In today’s digital era, the demand for real-time data insights is surging, driving organizations to innovate with advanced technologies. A key transformation is happening in data warehouse management, where automation technologies are simplifying complex multi-cloud ETL workflows. Artificial intelligence (AI), machine learning (ML), and advanced orchestration tools are enhancing operational efficiency and data quality. This article, by Lakshmi Ayyappan, explores these innovations and highlights the strategies reshaping the future of real-time data processing and analytics.
The Evolution from ETL to ELT: Revolutionizing Data Processing
A major shift in data processing is the transition from Extract, Transform, Load (ETL) to Extract, Load, Transform (ELT). This evolution, adopted in recent years, processes data directly in the cloud, significantly speeding up data pipelines and reducing the complexities of traditional ETL workflows. By decoupling storage and compute resources, ELT enables organizations to scale efficiently, processing terabytes of data within minutes rather than hours or days. The benefits are clear: businesses implementing ELT have experienced up to a 60% reduction in pipeline development time and can achieve sub-second query response times, essential for real-time data analysis in critical industries.
Automation in Data Warehouse: Enhancing Efficiency and Reducing Complexity
Data warehouse automation has advanced to manage growing data volumes and processing needs. With Change Data Capture (CDC) systems, businesses can process millions of transactions per second with minimal latency, reducing manual tasks by up to 70%. AI and ML play a key role by optimizing workflows and predicting bottlenecks, enhancing operational efficiency. These systems not only automate processes but also improve real-time analytics, making data-driven decision-making more reliable and accessible.
Security, Governance, and Compliance in Multi-Cloud Workflows
Managing security and compliance in multi-cloud environments is inherently complex. However, automation frameworks that integrate policy enforcement, identity management, and compliance monitoring effectively address these challenges. Organizations adopting these systems have reported a 75% reduction in security incidents and a 50% decrease in audit preparation time. These advancements not only ensure strong data protection but also help businesses meet regulatory data privacy and security requirements, maintaining compliance and reducing risk.
Optimizing Data Pipelines with AI and Machine Learning
As data systems grow in complexity, efficient pipeline architectures are crucial. AI integration optimizes resource allocation, query performance, and bottleneck prediction, improving system performance by 65% and reducing costs by 40%. Additionally, low-code platforms allow business analysts and data scientists to build sophisticated pipelines, boosting cross-functional collaboration and accelerating time-to-market for data-driven initiatives.
Improving Data Quality and Governance Through Automation
Maintaining high-quality data is one of the biggest challenges faced by modern enterprises. Automation in data profiling, validation, and cleansing has transformed how organizations manage data integrity. By employing machine learning algorithms, these automated systems can detect anomalies and inconsistencies, reducing data quality incidents by 70%.
Furthermore, AI-driven data governance solutions are helping businesses adhere to compliance standards more effectively. Automated validation and monitoring systems now ensure that data handling processes are continuously checked against regulatory requirements, with some organizations reporting a 75% reduction in compliance-related incidents.
The Road Ahead: Preparing for the Future of Data Warehouse Automation
Looking toward the future, the convergence of quantum computing, edge processing, and further advancements in AI will continue to revolutionize the data warehouse landscape. AI and machine learning will further reduce manual data management efforts, improve operational efficiency, and enhance data quality.
Enterprises are advised to build flexible architectures that can easily adapt to these emerging technologies, ensuring that they remain competitive in an increasingly data-driven world. By focusing on automation, businesses can not only enhance their data capabilities but also ensure that they are well-positioned to navigate the evolving challenges of the digital age.
In conclusion, data warehouse automation has a transformative impact, driving improvements in data processing efficiency, security, and governance through AI and ML-powered frameworks. As technology continues to evolve, adopting advanced automation tools is vital for staying competitive. For businesses aiming to thrive in the data-driven economy, prioritizing automation is crucial. This article by Lakshmi Ayyappan outlines key innovations in automation, providing valuable insights into how organizations can leverage these technologies to streamline operations and achieve their strategic objectives.
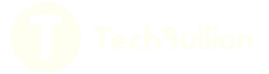