In a significant step toward restoring America’s semiconductor leadership, the U.S. Department of Commerce has announced $1.4 billion in new awards under the CHIPS National Advanced Packaging Manufacturing Program (NAPMP). The goal is to establish a domestic ecosystem where the most advanced chips are designed, fabricated in the United States, and packaged at scale. This shift is crucial for sectors like autonomous vehicles, defence systems, and AI hardware, where every layer of the chip stack must be reliable, secure, and production-ready.
However, while the spotlight often falls on design and computing power, another critical discipline ensures these chips are safe to deploy: Design for Testability (DFT). As chips grow more complex, testing and validation have become technically and geopolitically strategic priorities. To understand what’s changing behind the scenes, we spoke with Vijayaprabhuvel Rajavel, a Technical Architect at HCL America, who leads DFT for custom complex, high-performance ASICs in automotive and intelligent systems. A Senior Member of IEEE, a Fellow of the Soft Computing Research Society, an Exemplary Initiate of Epsilon Pi Tau, and the creator of ExploreDFT, he shares how testability impacts chip trust, safety, and innovation, particularly when lives are at stake.
Vijayaprabhuvel, given the recent CHIPS Act funding, how does DFT factor into this new ecosystem, where design, manufacturing, and packaging are entirely within the U.S.?
The CHIPS Act is reshaping how the U.S. approaches semiconductor development by bringing all primary phases—design, fabrication, and packaging—into a single national framework. Design for Testability becomes essential in that environment to ensure each chip leaving the line is functionally sound, traceable, and production-ready at scale. As advanced packaging techniques, such as chiplets and 3D integration, gain traction, the role of DFT becomes increasingly important. It must account for new interconnect paths, thermal variations, and integration-related failure modes that traditional test flows were not designed to handle. At the IEEE Congressional Visits Day (CVD) 2025 in Washington, D.C., I directly engaged with the Senators, House Representatives, and their staff to discuss technology policies, reinforcing DFT’s critical role in national technology strategies.
DFT also centrally enables data continuity across the manufacturing pipeline. With manufacturing and packaging localised, there’s a greater opportunity to correlate test data with layout, process variations, and system-level behaviour. DFT architectures designed with this in mind can unlock faster yield learning and more effective root-cause analysis, particularly in safety-critical domains such as defence and automotive.
You were Alphawave Semi’s first DFT hire in the U.S., leading the productization of DFT IP and shift-left scan strategies. How do the DFT challenges in complex, high-performance Application Specific Integrated Circuits (ASIC) for chiplets and autonomous systems differ from those in more traditional chip designs?
High-performance ASICs for chiplets and autonomous systems demand greater design flexibility and fault coverage than traditional applications. These chips often integrate a combination of high-speed serial interfaces, dense memory arrays, and real-time processing cores, creating a fragmented landscape for scan insertion and coverage closure. In this environment, design-for-test requires a modular, Shift-left, or RTL-aware approach, where each subsystem is treated with tailored strategies to ensure signal visibility and controllability without compromising performance.
In addition, safety and traceability become critical. Faults must be isolated quickly, sometimes even during deployment, and power constraints during tests are stricter due to thermal and long-term reliability concerns. At Alphawave, the emphasis was building scan architectures that could meet these demands—reusable, scalable, and validated across different designs. As the first DFT engineer on the U.S. team, I ensured that the IP was testable, the methodology was reusable, and ready for seamless integration into advanced silicon.
Testing is often viewed as a final step, but how early should testability be integrated into the design process in high-risk applications such as self-driving vehicles or defense?
In mission-critical applications, testability must be considered from the architecture stage, not after the RTL is frozen. The earlier DFT is part of the design conversation, the more seamlessly it can align with performance, power, and area goals, especially in systems where faults can translate into safety violations. Waiting until the back end often leads to compromises, patchwork fixes, or even redesigns when coverage targets or timing budgets aren’t met.
Early integration enables test logic to be co-optimized with functional blocks, improving scan insertion quality and making power-aware strategies more effective. It also opens the door for predictive validation, which involves running testability checks and simulations during early design iterations rather than reacting at the end. In safety-critical domains, this proactive approach is not a luxury but a requirement for meeting regulatory standards and production timelines.
At Cadence, a top-tier EDA company, you led DFT projects that raised scan coverage from 4% to over 95%, earning awards “Creator” and “Explorer” for your impact on customer success. What techniques made that transformation possible in such complex designs?
Improving scan coverage involved aligning the DFT approach with the structure of each design rather than applying a one-size-fits-all method. Many initial gaps came from scan-excluded blocks, inconsistent constraints, or legacy IP integration. We also employed wrapper insertion techniques and strategically placed test points to enhance accessibility and coverage across challenging blocks, resolving synthesis-time constraints early without creating routing or timing issues.
Another focus was automating DRC cleanup and tightening the interface between scan insertion and ATPG pattern generation. This helped avoid late-stage surprises and ensured that patterns were highly covered and compression-friendly. The process was refined across several projects, contributing to debug cycles faster, improved tool stability, and more consistent results in production environments.
At Cadence and Samsung, you worked extensively on addressing power challenges during test operations. How can engineers effectively balance thorough testing with minimizing power consumption in semiconductor designs? How do you balance thorough testing and minimising power consumption in these environments?
Managing power during test mode requires targeting the unique sources of switching activity in scan operations. A primary contributor is uncontrolled toggling across long scan chains, with profound logic and high-density compute units. The switching was contained without reducing coverage by applying toggle-aware pattern generation and integrating clock gating into the scan enable paths.
In addition, analysing power domains and applying localised compression helped distribute test activity more evenly. Certain blocks were isolated during scan insertion, allowing high-activity regions to be managed independently. These adjustments were made early in the flow, which prevented late-stage surprises and ensured that test patterns remained within safe dynamic power limits. Across several implementations, this approach consistently reduced test power without delaying delivery or altering core logic.
Rajavel, you have received prestigious awards, including the Cases and Faces Award, the International Achievers’ Award, and Samsung’s Award of Excellence. What skills will engineers need to stay ahead as AI/ML reshapes the future of DFT?
Engineers with a strong foundation in digital design and testing and good data skills are better prepared for the changing demands of DFT. As new tools and methods are added, like using data to predict faults or improve scan efficiency, understanding the design and the data becomes more important. Knowing how to write scripts, analyze data, and connect different parts of the test process helps create test flows that are smarter and more flexible. DFT is no longer just about hardware—it’s also about working with information in innovative ways.
This interdisciplinary mindset—combining technical depth with a broader view and blending hardware knowledge with software and systems was recognized, for example, when I received the Cases and Faces Award. Selected from over 1000 global applicants, the award is judged by an international jury of experts in engineering, AI, and venture leadership; I was honored in the “Achievement in Product Innovation” category. It was significant because it highlighted engineers who solve complex problems and make a lasting impact. The award reflects how semiconductor design for test engineering is becoming more interdisciplinary, and it encouraged me to keep exploring new ways to improve processes and deliver meaningful results.
As a recognized expert, invited speaker at IEEE VTS 2025, judge for renowned competitions such as the Globee Awards, Innovate 2025, IEEE Arduino Contest and The Tech Challenge, and a reviewer for leading global conferences such as IJCNN, InC4, VLSI SATA, how do you see machine learning shaping the future of DFT?
Machine learning(ML) influences how test flows are designed, executed, and refined. DFT learns from silicon data, predicts fault-prone regions, optimizes test pattern selection, and reduces diagnostic time. These applications are especially valuable in complex, heterogeneous designs where traditional rule-based methods struggle to scale.
Through my judging and reviewing roles, I have seen a clear trend: the best ideas are no longer focused solely on raw coverage or compression, but on adaptability—how test systems respond to real-world variations. ML enables that adaptability. Engineers are beginning to view test data as a resource for continuous improvement, rather than a one-time checkpoint, which shifts the role of DFT toward something more intelligent, iterative, and fully integrated with the entire chip lifecycle.
At the recent VLSI Test Symposium(VTS) held in Arizona, a premier international semiconductor test, validation, and reliability conference, I discussed how machine learning accelerates DFT through predictive pattern generation, adaptive test flow, and generative AI automation, helping accelerate development timelines. In the same session, other leading experts like Sri Ganta, Principal Product Manager from Synopsys, presented TSO.ai on test space optimization, and Bonita, DFX Director from NVIDIA, showcased ChipNemo. This production-grade agentic ecosystem accelerates triage and test debugging using generative AI.
ML is no longer a future concept but a present-day enabler in production-grade DFT workflows. Looking forward, I envision intelligent, self-learning test systems that evolve with silicon behavior, enabling scalable, power-aware, and high-quality testing for advanced nodes and heterogeneous integration. These advancements mark a paradigm shift, turning semiconductor testability from a static process into a dynamic, learning-driven system.
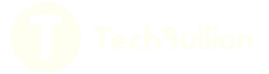