The intersection of engineering management and enterprise data leadership is evolving under the weight of AI and machine learning. Organizations that once relied on fragmented data strategies now recognize that success depends on modern data platforms capable of supporting advanced analytics, scalable engineering solutions, and real-time business intelligence. AI is no longer an experimental feature, it’s the backbone of decision-driven enterprises.
Engineering leaders are no longer just managing teams; they are orchestrating intelligent workflows that extract value from complex data ecosystems. AI and machine learning aren’t simply about automation, they enable engineering managers to make strategic decisions based on predictive analytics, anomaly detection, and optimization algorithms.
“AI-powered analytics is reshaping how engineering leaders think about scalability, governance, and operational efficiency,” says Soumya Banerjee, a leader in enterprise data strategy with nearly two decades of experience spanning Google, AWS, HBO, and IBM. At the Google Next ’25conference, where he was a featured speaker, Banerjee emphasized the importance of reducing data friction across complex architectures. “The challenge isn’t in accessing data, it’s in managing its complexity and ensuring decision-makers have the right insights at the right time.”
Banerjee has also observed this shift in the media and entertainment industry, where AI-driven data strategies helped platforms like HBO Max streamline content recommendations and audience analytics. The rise of streaming services has underscored the necessity of AI-powered decision-making at scale, influencing how engineering teams optimize data pipelines and content delivery.
This shift demands a new breed of engineering leadership, one that understands both data engineering and strategic management, bridging the gap between raw analytics and actionable intelligence. Without this, AI remains a powerful but untapped asset.
Building Intelligent, Scalable Data Platforms
Legacy data architectures struggle to meet the growing demands of AI-driven decision-making. Enterprise leaders are moving towards modern data platforms that support real-time processing, self-optimizing data pipelines, and automated governance.
One key challenge is balancing scalability and security. As enterprises ingest massive datasets, traditional data warehousing approaches fall short. Instead, organizations are adopting hybrid architectures that integrate cloud-native storage, edge computing, and federated learning models.
Banerjee, whose scholarly paper titled The Role of GenAI in Enhancing Data Security and Analytics in Modern Software Development, highlights it as an example of how enterprises are rethinking database solutions. This innovation merges relational and graph database capabilities, enabling cross-functional AI applications without requiring standalone graph databases. The ability to unify structured and unstructured data within a scalable cloud-based environment is proving essential for enterprises managing vast data ecosystems.
“We’re seeing a clear industry shift toward decentralized data platforms that empower domain-specific teams without creating silos,” Banerjee notes. “It’s about enabling high-performance analytics while maintaining strong governance over enterprise data.”
From Data Engineering to Visual Analytics: The New Competitive Edge
Raw data, no matter how well-managed, is useless without effective visualization and interpretation. Enterprise leaders are prioritizing visual analytics to make complex AI-driven insights accessible to stakeholders across departments.
Modern AI-powered visualization tools can process terabytes of information in seconds, translating dense statistical models into intuitive dashboards. This isn’t just about making data more digestible, it’s about accelerating decision cycles and enabling proactive enterprise strategy.
“We’re moving beyond static reports,” Banerjee, who was a speaker at SQLSaturday sharing insights on Dimensional Modeling, explains. “Interactive, AI-driven visual analytics allow leaders to explore scenarios, detect patterns, and react instantly. In a competitive environment, this agility is non-negotiable.”
Redefining Enterprise Data Leadership
The role of enterprise data leadership is no longer confined to data governance and compliance, it now extends to engineering strategy, AI adoption, and cross-functional decision intelligence. Leaders must navigate challenges such as bias in AI models, ethical AI deployment, and the need for transparent data policies.
Successful enterprise data leadership requires an alignment between AI engineers, data scientists, and business executives, ensuring AI investments translate into measurable business outcomes. It’s no longer enough to implement machine learning models; organizations must operationalize AI so that insights drive action.
The Future of AI-Driven Enterprise Engineering
The next decade will see AI further embedded into engineering management and enterprise data ecosystems. From self-healing data pipelines to AI-powered project management tools, the focus will shift from AI as an analytical tool to AI as a real-time operational asset.
Banerjee points out that companies like Google, AWS, and HBO Max have already demonstrated the power of AI-driven enterprise engineering. AI is helping them optimize everything from content recommendation algorithms to cloud-native database management. Organizations that fail to evolve their engineering and data leadership frameworks risk falling behind.
The future belongs to those who integrate AI-driven decision-making, scalable data platforms, and advanced visual analytics, turning data into a strategic advantage rather than an operational burden.
“Enterprise data leadership isn’t just about managing data anymore, it’s about harnessing AI to shape the future of engineering, operations, and strategy,” Banerjee concludes. “Companies that recognize this shift will be the ones setting the industry standard.”
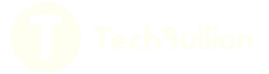