In today’s digital economy, the explosion of data has transformed how financial institutions operate, but it has also opened new avenues for fraud. The sheer volume of transactions and the complexity of data streams make it increasingly challenging to detect fraudulent activities. According to the Federal Trade Commission, consumers reported losing over $8.8 billion to fraud in 2022, a staggering 30% increase from the previous year. Similarly, the Association of Certified Fraud Examiners (ACFE) estimates that organizations lose about 5% of their revenue annually to fraud. These alarming statistics highlight the urgent need for more effective data-driven fraud detection and prevention mechanisms.
The Critical Role of Data Engineering in Fraud Detection
As financial transactions become more digital and instantaneous, the role of data engineering and data science has never been more pivotal. Traditional fraud detection systems struggle to process and analyze the massive influx of data in real-time. The challenge lies not just in detecting fraud but in efficiently ingesting, processing, and analyzing vast amounts of data to identify anomalies quickly.
“Data is the new oil, but it’s only valuable if you can refine it effectively,” says Pankaj Gupta, a leading expert in data engineering, machine learning, and artificial intelligence. “In the context of fraud detection, the ability to handle and process data efficiently is just as important as the algorithms used to detect anomalies.”
An Innovative Approach to Data Engineering for Risk Management
Recognizing these challenges, Gupta spearheaded the development of an advanced data ingestion and fraud detection platform for a major U.S. bank. “Our primary goal was to overhaul the data infrastructure to support real-time analytics,” Gupta explains. “We needed a system capable of ingesting millions of transactions per second and processing them with minimal latency.”
The solution? A robust, scalable data engineering framework that integrates advanced machine learning algorithms for real-time anomaly detection.
Transformative Results
Since its implementation, the platform has delivered impressive results that highlight its effectiveness:
- Enhanced Data Processing Capabilities: The new system can ingest and process data streams in real-time, reducing latency from several hours to mere seconds. “Speed is of the essence in fraud prevention,” Gupta emphasizes. “Our data engineering efforts ensure that data is available for analysis almost instantaneously.”
- Reduction in False Positives: The improved data quality and real-time processing led to a 20% decrease in false positives. “By having cleaner, more timely data, our machine learning models can make more accurate predictions,” says Gupta.
- Scalability and Efficiency: The platform was designed to handle millions of daily transactions without performance loss. “As transaction volumes grow, our system scales effortlessly,” Gupta notes. “This is a testament to the robust data engineering underpinning the platform.”
- Dynamic Risk Scoring: The integration of adaptive machine learning models improved risk assessments, leading to a 25% reduction in payment delinquencies. “Efficient data pipelines enable our models to learn and evolve,” explains Gupta. “This adaptability is key to staying ahead of fraudsters who constantly change their tactics.”
- Significant Cost Savings: The institution realized estimated annual savings of $15 million through reduced fraud losses and operational efficiencies. “Optimizing data workflows not only prevents losses but also reduces operational costs,” Gupta remarks. “It’s about doing more with less.”
A Real-World Case Study: Data Engineering Challenges in Fraud Detection
To understand the broader impact of advanced data engineering in fraud detection, consider the issue of Real-Time Payment (RTP) fraud. According to a report by McKinsey & Company, the adoption of RTP systems has increased significantly, with transaction volumes expected to grow by 23% annually through 2025. However, this rapid growth presents data processing challenges that traditional systems struggle to meet.
One major financial institution faced difficulties in handling the data throughput required for effective fraud detection in RTP. By overhauling their data engineering infrastructure—implementing scalable data pipelines and real-time processing frameworks—they achieved a 40% reduction in fraud losses. This case underscores the critical role that efficient data engineering plays in modern fraud prevention.
Behind the Development Process
Creating such a sophisticated system was no small feat. Gupta and his team faced the daunting task of integrating cutting-edge data engineering solutions into an established banking infrastructure without disrupting services. “One of our main challenges was ensuring seamless integration,” Gupta recalls. “We had to build data pipelines robust enough to handle real-time data streams yet flexible enough to adapt to the bank’s existing processes.”
Collaboration was key. The team worked closely with various departments to understand their data needs and pain points. “We weren’t just introducing new technology; we were fostering a cultural shift towards embracing data-driven solutions,” says Gupta. They also prioritized compliance with regulatory standards like the General Data Protection Regulation (GDPR) and Payment Card Industry Data Security Standard (PCI DSS) to ensure data security and privacy.
Changing the Landscape of Fraud Detection Through Data Engineering
The success of Gupta’s platform signifies more than just technological advancement; it represents a shift in how financial institutions approach data management and security. By prioritizing efficient data engineering and real-time processing, banks can not only detect but also predict and prevent fraudulent activities more effectively.
“Efficient data engineering is the backbone of any advanced analytics platform,” Gupta notes. “Our goal is to empower machine learning models with high-quality, real-time data so they can anticipate and counteract fraudulent activities.”
Looking Ahead
The implications of this development extend beyond a single institution. It sets a precedent for the industry, highlighting the necessity of embracing advanced data engineering techniques to combat fraud. Gupta believes that ongoing investment in data infrastructure is crucial. “This is just the beginning,” he says. “As data volumes grow, our systems need to become even more efficient and scalable.”
Moreover, the platform’s success underscores the importance of customer trust and satisfaction. “At the end of the day, it’s about protecting our customers and providing them with peace of mind,” Gupta emphasizes. “When they know their transactions are secure, it strengthens their relationship with the bank.”
Conclusion
The advent of this advanced data engineering and fraud detection platform marks a significant milestone in financial security. By addressing longstanding challenges with innovative data solutions, Pankaj Gupta and his team have not only enhanced the protective measures of a major U.S. bank but have also paved the way for industry-wide transformation.
As financial transactions continue to surge in both volume and complexity, the need for robust, intelligent data engineering systems becomes ever more critical. Gupta’s work serves as a testament to what can be achieved when expertise in data engineering, innovation, and a commitment to excellence converge.
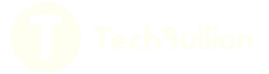