Financial decision-making is undergoing a transformation, thanks to artificial intelligence (AI) and its rapid advancements in predictive modeling. Iswarya Konasani, an expert in AI-driven risk management, explores innovations, shedding light on how predictive analytics and machine learning are redefining financial strategies. This article delves into core advancements in AI-based risk assessment and their implications.
Redefining Risk Assessment with AI
Traditional risk assessment methods relied on historical data and human judgment, leading to inefficiencies and inaccuracies. AI-driven systems have redefined this landscape by integrating real-time data analytics and machine learning models. These systems have demonstrated a 73.8% improvement in risk prediction accuracy compared to conventional statistical models, processing vast amounts of data with unmatched precision.
The ability to identify patterns and correlations has revolutionized credit scoring and underwriting. Advanced AI models analyze thousands of data points per loan application, achieving predictive accuracy rates of over 90%. As a result, financial institutions have reduced credit losses and improved lending decisions, making financial services more accessible.
Fraud Detection and Early Risk Identification
Fraudulent activities pose a challenge to financial institutions, leading to substantial losses. AI-driven fraud detection systems have improved fraud identification rates while reducing false positives. With real-time monitoring, AI algorithms detect anomalies in transaction patterns and flag potential risks with up to 94.3% accuracy.
These advancements have led to an 80.1% reduction in loan processing times, enabling financial institutions to make informed decisions faster. AI-powered early warning systems predict potential defaults an average of 42 days in advance, allowing lenders to take preventive measures.
Enhancing Compliance and Regulatory Oversight
Regulatory compliance has traditionally been labor-intensive. AI-driven solutions have streamlined processes, reducing compliance-related incidents by 72.4%. Machine learning models process thousands of compliance rules simultaneously, ensuring institutions adhere to evolving regulations.
Automated compliance systems have also led to a 48.6% decrease in manual review requirements, enabling regulatory teams to focus on strategic oversight. These improvements have enhanced transparency while minimizing errors, contributing to robust financial governance.
Portfolio Optimization and Market Adaptability
Managing investment portfolios in volatile markets requires rapid adaptability. AI has emerged as a game-changer in portfolio optimization by enabling real-time risk assessments. Reinforcement learning models now enhance portfolio performance by 41.8%, allowing investors to adjust strategies dynamically based on market conditions.
Market adaptability is further reinforced by AI’s ability to analyze thousands of market variables in real time. With high-frequency data processing, these systems identify emerging risks with 87.9% accuracy, ensuring investment strategies remain resilient.
The Role of Alternative Data in Financial Inclusion
One of AI-driven risk management’s profound impacts is its role in expanding financial inclusion. Traditional credit assessment models often exclude individuals with limited credit histories. AI addresses this gap by incorporating alternative data sources, such as mobile phone usage and digital transaction patterns, into credit evaluations.
By leveraging these unconventional data points, financial institutions have increased lending to underserved populations by 43.9%, while maintaining risk parameters. This shift has enabled millions to access financial services that were previously beyond reach, fostering greater economic participation.
Quantum Computing: The Next Frontier
Looking ahead, quantum computing is set to revolutionize AI-driven risk management. Early trials suggest quantum-powered risk analysis could process complex financial scenarios 12,000 times faster than existing AI models. This breakthrough will enable institutions to handle millions of variables simultaneously, optimizing risk assessment with accuracy.
Quantum computing’s potential extends to fraud detection and scenario analysis. These systems are expected to identify market risks up to 127 days earlier than traditional models, providing financial institutions with valuable foresight. As this technology matures, it will redefine financial risk management.
In conclusion, AI-driven risk management is not just an enhancement to existing financial practices, it is a paradigm shift. From fraud detection and compliance to credit scoring and market adaptability, AI has reshaped financial institutions. As innovations in machine learning and quantum computing evolve, the future of financial decision-making will be characterized by greater accuracy, efficiency, and inclusivity. Iswarya Konasani’s research highlights the transformative power of AI, offering a glimpse into a future where financial risk is managed with intelligence.
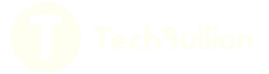