Introduction:
In the dynamic realm of Artificial Intelligence (AI), two prominent methodologies stand out: supervised learning and unsupervised learning. These approaches serve as the bedrock for training machine learning models, each offering distinct advantages and applications. In this exploration, we will unravel the intricacies of supervised and unsupervised learning, shedding light on how these approaches shape the landscape of AI.
Understanding Supervised Learning:
Supervised learning is akin to a guiding hand, where the model is trained on labeled data—input paired with corresponding output. The objective is for the algorithm to learn the mapping between inputs and desired outputs, making predictions or decisions without explicit programming. This approach is analogous to a teacher providing examples and guiding a student toward the correct answers.
In supervised learning, the training dataset acts as a blueprint for the model, allowing it to learn from historical data and generalize to new, unseen data. The process involves feeding the algorithm with labeled examples, enabling it to make predictions or classifications based on the patterns it learns during training.
Accuracy and Precision:
The Benchmarks of Supervised Learning:
The success of supervised learning hinges on the accuracy and precision of the model’s predictions. Accuracy refers to how well the model performs in making correct predictions, while precision measures the consistency of these predictions concerning the defined criteria.
Supervised learning is prevalent in tasks that require precise outcomes, such as image recognition, speech-to-text conversion, and sentiment analysis. By training on labeled data, the model refines its ability to make accurate predictions in real-world scenarios.
Transitioning to Unsupervised Learning:
In contrast, unsupervised learning takes a more exploratory path. Here, the algorithm is presented with unlabeled data, and its task is to uncover inherent patterns, relationships, or structures within the information. Think of it as a student exploring a dataset without predefined answers, seeking to identify hidden insights independently.
Clustering and Dimensionality Reduction:
Unveiling Patterns in Unsupervised Learning:
Unsupervised learning techniques are often employed in tasks such as clustering and dimensionality reduction. In clustering, the algorithm groups similar data points together based on intrinsic similarities, revealing natural clusters within the dataset. Dimensionality reduction, on the other hand, focuses on simplifying complex datasets by extracting essential features and reducing the overall number of variables.
One common algorithm used in unsupervised learning is the k-means clustering algorithm, which segments data into k clusters based on similarity. Another notable technique is Principal Component Analysis (PCA), a dimensionality reduction method that identifies and retains the most critical features in a dataset.
Anomaly Detection:
Identifying Outliers in Unsupervised Learning:
Unsupervised learning also excels in anomaly detection, where the algorithm identifies patterns that deviate from the norm. This is particularly valuable in detecting fraudulent activities, network intrusions, or any irregularities within a system.
The Lack of Labels:
Challenges and Advantages:
While unsupervised learning offers a more exploratory and flexible approach, it comes with its set of challenges. The lack of labeled data means that the algorithm must rely on intrinsic patterns within the data, making it more susceptible to noise and subjective interpretations. However, this very lack of labels also grants unsupervised learning a unique advantage—its applicability to datasets where labeling is impractical or expensive.
Semi-Supervised Learning:
Bridging the Gap:
In the realm of machine learning, a middle ground between supervised and unsupervised learning exists: semi-supervised learning. This hybrid approach combines a small amount of labeled data with a more extensive pool of unlabeled data. The model initially learns from the labeled examples and then generalizes its knowledge to the unlabeled data.
Semi-supervised learning is particularly useful when acquiring labeled data is challenging or costly. It leverages the benefits of both approaches, offering the precision of supervised learning while tapping into the flexibility and scalability of unsupervised learning.
Real-World Applications:
Where Each Approach Shines:
The choice between supervised and unsupervised learning often depends on the nature of the task at hand. Supervised learning finds its forte in scenarios where precision and accuracy are paramount. Common applications include:
Image and Speech Recognition:
Training models to recognize specific patterns or phonetic features.
Predictive Analytics:
Making predictions based on historical data, such as stock price forecasting or sales predictions.
Natural Language Processing (NLP):
Enabling machines to understand and generate human language.
On the other hand, unsupervised learning thrives in scenarios where the goal is to explore, discover, or uncover hidden patterns. Key applications include:
Customer Segmentation:
Grouping customers based on shared characteristics for targeted marketing.
Anomaly Detection:
Identifying unusual patterns or outliers in datasets.
Recommendation Systems:
Uncovering patterns in user behavior to make personalized recommendations.
Hybrid Approaches and Ensemble Learning:
In the pursuit of optimizing model performance, practitioners often turn to hybrid approaches and ensemble learning. Hybrid models may combine aspects of both supervised and unsupervised learning, allowing for a more nuanced understanding of complex datasets.
Ensemble learning, on the other hand, involves combining multiple models to achieve better performance than any individual model. This can include a mix of supervised and unsupervised models, each contributing its unique strengths to the overall ensemble.
Challenges and Future Directions:
While supervised and unsupervised learning have proven their mettle in various applications, challenges persist. Supervised learning relies heavily on the availability of labeled data, which can be a bottleneck in scenarios where obtaining labels is resource-intensive. Unsupervised learning, while more flexible, often requires sophisticated algorithms to extract meaningful patterns from unlabeled data accurately.
The future of AI and machine learning may see the convergence of these approaches, with advancements in semi-supervised and unsupervised learning techniques addressing the challenges associated with labeled data scarcity. Additionally, the ethical implications of AI algorithms, including issues related to bias and fairness, continue to be at the forefront of research and development efforts.
Conclusion:
In the grand tapestry of Artificial Intelligence, the threads of supervised and unsupervised learning weave a narrative of innovation and exploration. Supervised learning, with its labeled datasets and precision-driven outcomes, stands as the cornerstone of tasks demanding explicit guidance. Meanwhile, unsupervised learning ventures into uncharted territories, uncovering hidden patterns within unlabeled data and providing invaluable insights into complex systems.
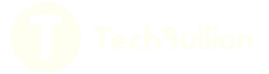