In the rapidly evolving landscape of technology, Artificial Intelligence (AI) has emerged as a catalyst for transformative change in organizational productivity. This article delves into the innovative metrics and frameworks proposed by Vinay Puri to measure AI’s impact on productivity comprehensively. Puri, a renowned expert from Florida International University, provides insights and practical strategies to quantify the multifaceted effects of AI initiatives within organizations.
The Potential and Challenges of AI Integration
AI integration into organizational workflows promises significant productivity gains, ranging from automating routine tasks to providing advanced analytics. However, realizing these benefits requires careful measurement and evaluation. A recent study by Accenture indicates that AI could boost labor productivity by up to 40% by 2035, underscoring its vast potential. Yet, many organizations struggle to quantify the specific impacts of their AI investments. According to a NewVantage Partners survey, only 31% of firms consider themselves data-driven, highlighting a significant gap between AI adoption and measurable outcomes.
The complexities of measuring AI’s impact on productivity are multifaceted. AI often affects multiple aspects of an organization simultaneously, making it difficult to isolate its specific contributions. For instance, an AI-powered customer service chatbot may reduce response times, improve customer satisfaction, and free up human agents for more complex tasks. Quantifying these interrelated effects requires a holistic approach.
Innovative Metrics for Measuring AI Impact
Vinay Puri introduces innovative metrics to comprehensively assess AI’s impact on organizational productivity across several dimensions. The Task Complexity Index (TCI) evaluates how AI enables employees to shift from low to high-complexity tasks, exemplified by a financial services company increasing its TCI from 4.2 to 7.1 with AI implementation. The Quality Consistency Score (QCS) measures reductions in product variability, as seen when an automotive parts manufacturer improved its QCS from 82% to 95% through AI-driven quality control.
The AI Value Creation Index (AVCI) combines cost savings, revenue growth, and intangible benefits; a retail company used it to prioritize a customer recommendation system with an AVCI of 85. The AI Innovation Diffusion Rate (AIDR) tracks the spread of AI innovations, illustrated by a multinational consumer goods company’s market trend prediction tool adoption rate increasing from 30% to 75% in a year.
Best Practices for AI Impact Measurement
Implementing robust measurement methodologies and addressing common challenges are crucial for effectively assessing AI’s impact on productivity. The Baseline Stability Index (BSI) assesses the reliability of pre-AI metrics to ensure consistent and representative baseline data for accurate comparisons. For instance, a telecommunications company extended its baseline measurement period for customer churn rate to achieve a stable comparison point.
Dynamic A/B Testing continuously optimizes the allocation of users to test groups based on real-time performance data, enabling more efficient and responsive testing of AI systems. An online education platform used this method to validate its AI-powered course recommendation system’s effectiveness. AI Impact Network Analysis maps the ripple effects of AI implementations across departments and processes, offering a comprehensive view of AI’s organizational impact.
Combining multiple performance indicators into a single metric, AI System Health Scores allow for quick identification of AI systems needing attention. A financial institution used this approach for monitoring various AI applications, including fraud detection and chatbots. Additionally, AI Sentiment Analysis Framework processes and quantifies qualitative feedback from stakeholders, turning subjective insights into actionable metrics. A healthcare provider improved patient sentiment scores by implementing a patient education program about AI in healthcare.
The Long-Term and Ethical Considerations of AI
The dynamic nature of AI necessitates a flexible and adaptive approach to productivity measurement. As new AI capabilities emerge, organizations must continuously update their measurement frameworks to capture potential impacts. Additionally, ethical considerations and societal impact should be integral to AI impact measurement. Metrics should incentivize responsible AI use and consider potential negative externalities.
In conclusion, successfully measuring AI’s productivity impact requires a multifaceted approach combining rigorous statistical methods, adaptive measurement frameworks, and ethical considerations. By implementing the proposed metrics and best practices, organizations can better understand the value of their AI investments, make informed decisions about future AI initiatives, and navigate the evolving landscape of AI-driven productivity enhancement.
By adopting this comprehensive and nuanced approach to defining productivity in the context of AI, organizations can more accurately assess the true impact of their AI initiatives and make informed decisions about future investments and strategies.
Read More From Techbullion And Businesnewswire.com
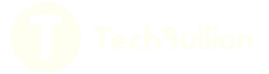