In today’s world, anomaly detection is an urgent task of information technology. This applies to absolutely all areas of society, from finance, e-commerce, healthcare, or insurance. Anomaly detection allows us to identify outlier records if we set a starting point that similar data units in the same data set are relatively homogeneous. This is very important for cybersecurity and information leak prevention.
Given the above, it is unsurprising that the anomaly detection method is quite common in various industries. Taking care of cybersecurity and data security is a priority for most large companies and holdings. Below we will look at the main content of the anomaly detection method in more detail.
What is an anomaly?
In our understanding, an anomaly is a value that represents a significant degree of deviation from the norm. An anomaly is often a rare occurrence and has unique to this particular case qualities. Anomaly detection systems are usually set up to isolate anomalies from the main data set. Thus, the detection process involves establishing patterns and then identifying the units that violate these patterns. Wish to get a more detailed and professional explanation – check this post by Serokell, I got a lot of information there.
Thus, according to the above definitions, anomalies can include anything that is out of the norm and substantially out of sync with the normal value characteristic of a particular data set. A data unit is often too large or too small compared to the bulk of the data. With technology capable of effectively detecting outliers, “abnormal” units stand out firmly during visualization against the bulk of the data set.
Despite the development of technology, detecting anomalies in practice may not always be a simple task. The matter is that in view of the uniqueness of each anomaly, it often turns out to be impossible to detect it the first time. Experts divide anomalies into global and local. As a rule, the first of them is detected quite easily, even with a superficial data diagnosis. Local anomalies, microclusters of deviated data, are often challenging to solve. Their rapid detection, as a rule, turns out to be beyond the power of most modern programs.
Why Is it Important to Detect Anomalies?
Any cyber system operates within a strictly defined framework. The appearance of minor anomalies can serve as an alarm signal for security personnel.
Of course, the appearance of anomalies is a clear signal of the presence of serious problems. The appearance of anomalies in a computer network is also quite common.
Any abnormal traffic patterns found in it may be a sign of a leak of sensitive data from a hacked computer. Thus, as can be seen, error detection can be helpful in various industries and areas, helping professionals quickly and efficiently detect the norm.
Let’s look at some of the most striking ways to detect anomalies:
Cyber intrusion
Cybersecurity can be best achieved with Network Behavior Anomaly Detection (NBAD) technologies. The system finds deviations and signals about the occurrence of threats as a result of a comprehensive analysis of the packet signature. In this case, all data that could pose a threat are subjected to blocking.
Fraud
Using graphs (GBAD) to deal with emerging errors allows you to effectively resist fraud with bank cards and insurance. Machine learning systems help commit fraud in online banking using behavioral biometrics. Which, in turn, can be used to detect and eliminate anomalies.
Counteraction to medical anomalies
There are many ways to deal with errors in a clinical setting. For example, density-based clustering is commonly used when analyzing a medical journal with patient data. An analysis of the patient’s medical history is performed to determine if a particular patient’s service flow trace is abnormal. Anomaly detection in medicine allows for a detailed diagnosis of the disease.
Control detection
Supervised detection is used with fully labeled training and test datasets. This method is most suitable for dealing with and labeling imbalanced data classes. However, it will work well, provided the system has successfully detected the anomalies. Thus, it is not applicable in cases where outliers have not yet been determined.
Semi-supervised discovery is also capable of using training and test datasets. However, in this case, they will be devoid of anomalies. This approach assumes that the system will calculate an anomaly as soon as a normal data set is scanned and undesirable deviations are detected.
Design the anomaly detection system
If you need professional support in designing your secured solution with various settings suitable for many purposes and works with different data sets, take advantage of experienced machine learning developers to create your software solution.
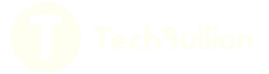