In an era where artificial intelligence (AI) is becoming embedded in our daily lives, the evolution of how machines process and retrieve information is paramount. Sarat Kiran‘s recent article on hybrid retrieval-augmented generation (RAG) systems introduces an innovative approach that enhances the performance of large language models (LLMs). By seamlessly integrating embedding vector databases with traditional retrieval methods, this research aims to overcome critical limitations of existing AI systems, offering promising solutions for more accurate and efficient AI-powered applications.
Bridging the Gap in AI Knowledge
Large language models (LLMs) have made significant strides in natural language processing, exhibiting impressive capabilities in text generation and understanding. However, these models often struggle with issues like knowledge cutoff, hallucinations, and the inability to access up-to-date, domain-specific information. These challenges limit the effectiveness of LLMs in applications like healthcare, legal services, and support.
The concept of Retrieval-Augmented Generation (RAG) was introduced as a solution to these shortcomings. RAG systems enhance LLMs by supplementing their capabilities with external knowledge retrieval mechanisms, allowing the model to pull relevant information from up-to-date databases before generating responses. This integration helps reduce inaccuracies, such as hallucinations, by grounding outputs in verified data.
The Hybrid Advantage: Combining the Best of Both Worlds
Traditional retrieval methods often rely on keyword matching, while modern embedding-based retrieval methods focus on semantic similarity. Both approaches have their advantages and limitations, but combining them can lead to a more powerful system. Kiran’s research introduces a hybrid retrieval strategy, combining dense vector search with traditional keyword-based techniques. This approach addresses the weaknesses of each method—dense retrieval is excellent for capturing semantic meaning but can miss exact term matches, while keyword-based methods excel in matching but overlook nuances.
By integrating these strategies, the hybrid system achieves significant improvements in recall and relevance. Hybrid methods outperform both standalone vector-based retrieval and keyword-only approaches, improving recall by 15-35% and precision by up to 30%. This is crucial in environments that demand both semantic understanding and precise, fact-based responses.
A Modular Architecture for Flexibility and Efficiency
The proposed RAG system architecture is modular, with distinct components for the retriever and generator functions. This separation allows for integration with various vector database technologies, ensuring the system can be tailored to meet specific requirements. The retriever component identifies relevant documents from a vast corpus using dense passage retrieval, while the generator synthesizes responses by conditioning on the retrieved information.
This modular design also ensures scalability and efficiency. By leveraging feedback loops and re-ranking strategies, the system can refine its retrieval and generation processes to enhance response quality. The flexibility of this architecture means it can be easily adapted for industries, from healthcare to finance, where domain-specific knowledge is essential for accurate AI-driven outcomes.
Real-World Applications and Future Prospects
One of the most promising aspects of this research is its potential for real-world applications. The hybrid RAG system demonstrates its utility in specialized domains, including healthcare, legal services, and financial sectors, where accuracy and up-to-date information are critical. In healthcare, domain-specific RAG systems have shown error reduction rates of up to 85%, ensuring more reliable responses for clinical queries. In the legal field, hybrid retrieval methods combine semantic search with exact citation matching, navigating legal terminology with greater precision.
The success of this system underscores the importance of tailored solutions in AI. Rather than relying on generic approaches, domain-adapted RAG systems achieve superior performance by incorporating knowledge specific to each field.
In conclusion, as AI continues to evolve, the integration of hybrid retrieval-augmented generation systems is poised to play a crucial role in bridging the gap between machine learning models and real-world applications. By enhancing LLMs with external knowledge sources, these systems will provide more accurate, contextually relevant, and reliable results across a range of industries. Sarat Kiran’s research offers an exciting glimpse into the future of AI, highlighting how hybrid retrieval strategies and embedding vector databases can revolutionize the capabilities of language models. These advancements will be pivotal in ensuring that systems can provide timely, accurate, and domain-specific insights, meeting the growing demands of industries that rely on precise and context-aware information.
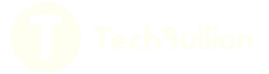