In this modern era, the integration of real-time genomic analytics into clinical practice is transforming healthcare delivery by enabling faster diagnostics and personalized treatment. Viswaketan Reddy Prodduturi, a researcher in genomic data systems, has developed a framework for high-throughput genomic data processing to enhance clinical decision-making and precision medicine.
The Evolution of Genomic Data Processing
The rise of next-generation sequencing technologies has dramatically increased the volume of genomic data generated in clinical settings. Traditional batch processing systems, which once required days for variant analysis, have been replaced by real-time genomic analytics capable of delivering results in hours. Modern parallel computing frameworks and stream processing technologies now enable healthcare providers to integrate genomic data into clinical workflows seamlessly, improving the speed and accuracy of diagnostic decisions.
Overcoming Challenges in Real-Time Analytics
Managing Data Volume and Speed
Handling vast amounts of genomic data remains a key challenge. Current high-throughput sequencing platforms generate terabytes of data per run, necessitating sophisticated computational frameworks. Distributed storage systems combined with real-time stream processing allow for rapid data analysis while maintaining operational efficiency. These solutions ensure that genomic data can be processed within clinical timelines without compromising accuracy.
Data Integration for Clinical Insights
Integrating genomic data with electronic health records (EHRs) and other clinical information is critical for delivering actionable insights. Multi-modal data harmonization techniques ensure consistency across diverse data sources, enabling meaningful correlations between genetic variants and patient phenotypes. Automated validation protocols and error detection systems improve data reliability, ensuring accurate clinical interpretations while minimizing delays.
Privacy and Security
The sensitive nature of genomic data requires robust privacy-preserving frameworks. Advanced encryption techniques, such as homomorphic encryption, allow computations to be performed on encrypted data without compromising security. Role-based access controls and compliance with regulations like HIPAA and GDPR further ensure that patient data remains protected throughout the analysis pipeline.
Transformative Impact on Clinical Applications
Faster Diagnostics and Personalized Medicine
Real-time genomic analytics has revolutionized diagnostics by enabling rapid identification of disease-causing mutations. Variant calling pipelines now achieve diagnostic accuracies of up to 98%, with processing times reduced from 72 hours to under 8 hours. These advancements allow clinicians to tailor treatments to individual genetic profiles, particularly in time-sensitive scenarios like cancer therapy and rare disease management.
Population Health Insights
The implementation of genomic analytics has facilitated large-scale studies of genetic patterns across populations. By analyzing vast datasets in real time, researchers can identify genetic risk factors and disease-associated variants more effectively. These insights have led to the development of targeted screening programs and preventative healthcare strategies, improving public health outcomes on a broader scale.
Technical Infrastructure Driving Real-Time Genomics
Distributed Storage and Processing
Modern genomic analytics relies on distributed storage architectures that balance scalability and performance. Hybrid systems combining cloud and on-premise storage enable institutions to handle computationally intensive tasks while maintaining regulatory compliance. Stream processing frameworks like Apache Kafka facilitate continuous data ingestion and analysis, providing real-time insights to clinicians.
Optimization Through Machine Learning
Machine learning algorithms enhance the efficiency of genomic workflows by predicting workload patterns and optimizing resource allocation. These systems enable dynamic load balancing, ensuring consistent performance during peak data processing periods. As a result, healthcare providers can maintain high levels of service reliability while reducing operational costs.
The Future of Genomic Analytics
Emerging technologies such as edge computing, blockchain, and advanced visualization tools promise to further transform genomic analytics. Edge computing reduces latency, enabling point-of-care genomic analysis in resource-limited settings. Blockchain ensures secure and transparent data sharing, while interactive visualization tools enhance the interpretability of complex genomic datasets. These innovations will expand access to genomic medicine and improve the precision of healthcare delivery.
In conclusion, Viswaketan Reddy Prodduturi’s framework for real-time genomic analytics highlights the potential of integrating advanced computing solutions into clinical practice. By addressing challenges in data volume, integration, and security, these innovations enable faster diagnostics and personalized care. As genomic medicine continues to evolve, real-time analytics will play a central role in advancing precision medicine and improving patient outcomes. These advancements pave the way for a future where genomic data becomes a cornerstone of modern healthcare delivery.
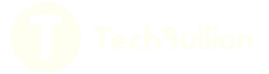