In recent years, the world of network management has witnessed a dramatic transformation, driven by the integration of artificial intelligence (AI). This shift marks a significant departure from traditional methods and is reshaping how telecommunications infrastructure is monitored, maintained, and optimized. A leading force in this revolution is Venkatesh Cumbakonam Gokulraju, whose insights have demonstrated how AI can not only enhance efficiency but also automate network operations to a degree previously thought impossible.
The Challenges of Traditional Network Management
As networks grow in complexity, managing them has become increasingly difficult. Enterprises today handle a staggering amount of data and thousands of devices that need to remain connected and functioning seamlessly. The complexity has skyrocketed, leaving traditional methods struggling to keep up. For example, the average network now processes petabytes of data monthly, with teams managing thousands of alerts daily. Conventional approaches have simply not kept pace, with downtime averaging several hours and service disruptions happening before issues are even detected.
AI: The Backbone of Modern Network Management
At the heart of AI-driven network management lies a six-layer architecture designed to address the growing demands of modern telecommunications. This architecture integrates machine learning (ML) and advanced algorithms to automate processes like anomaly detection, resource allocation, and predictive maintenance. By leveraging neural networks and deep learning, AI systems are able to identify patterns, detect issues early, and provide solutions before problems impact the end user.
One of the most significant advantages of this approach is its ability to self-heal. Where traditional systems would require manual intervention, AI-driven networks can autonomously correct issues, making real-time adjustments to maintain service quality. This is a game changer for industries where downtime can result in significant financial losses.
Enhancing Network Efficiency with Predictive Capabilities
Predictive maintenance is another area where AI is making waves. Traditional systems rely on reactive maintenance, only addressing issues once they have already caused damage. In contrast, AI-driven architectures anticipate potential failures before they occur, using data and pattern recognition to predict when and where problems might arise. This foresight allows teams to schedule repairs in advance, dramatically reducing unscheduled downtime and improving overall system reliability.
Moreover, the predictive capabilities extend beyond just maintenance. By analyzing network performance data in real-time, AI systems can optimize resource usage, ensuring that bandwidth, computing power, and storage are allocated where they are needed most. This reduces inefficiencies and lowers operational costs, delivering both technical and financial benefits.
Autonomous Decision-Making and Operational Optimization
Autonomous decision-making is a core feature of AI-powered network management. Using decision intelligence algorithms, these systems execute policies and make operational decisions without human intervention. AI-driven networks enhance operational efficiency and ensure the network remains agile, adapting to changing conditions in real-time.
For example, the integration of Software-Defined Networking (SDN) controllers enables dynamic resource allocation, allowing the network to respond instantly to shifting demands. Whether adjusting for traffic spikes or ensuring optimal data flow, AI-driven orchestration reduces the need for manual intervention, freeing up resources and allowing teams to focus on strategic tasks.
Real-Time Analytics: Unlocking Unprecedented Insights
Real-time analytics is another transformative element of AI-driven network management. With the ability to analyze vast amounts of data instantly, these systems offer operators insight previously unattainable. Real-time monitoring of network health allows for immediate detection of issues, while sophisticated visualization tools provide a clear view of performance metrics.
AI systems also enable operators to forecast network behavior based on historical data and usage patterns, offering a proactive approach to management. These tools can highlight potential bottlenecks, security threats, or capacity issues before they become significant problems, allowing businesses to act swiftly and prevent disruption.
In conclusion, the integration of AI in network management is not merely an upgrade, it’s a complete rethinking of how networks operate. With the ability to self-heal, predict failures, and optimize resources autonomously, AI-driven architectures are transforming the way businesses manage their networks. These advancements are setting new standards for performance, efficiency, and reliability in telecommunications. As Venkatesh Cumbakonam Gokulraju’s work has demonstrated, the future of network management is one where AI not only supports operations but drives them, creating a resilient, efficient, and ever-evolving infrastructure.
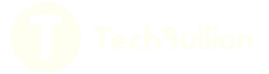