In an era where supply chain disruptions can significantly impact business performance, AI-driven solutions are redefining procurement strategies. Suman Etikala, an expert in intelligent automation, explores the transformative power of Machine Learning Operations (MLOps) in procurement replenishment. This article delves into the innovations that are enhancing efficiency, accuracy, and resilience in supply chain management.
Real-Time Inventory Monitoring: A Game Changer
Traditional inventory management systems often struggle with delays and inaccuracies, leading to either overstocking or stockouts. By integrating real-time monitoring through IoT sensors and RFID technology, organizations can track inventory levels with precision. Studies have shown that IoT-enabled inventory management reduces labor costs by up to 30% and improves stock accuracy by 20-30%. With continuous monitoring, companies can react to inventory discrepancies immediately, reducing stockout scenarios by as much as 90%.
Predictive Analytics: Smarter Demand Forecasting
Demand forecasting has evolved from static historical data analysis to dynamic, multi-source predictive modeling. Advanced AI systems integrate market indicators, competitive intelligence, and historical sales data to improve forecasting accuracy. Research indicates that organizations using multi-source demand forecasting achieve up to 15% lower error rates compared to traditional methods. By incorporating dynamic feature engineering, businesses can reduce forecasting errors by up to 30%, ensuring inventory levels align with real demand.
MLOps: Automating Model Retraining for Accuracy
MLOps introduces a robust automation framework that ensures predictive models remain relevant over time. Automated retraining mechanisms analyze key performance indicators and trigger updates when performance degrades. Studies suggest that organizations employing automated retraining improve model longevity by 30-50% and detect performance issues 40% faster than with conventional periodic reviews. This continuous learning approach minimizes disruptions while optimizing procurement strategies.
Supplier Performance Metrics: Data-Driven Procurement Decisions
Choosing the right suppliers is critical to maintaining an efficient supply chain. AI-driven supplier evaluation systems analyze quality, delivery reliability, and cost efficiency. Research has shown that organizations using structured supplier performance measurements experience a 26% improvement in quality, a 28% increase in on-time delivery rates, and a 24% reduction in procurement costs. By leveraging comprehensive vendor scorecards, procurement teams can make informed, risk-adjusted decisions that drive long-term efficiency.
Dynamic Safety Stock Optimization: Balancing Cost and Availability
Maintaining the right level of safety stock is essential to avoid disruptions while minimizing excess inventory costs. AI-powered safety stock optimization continuously recalibrates inventory buffers based on real-time demand patterns, supply chain variability, and service level targets. Organizations implementing dynamic safety stock systems report reductions in carrying costs by up to 20%, with stockout incidents decreasing by 25-30%. These adaptive models provide a balance between cost savings and uninterrupted supply.
Automated Cost-Benefit Analysis: Smarter Procurement Strategies
Procurement is no longer just about finding the lowest price. AI-driven cost-benefit analysis evaluates multiple factors, including order frequency, transportation efficiency, and stockout risk penalties. Studies indicate that organizations employing automated economic modeling reduce total supply chain costs by 8-12%. Advanced procurement engines dynamically calculate order quantities to optimize both cost and service levels, leading to a 5-8% decrease in procurement expenses.
Risk-Adjusted Recommendation Engine: Enhancing Supply Chain Resilience
Procurement strategies must adapt to external risks, such as geopolitical instability, weather disruptions, and market fluctuations. AI-driven recommendation engines integrate real-time risk assessments into procurement planning. Research has shown that organizations leveraging risk-based procurement strategies reduce supply chain disruptions by up to 45% and improve crisis response times by 36-50%. By incorporating predictive analytics, companies can preemptively mitigate risks and maintain operational stability.
The Future of AI in Procurement
As supply chains become more complex, the role of AI in procurement will only grow. MLOps-driven automation ensures continuous improvements, making supply chains more resilient and cost-efficient. Organizations that embrace these intelligent systems gain a strategic advantage through enhanced decision-making, optimized inventory levels, and improved supplier relationships.
In conclusion, Suman Etikala’s research underscores that the integration of AI and MLOps in procurement is not just a trend but a necessity for businesses aiming to thrive in an unpredictable global market. By leveraging these technologies, organizations can enhance decision-making, optimize supply chain operations, and drive greater efficiency. As procurement continues to evolve, AI-driven automation and data-driven insights will be crucial in ensuring resilience, agility, and long-term success.
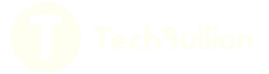