A leading expert in banking transformation and AI-driven financial strategies shares insights on how financial institutions can successfully implement and optimize modern operating models for long-term resilience and growth.
Digital transformation is no longer a buzzword—it’s a strategic imperative reshaping the future of financial institutions. According to a recent PwC report, 22% of financial services organizations now cite digital transformation as their top strategic focus, while 21% highlight the impact of emerging technologies and 20% emphasize the growing burden of regulatory compliance.
At the same time, the adoption of artificial intelligence is accelerating across the industry. A recent study shows that 77% of companies are already using or actively exploring AI in their operations, marking a decisive shift toward automation, real-time analytics, and intelligent decision-making.
All these changes are possible with the help of specialists like Olga Zueva, a leading expert in banking transformation who has spent over a decade reshaping the way global financial institutions operate. She has successfully advised top-tier investment banks, fintech firms, and major retail banks on cost efficiency, AI-driven banking solutions, and large-scale operating model transformations. By bridging financial strategy and technology, she helps institutions optimize performance while ensuring sustainable growth.
In this interview, we explore the key factors behind successful banking transformations and the challenges financial organizations face in adapting to the rapidly evolving market.
Olga, why is the banking system undergoing this change, and what is the core purpose of it?
The banking system is changing because traditional models built on outdated systems, isolated or siloed departments, and rigid processes can no longer keep up with today’s digital economy. Technology and AI are reshaping how banks operate, enabling automation, real-time analytics, and personalized services. At the same time, competitive pressures from fintech disruptors and digital-first institutions are pushing banks to modernize their operating models to remain relevant.
This shift is about making banking faster, smarter, and more responsive. Customers now expect seamless, intuitive experiences similar to those of top tech companies. To compete, banks must redesign their operations, breaking down inefficiencies and embracing agile, data-driven models that support real-time decision-making and personalized financial solutions. Institutions that adapt will not only stay competitive but set new standards for the future of banking.
Most financial institutions are successfully restructuring their operations, yet a third still struggles to see measurable results. As a unique expert who has led multiple large-scale transformations, what do you think separates those who succeed from those who fail?
The successful institutions recognize that transformation isn’t just about technology – it’s about adopting a platform-based operating model that integrates technology, people, and business strategy. While AI, automation, and cost-efficiency measures are critical, the real game-changer is organizational adaptability.
For example, I led a team that implemented a platform operating model at a top U.S. financial institution that restructured how core business functions operated and impacted thousands of employees. The biggest hurdle wasn’t technical; it was helping teams transition from a traditional product-centric structure to a platform-based model that enabled efficiency, flexibility, and integration between business lines. To ensure success, I designed a phased rollout strategy, secured leadership buy-in, and implemented training programs for employees to equip teams with the right capabilities to operate within a platform-based framework.
On the other hand, the projects that struggle tend to underestimate the human factor. You can build the best operating model on paper, but the transformation stalls if the people executing it aren’t fully on board.
This leading financial institution project restructured its operating model, shifting from a traditional functional structure to a platform-driven approach and streamlined operations for thousands of employees. Yet, large-scale transformations often face internal resistance. How did you overcome skepticism and ensure adoption?
Frankly, mid-level managers were among the most resistant to change because platform-based models redistribute decision-making authority, requiring a shift from function-specific control to cross-functional collaboration within platform teams. They could have slowed down or blocked the process if they weren’t engaged early.
To prevent this, I led my team in launching a leadership alignment initiative, giving managers ownership over parts of the transformation so they became champions of the change rather than opponents. In parallel, I insisted on introducing early performance indicators—once teams saw that efficiency was improving without job losses, trust grew.
Another key step was phased implementation. My team tested the new model in specific departments first, refining processes before scaling. When employees saw firsthand how the new operating model enabled teams to work in a more integrated and agile environment, adoption became a natural progression rather than a forced transition.
Still, balancing long-term goals with short-term business continuity is the biggest challenge in any operating model transformation. Success depends on sequencing change carefully, ensuring that new platform-driven ways of working enhance business operations rather than disrupt them.
You successfully did that: combined long-term efficiencies with keeping day-to-day operations running smoothly during the transformation period. What strategies help organizations achieve this balance?
One of the biggest mistakes of a company during transformation is attempting to execute everything simultaneously. When operations face disruption, leadership often reacts by scaling back initiatives, leading to wasted investment and stalled progress. A more effective approach is structuring transformation in phases, ensuring stability while driving change. We addressed this challenge at a top Russian retail bank by shifting toward a platform operating model in a controlled, phased manner. While many industry leaders were opting for abrupt transformations to demonstrate immediate cost reductions to the market, I took a more strategic approach. Instead of forcing a disruptive shift, I designed a transformation roadmap that introduced foundational changes incrementally, minimizing risk and operational disruptions. As these foundational changes took hold, we focused on capability building and training employees on new systems while maintaining legacy processes to ensure continuity. Only once all risk factors were addressed did we transition to a fully optimized platform-based model, allowing for a seamless and effective transformation.
This highlights a broader issue — many companies still view transformation primarily as a means to cut costs, missing the opportunity to create value through more efficient operating models. True transformation isn’t just about reducing expenses – it’s about strategically reallocating resources to enhance efficiency, innovation, and competitive advantage.
Your work with a major U.S. investment bank showed how optimizing market data procurement saved 5–10% annually while ensuring long-term resilience. How exactly can banks reduce costs while still driving innovation?
The most successful companies understand that cost optimization must be embedded into the operating model rather than treated as a one-time budget-cutting exercise. Banks that focus purely on expense reduction often undermine their long-term competitiveness.
For instance, I led a team that helped a major U.S. investment bank optimize market data procurement costs in one project, achieving millions in annual savings. However, the real success came from integrating cost efficiencies into the bank’s operating model—ensuring that savings weren’t just a short-term gain but part of a sustainable framework for resource allocation. We enabled long-term financial resilience by redesigning procurement processes, automating cost tracking, and embedding efficiency levers within decision-making workflows. This approach was truly unique because it didn’t just focus on reducing expenses—it introduced a dynamic, AI-enhanced cost optimization model that continuously adapted to market changes, allowing the bank to proactively manage vendor spending and unlock new efficiency opportunities in real-time. This forward-thinking strategy transformed cost management from a reactive budgeting exercise into a strategic lever for financial and operational agility.
Another example is in AI-driven SME banking analytics, where I led operating model refinements that introduced a truly novel approach—such as shifting customer engagement from traditional, reactive service models to highly scalable, predictive AI-driven systems. Rather than simply automating existing workflows, my team embedded AI into the core of relationship management, enabling the bank to anticipate customer needs in real time.
This is why AI and automation must be core to operations, not isolated tools, to streamline processes and drive innovation.
AI is reshaping the financial industry, yet many banks struggle to integrate it effectively into their core operations. Based on your experience in AI-driven banking transformations, what factors enable successful AI adoption within an operating model?
AI delivers the most value when integrated into the operating model rather than treated as an experimental tool for isolated processes. Many banks run AI pilots but fail to embed them into core business workflows, which leads to underwhelming results.
At a top Russian retail bank, I led one of the most impactful AI-driven marketing transformations, embedding Next Best Product (recommendations for relevant products) and Next Best Offer (tailored promotional offers) models into the customer engagement platform. In this transformation, rather than simply automating outreach, we leveraged machine learning to analyze customer transactions and behavioral patterns in real-time, allowing relationship managers to anticipate customer needs proactively with hyper-personalized product recommendations. This resulted in higher conversion rates (by 25-30% for select campaigns), improved customer retention, and optimized marketing spending. Ultimately, we were pioneers in this transformation – our AI-driven engagement model was first adopted in other business units within the bank before being replicated across the industry by competing financial institutions.
The key to success was threefold: integrating AI with human expertise so employees could use AI-driven insights to enhance customer interactions; starting with targeted applications where machine learning added clear value rather than overhauling everything at once; and securing strong executive support to ensure AI adoption was treated as a business priority, not just an experiment.
AI is now a competitive advantage in any sphere, including financial services.
As an experienced strategic consultant, what advice would you give to the 30% of financial institutions that have yet to restructure their operating models?
First, redefine decision-making structures to increase agility. Many banks still operate with rigid hierarchies that slow down execution. In my work with leading financial institutions, I have helped drive these changes by introducing cross-functional teams and empowering mid-level managers to drive transformation, ensuring that change isn’t bottlenecked at the top.
Second, prioritize data-driven scalability from the outset. Instead of retrofitting analytics and automation onto old workflows, organizations should design their operating models around real-time data insights, allowing for faster risk assessment, cost optimization, and improved customer personalization.
Third, embed adaptability as a core principle rather than treating transformation as a one-off project. Markets, regulations, and technologies are evolving rapidly, so institutions must build a framework for continuous iteration rather than just implement a static new model. This means creating internal mechanisms for ongoing assessment and recalibration, ensuring the operating model remains relevant as the industry shifts.
The bottom line? Successful financial institutions don’t just adapt once—they build models that adapt a constant capability.
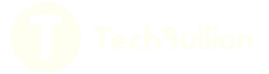