Demystifying Artificial Intelligence in Data Pipelines
As Artificial Intelligence (AI) continues to integrate into various sectors, its capabilities in automating complex processes and driving innovation are unmatched. However, one of the challenges that come with AI is its “black box” nature, wherein the inner workings of machine learning models remain opaque, even to those deploying them. This issue is especially critical in fields like data engineering, where large-scale pipelines manage massive amounts of data that fuel these AI models.
Dr. Mohan Raja Pulicharla addresses this concern in his recent study titled “Explainable AI in the Context of Data Engineering: Unveiling the Black Box in the Pipeline.” His research emphasizes the importance of Explainable AI (XAI) in ensuring transparency and trust in machine learning models, particularly within the intricate frameworks of data pipelines.
Explainable AI: Enhancing Transparency in AI Systems
Explainable AI (XAI) refers to a set of methods and techniques that allow human users to understand how AI systems make decisions. In the context of data engineering, where data must flow seamlessly through various stages of collection, transformation, and analysis, XAI has a significant role to play.
Dr. Pulicharla’s research highlights how traditional AI systems, while effective, often lack transparency. Engineers, decision-makers, and even end-users find it difficult to comprehend why a model arrived at a specific conclusion. This is particularly problematic when AI is used in critical areas such as healthcare, finance, or governance, where decisions can have significant consequences. Without insight into these decisions, AI systems can remain underutilized or mistrusted.
By implementing XAI within data pipelines, engineers can inspect models at various stages of processing. XAI offers a way to verify their accuracy, fairness, and reliability, leading to more informed and confident decision-making.
AI in Data Engineering: Bridging the Gap Between Models and Data
Data engineering involves designing, building, and managing the data infrastructure required for analysis and decision-making in AI systems. As data moves through the various stages of a pipeline—whether it’s being ingested, transformed, or analyzed—AI models are often applied to make sense of that data. Dr. Pulicharla’s research illustrates that these models are only as effective as the transparency they offer.
By integrating XAI into data pipelines, the study focuses on demystifying how machine learning models handle data at each stage. For instance, an AI model used to predict customer behavior may process raw transactional data, apply a series of transformations, and eventually make a prediction. XAI can help engineers and analysts track how the model arrives at its conclusion, ensuring the results are both explainable and reliable.
Dr. Pulicharla emphasizes that XAI can help identify potential biases or inconsistencies in models that may otherwise go unnoticed. Given that data engineering pipelines often handle sensitive data, such as personal information or financial records, explainability is crucial to maintaining ethical AI practices.
The Practicality of XAI in Data Pipelines
One of the key takeaways from Dr. Pulicharla’s research is the practicality of implementing XAI in real-world data pipelines. While XAI is often discussed in theoretical terms, his study delves into the technical aspects of how it can be embedded within existing infrastructure. This involves selecting the right tools and methods for achieving explainability without disrupting the efficiency of data pipelines.
The research outlines how model-agnostic techniques such as LIME (Local Interpretable Model-Agnostic Explanations) or SHAP (Shapley Additive Explanations) can be applied at various points in a data pipeline to interpret and explain model predictions. These techniques can be used to continuously monitor AI systems and detect any anomalies or unexpected behaviors.
In addition to practical implementation, XAI’s potential long-term benefits include fostering trust between AI systems and their users. Whether it’s a business analyst relying on AI-generated insights or a data engineer tasked with managing the pipeline, XAI ensures that the rationale behind each decision is clear, traceable, and defensible.
A Step Forward for Ethical AI:
As AI systems become more prevalent in decision-making processes, the need for transparency and trustworthiness cannot be overstated. Dr. Mohan Raja Pulicharla’s research on Explainable AI within data pipelines contributes to the ongoing efforts to make AI systems more accountable and understandable.
By focusing on the implementation of XAI in data engineering, his work highlights the critical role transparency plays in ensuring AI’s successful integration into industries that depend on reliable data insights. This research points to a future where AI is not just a tool for automation, but also a system that can be fully understood, trusted, and relied upon by its users.
For more details, read the full research article here: https://www.ijisrt.com/explainable-ai-in-the-context-of-data-engineering-unveiling-the-black-box-in-the-pipeline.
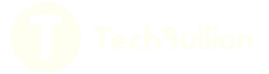