Author: Timur Egorov
By 2026, the global e-commerce market will reach $8.1 trillion. That’s a 56% increase from 2018. Along with profits, competition is on the rise. Predictive analytics, recommendation systems, dynamic pricing – these are just some of the competitive advantages that innovative e-commerce sites are gaining through the introduction of AI-based technologies.
Whether it’s neural networks predicting customer behavior based on their actions on the e-commerce site, on the Internet in general. Or about product recommendations that accurately guess the buyer’s needs.
The growth of conversions and purchases on marketplaces is related to the personalization of the buying process, which is provided by various neural network tools. Let’s review the trends in the use of AI in e-commerce that make the shopping experience increasingly personalized.
Predictive analytics tools, or how to predict the future
By collecting real-time information about user actions, neural networks can predict trends, changes in demand. This is the main area of application of AI-based predicative IT systems today. With the help of neural networks, machine learning (ML) and data analysis, it is possible to collect real-time information about user actions on the marketplace platform, as well as on the Internet in general. Based on this information, AI can make predictions about developments related to an individual customer, as well as all customers or groups of customers (cohort analysis).
When the predictive neural network is trained correctly and the prediction is correct, it is possible to fine-tune supply logistics and warehouse processes, more accurately calculate the parameters of discounts and promotions, reduce costs and increase profits. All the long-lasting advantages are hard to list. To give a full picture of the power of the tool a marketplace gets at its disposal, just a few more examples will suffice. Predictive AI tool analytics:
- Correctly determine the time when a marketing mailing will be most effective;
- assess the solvency of a particular user;
- determine his/her interests.
If we talk about trends for an individual customer, such KPI as the likelihood of repeat purchase is increasing as offers become more personalized. User satisfaction with the buying process is also increasing. Next to AI developments that make simple extrapolations of a user’s studied history: for example, by the brands he buys or by his demographic characteristics: gender, age, location, there are sophisticated neural networks capable of predicting the future: whether a particular customer will make a repeat order, whether or not it will be possible to attract a certain number of new customers from the same group of users (cohort).
Further development of AI in e-commerce is associated with such systems that look even further into the future and make predictions about specific events important for trade. Many large e-commerce marketplaces decide to develop their own AI-powered predicative modules, but smaller marketplaces don’t always have the opportunity to do something similar. Fortunately, many IT solutions in this area have entered the market as B2B products: Loginom, SAS Enterprise Miner, In-DAP. In some of them it is possible to get and use the results of predicate analysis even without code.
Recommendation systems, or how to get a customer to buy again
Perhaps the best way to demonstrate the work and effectiveness of AI-based recommendation systems in e-commerce is through the cases of companies that are already actively using this tool in their practice. A large online store Garderobo.ai, which sells clothing, footwear and accessories, increased the conversion rate (effectiveness) of product cards by 2.5 times, and the number of complaints about already purchased goods – by 40%, having implemented ad blocks on the site: “Recommended”, “Similar”, “Popular”, and Total Look.
AI selected ads for the first of them on the basis of analyzing the psychological characteristics of a particular current or potential buyer based on his actions on the site. In particular, according to the developers, for the development of those elements of the system that assessed the psychological state, the methodology of transactional analysis, common in psychology, was used. The content for the second block “artificial intelligence” formed by comparing the types of clothing purchased by users with other goods that were available at the e-commerce site. The comparison was based on 200 parameters. For example, one of them was size. In the third block, the neural network simply offered the most popular products from the categories that the individual user was interested in.
Finally, the Total Look block was implemented as a service for forming an individual style. The customer receives a customized style and links to new items of clothing that complement it. The “smart” stylist is controlled by a neural network that draws relevant conclusions based on the user’s past purchases.
Personalized offers allow to engage the customer in the shopping process many times stronger than it turns out for e-commerce platforms, which still do not apply AI-based tools. There are several basic mechanics that form the basis of recommendations to users, you can:
- Analyze the content of what a user views on a site or app. Analytics on some e-commerce platforms are more subtle than commonly thought. Not only the category of goods in the catalog, but also color, shape, and other more or less intuitive aspects can be taken into account;
- offer those products that the audience with similar interests and preferences is looking for. For example, if customers who previously bought bricks were often interested in lawnmowers and the neural network knows about it, it will offer a lawnmower to a new brick buyer;
- offer items related to something the user has already bought. This is called cross-selling. The logic is based on the fact that if a customer needs shoes, they will also need products to clean or dry them;
- offer a newer or more expensive product instead of the one the user has discovered and is about to purchase. This is called upselling.
The effectiveness of such mechanics is well demonstrated by the experience of large e-commerce platforms such as Netflix. The streaming service recently stated that 80% of views come from recommendations generated by “artificial intelligence”. In the field of personalized recommendation systems, as in predicative systems there are B2B frameworks, libraries and analytics services. Some Amazon Personalize or Google Recommendations AI platforms combine both cloud- and UI-embedded functionality and analytics platforms for marketers and e-commerce site management.
AI on the first line of customer support, or “you haven’t answered me”
An interesting example of AI-powered personalization is online cosmetics and apparel retailer Estee Lauder’s use of Google’s “artificial intelligence,” as part of collaboration with Google Cloud. By embedding PaLM2, a generative neural network that responds to the user’s statements and texts, AI PaLM2 embedded in shoppers’ paths through the site, unprecedented personalization was achieved. The Estee Lauder interface, thanks to this cooperation, responds with unprecedented precision to trends and the smallest actions of the user, and this happens in real time, as the visitor selects products for himself. Neural network processing of what the customer writes has a huge future and the potential is not limited to chats in which the customer participates. But the ability to hand over communication to AI, even partially, also plays a huge role.
According to Jivo, one of the major players in the chatbot market, if customer support on a website manages to respond in the first 10 seconds, the dialog with the customer will continue in 70% of cases. Stalling for a minute already causes this probability to drop to 62%. Buying goods and services on a marketplace raises many questions for customers and the quality of customer support for e-commerce marketplaces directly determines audience loyalty and propensity to make further purchases. Neural networks trained on Natural Language Patterns (NLP) can solve the problem of bloated customer support staffs.
Today, a neural network can capture the mood of a writer (e.g., the Watson Tone Analyzer framework) and relay the conversation to a human expert if the person is angry or worried. It decomposes incoming messages into essential and non-essential components, extracting meaning. Then, with the help of speech synthesis technologies, it responds, similar to the famous Chat GPT. The use of such technologies reduces the cost of a large customer service department and solves some problems that even the largest divisions of companies of this kind do not solve. In particular, “neurons” can respond almost instantly, which support operators usually cannot handle, plus the robot works 24/7.
Success in utilizing AI will determine the winner in competitive e-commerce marketplaces?
The growth of e-commerce has led to a very competitive atmosphere in this market. According to calculations of experts, on average, consumers start but do not finish the checkout process in the product card 70% of the time. Consumers are fastidiously moving between interfaces in search of the best offer. They will stay with the platform that offers them the most personalized interaction. On the current track, the success of some e-commerce platforms and the failure of others is determined by the successful integration of AI into their business processes and user interfaces.
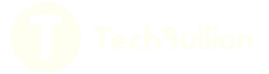