The financial sector has undergone significant transformations in data-handling practices over the past decade. With the advent of big data technologies, banks have transitioned from traditional data processing to more sophisticated, AI-driven analytics. Senior data scientist at the world’s largest custodian bank, Ranjeeta Bhattacharya, has witnessed this evolution firsthand, underscoring the sector’s growing challenge to master the deluge of data while enhancing decision-making and fostering innovation.
Financial institutions often find it overwhelming to handle this influx of data. Bhattacharya now shares some best practices for transforming complex big data into actionable insights that drive growth and customer trust in banking and other financial processes.
Understanding What Data Means
In her career spanning over 15 years, Bhattacharya has worn many hats. These positions range from software developer to solution designer, technical analyst to delivery manager, and project manager. Her multifaceted roles have exposed her to various data types and formats while working with Fortune 500 companies worldwide.
Despite the diversity of data she has encountered, Bhattacharya has consistently emphasised one fundamental principle: data is useless without understanding its meaning. This principle has guided her approach to data handling across different industries and types of data.
Bhattacharya’s extensive experience has taught her that the first and most crucial step in handling data is understanding what they are trying to convey. This understanding is especially critical in the banking and finance sector, where the unprecedented influx of data from diverse sources presents immense opportunities but also significant challenges in terms of data integrity, security, and regulatory compliance. In this context, mishandling data can lead to costly misunderstandings and errors.
“Data is the lifeblood of modern banking,” Bhattacharya mentions. “However, the true value lies not in merely accumulating data but in our ability to harness its potential through advanced analytics and machine learning techniques.”
Approaching Complex Data: Best Practices from the Front Lines
Data encompasses more than just financial transactions and balances or simple handling of finances. It extends deeply into customer service, fraud detection, and operational efficiency.
Each department within a financial institution deals with its own complex and voluminous data sets, each holding the potential to unlock valuable insights and drive strategic decision-making.
Bhattacharya points out that the sheer magnitude and intricacy of this data can be overwhelming, making manual and traditional analysis methods inadequate. This is where the power of Artificial Intelligence (AI) and Machine Learning (ML) comes into play, revolutionizing how banks and financial institutions harness their data.
With her vast experience supporting end-to-end AI/ML solutions from inception to deployment, Bhattacharya experienced firsthand how AI and ML algorithms excel at simplifying complex data structures and extracting meaningful patterns and insights. Her expertise spans diverse use cases, showcasing the versatility and impact of AI and ML in the financial sector.
In the case of customer service, Bhattacharya explains how AI-powered chatbots can analyze vast customer interaction data to identify common queries, sentiment patterns, and preferences. Banks can then tailor their services, offer personalized recommendations, and proactively address customer needs to enhance the overall banking experience.
Similarly, ML models can sift through millions of transactions in fraud detection, identifying anomalies and suspicious activities that might elude human observers. Bhattacharya warns that these intelligent systems allow banks to learn from historical fraud patterns and adapt to new threats, thus staying one step ahead of fraudsters and safeguarding customer assets and trust.
“Financial institutions can make data-driven decisions, improve efficiency, and deliver superior customer experiences by bridging the gap between complex data and practical applications,” Bhattacharya notes. “These tools allow us to process extensive data efficiently, uncovering previously indiscernible patterns.”
Being a Step Ahead
Bhattacharya understands that the effective use of AI in banking extends beyond mere technology adoption; it involves a continuous refinement of the methods used to process and interpret data. This ongoing improvement in data handling strategies ensures that financial institutions are not just reacting to changes but are proactively preparing for them.
For Bhattacharya, one must not merely react to the data at hand but always be ahead, anticipating potential use cases and scenarios and preparing accordingly.
She emphasizes the necessity of building systems that, more than being technically advanced, are versatile and resilient. She advocates for a modular framework in technology deployment, where individual components can be updated or modified without disrupting the overall system.
In the financial sector, where regulatory shifts and market dynamics can alter the landscape with little warning, such agility ensures institutions maintain compliance and service quality, even as external conditions change.
Bhattacharya’s forward-thinking mindset is crucial in this high-stakes sector, where the consequences of mishandling data can be severe and costly.
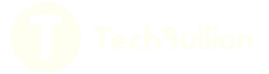