Unraveling the Intersection of Kubernetes, ML, and Cybersecurity: A Conversation with Dinesh Chittibala
In today’s rapidly evolving technological landscape, the convergence of Kubernetes, machine learning (ML), and cybersecurity has become increasingly critical. At the forefront of this intersection stands Dinesh Chittibala, a seasoned leader with a track record of spearheading innovative solutions in this domain. In a recent interview, Dinesh shared his insights and experiences, shedding light on the significant impact of his work in driving forward advancements in technology.
In a recent interview with Dinesh Chittibala, we gained valuable insights into the intersection of Kubernetes, ML, and cybersecurity, and the significant impact of his work in this arena.
“Complexity management is one of the foremost challenges in deploying ML models at scale,” Dinesh remarked. “Kubernetes has emerged as a critical tool for abstracting away some of this complexity, allowing teams to focus on the ML models themselves rather than the intricacies of deployment and scaling.”
If we emphasize more on the importance of automation across the entire ML lifecycle, from data preparation to model training, deployment, and monitoring. “Tools and practices that facilitate automation, such as CI/CD pipelines tailored for ML workflows, are vital for enhancing efficiency and reducing the potential for human error,” he noted.
Moreover, Dinesh provided insights into future trends and practices in the field, highlighting the evolving landscape of AI/ML Ops maturity and the increasing adoption of hybrid and multi-cloud strategies. “As organizations strive for flexibility and resilience, hybrid and multi-cloud deployments of ML models will become more common,” he explained. “My expertise in creating cloud-agnostic Kubernetes solutions positions me well to lead in this space.”
Dinesh’s journey in the realm of Kubernetes, ML, and cybersecurity has been marked by remarkable achievements, showcasing his proficiency and leadership in the field. One notable accomplishment is his pioneering role in developing a cutting-edge Kubernetes-based solution aimed at streamlining the deployment, scaling, and management of ML applications. Through this initiative, Dinesh successfully reduced deployment times and operational complexities, ultimately enhancing the productivity of data scientists and ML engineers.
Furthermore, Dinesh’s expertise extends to optimization and performance enhancements in production environments. By integrating advanced monitoring and autoscaling capabilities into Kubernetes, he achieved a significant improvement in resource efficiency and application responsiveness, thereby bolstering the reliability and performance of ML applications.
Collaboration and leadership are also hallmarks of Dinesh’s approach to driving technological innovation. Leading a cross-functional team comprising DevOps engineers, ML engineers, and data scientists, he fostered collaborative development and deployment practices, ensuring seamless integration of ML models into production pipelines.
Within his workplace, Dinesh’s contributions have resulted in tangible outcomes, ranging from efficiency gains to cost savings. By streamlining ML deployment processes, he engineered a more efficient and scalable approach, reducing operational overhead and boosting productivity. Additionally, his efforts in enhancing system reliability and uptime have minimized downtime, thereby enhancing user satisfaction and trust in the organization’s ML capabilities.
Expert’s involvement in significant projects both within and outside his organization further underscores his expertise and innovative approach. From creating end-to-end ML deployment pipelines to developing multi-cloud ML platforms, his projects have demonstrated his ability to architect and implement complex solutions tailored to the specific challenges of ML deployment.
The impact of his work can be measured in quantifiable terms, reflecting his contributions to efficiency, reliability, and cost savings. Through his interventions, he has achieved notable improvements in deployment times, resource utilization, system availability, and overall model performance.
Looking ahead, Dinesh remains optimistic about the future trends and practices in the field of Kubernetes, ML, and cybersecurity. He emphasizes the importance of automation, collaboration, and continuous learning in driving forward technological advancements and addressing the evolving challenges in this domain.
In conclusion, Dinesh Chittibala’s expertise and contributions in the intersection of Kubernetes, ML, and cybersecurity stand as a testament to his leadership and innovation in the field. As technology continues to evolve, his insights and experiences serve as valuable guiding lights for navigating the complexities of the digital age.
Read More From Techbullion
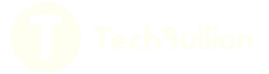