AI doesn’t have to break the bank. Here are strategies to optimize your spending:
- Start Small: Build a Minimum Viable AI (MVA) to validate assumptions
- Use Pre-trained Models: Leverage open-source models like BERT, GPT, or YOLO
- Cloud Platforms: Use AWS SageMaker, Google Vertex AI, or Azure ML to reduce infrastructure costs
- Outsource to Experts: Partnering with a seasoned AI development company can bring technical depth and cost-efficiency
Risks, Challenges, and How to Mitigate Them
AI development has inherent risks, including:
- Cost Overruns: Due to changing scope or poor planning
- Bias and Ethics: Flawed training data can cause unfair or inaccurate outcomes
- Data Privacy: Compliance with GDPR, HIPAA, and other regulations
- Model Drift: AI models degrade if not updated with fresh data
Risk Mitigation Checklist:
- Define project scope clearly
- Use diverse training datasets
- Involve domain experts in model design
- Establish governance and audit mechanisms
Future-Proofing Your AI Investment
To sustain value from AI, businesses must prepare for continual evolution:
- Adaptive Learning Systems: Enable models to evolve as data changes
- AI Ops: Automate monitoring and management of AI in production
- Ethical AI: Build trust through transparency, explainability, and fairness
- Data Strategy: Establish scalable data pipelines and storage solutions
FAQs: In-Depth Answers to Common Business Concerns
- How much does custom AI development cost in 2025? Costs vary based on complexity, data availability, and industry. For basic solutions, expect $30,000–$80,000. Enterprise-grade AI involving deep learning or NLP can exceed $300,000.
- What’s the ROI timeline for custom AI projects? Most businesses start seeing ROI within 6–18 months, depending on the use case. For instance, a predictive maintenance system may reduce operational costs within a year.
- Can small businesses afford custom AI development? Yes. SMBs can start with low-cost, open-source tools or collaborate with startups and academic institutions. Building a pilot or prototype (MVA) keeps initial costs manageable.
- What factors increase AI development costs unexpectedly? Unexpected increases stem from poor data quality, scope creep, underestimation of infrastructure needs, and integration with legacy systems. Mitigation starts with good planning and stakeholder alignment.
- How to calculate Total Cost of Ownership (TCO) for AI systems? TCO includes development, deployment, maintenance, cloud infrastructure, human monitoring, and upgrades. It’s essential to account for hidden costs like downtime or retraining needs.
- Should we build AI in-house or hire an AI development company? In-house is ideal if you have the talent and long-term needs. Otherwise, outsourcing offers faster time-to-market, access to skilled experts, and cost efficiency. A hybrid model (internal ownership + external execution) is often optimal.
- How do I ensure my AI model remains cost-effective over time? Implement regular retraining schedules, monitor model performance (AI Ops), and optimize cloud usage. Automate processes where possible and stay updated on the latest ML frameworks to reduce long-term costs.
Conclusion
Investing in custom AI development is a strategic move that can drive business innovation, efficiency, and a sustainable competitive edge. While upfront AI development costs can be significant, the long-term value, especially when aligned with core business objectives, is immense. By understanding the true cost drivers, planning for ROI, and mitigating risks, businesses can harness the full power of AI to lead in their industries.
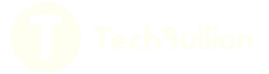