A decade ago, machine learning was a specialty learned by the elite few. However, businesses have now embraced it, rolling its algorithms across various projects via easy-to-use machine learning platforms.
Machine learning is a cognitive shift from earlier restrictive programming techniques, and its transformative powers are now building formidable applications. Its use cases range from product recommendations, image recognition, and self-driving cars to medical diagnoses.
The machine learning market size could scale to $209 billion by 2029 from its current $21 billion value. You, too, can partake of this massive opportunity by accelerating your data processing and workflow ML automation. Optimize any related data functionalities via these machine learning platforms below.
Qwak
Qwak is a pain-free solution to all your in-production machine learning needs. It is a fully managed data operations and machine learning engineering platform that supports large-scale AI models’ continuous productization.
Qwak will function seamlessly as an in-house machine learning engineering platform, neutralizing friction between IT teams and data scientists. In addition, its ML productization process is so streamlined that your teams can kick off their ML creation process without a single line code. This feature will save your data scientists valuable infrastructure setup time.
Some formidable ML teams leveraging Qwak in their model builds are JLL, Yotpo, and Guesty. Its features include an off-the-shelf data lake accessible via the Qwak SQL interface. On the other hand, its automation feature eases production environment model surveillance via triggers and alerts.
KNIME
KNIME, or the Konstanz Information Miner, is an open-source machine learning platform. Its open architecture and zero license fee features make it perfect for hobbyists and beginners that need a data analytics and integration platform.
It has various data mining and machine learning components and a low code interface that lowers ML project creation complexity for non-data scientist teams. KNIME integrates with Amazon Web Services (AWS)and Azure, but its Python and R Code-based scripting base may hinder ML model customization. It is also computational resource-heavy and does not offer a data lake as Qwak does.
Anaconda
Anaconda is an open-source, easy-to-use platform that offers various commercial bandwidths and packages. It is a good choice for freelancers or small businesses that want to dip their toes in machine learning waters.
Its free Individual Edition opens access to a wide variety of dataset exploration and modeling tools. However, Anaconda’s custom repositories are only available to its Enterprise Edition users.
IBM Machine Learning
Like KNIME, IBM Machine Learning is open source. Its best features include drag and drop data preparation features. Additionally, it supports analytics on unstructured data, and its API is easy to use.
It is, however, a clunky platform, opening all its services in separate tabs. The IBM Machine Learning integrates with the IBM Cloud Pak, IBM Watson Studio, and the IBM Watson OpenScale.
Google Vertex AI
Google Vertex AI is a low-code ML platform. It offers an easy-to-use no-code point-and-click model optimization and data science for the less skilled programmer. That said, it can scale to user programming skill levels supporting the professional data scientist’s need for a code-based machine learning experience.
This machine learning platform provides a unified and comprehensive user experience and top-notch out-of-the-box model algorithms. But, overall, Google Vertex AI is ill-suited for businesses that may opt for hybrid cloud deployments.
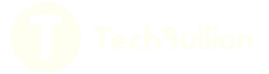