In the fast-paced world of online transactions, fraud prevention is a critical challenge for businesses. As fraud tactics evolve, traditional security systems struggle to keep up, leading to financial losses and poor customer experiences. To combat these challenges, Surendra Lakkaraju has introduced a groundbreaking AI-powered risk assessment framework. His research explores the integration of reinforcement learning, Bayesian networks, and real-time data processing to create a more adaptive and effective fraud detection system.
Dynamic Risk Scoring: A Shift from Static Models
Traditional fraud detection relies on rule-based systems, which can be rigid and ineffective against emerging threats. The new AI-powered framework replaces static risk-scoring methods with dynamic learning models that continuously evolve. By analyzing vast datasets, the system can detect fraud patterns in real time, allowing for proactive security measures rather than reactive responses. The technology leverages advanced anomaly detection algorithms to identify suspicious activities that might evade conventional systems, adapting to fraudsters’ evolving techniques while minimizing false positives and operational friction.
Machine Learning at the Core
The implementation of machine learning in fraud detection significantly enhances security by analyzing over 3,700 data points per transaction. The system leverages reinforcement learning models that continuously update risk parameters based on new fraud patterns. This adaptive approach leads to an impressive reduction in false positives, minimizing unnecessary transaction declines and improving customer trust.
The Role of Bayesian Networks in Fraud Detection
Bayesian networks play a vital role in establishing probabilistic relationships between transaction variables. These networks enable real-time fraud assessments by dynamically adjusting detection thresholds based on evolving risk factors. This approach improves accuracy and reduces false alarms, ensuring that genuine transactions are not mistakenly flagged as fraudulent.
Real-Time Processing for Enhanced Security
One of the major innovations in this framework is the incorporation of real-time transaction monitoring. The system processes vast amounts of transactional data at an unprecedented speed, ensuring that potential threats are identified and mitigated within milliseconds. This real-time capability is essential for large-scale e-commerce platforms handling millions of transactions daily.
Reinforcement Learning for Adaptive Security
Unlike traditional models that require manual updates, reinforcement learning enables the system to automatically refine its detection techniques. By continuously learning from new fraud patterns, the AI can dynamically adjust its response mechanisms. This leads to a 286% improvement in early fraud detection, making it highly effective against sophisticated cyber threats.
Advanced Feature Engineering for Precision
The system employs advanced feature engineering techniques, analyzing transaction behavior across multiple dimensions. By leveraging historical data, network patterns, and behavioral analytics, the AI can distinguish between genuine and fraudulent transactions with remarkable accuracy. With a fraud detection success rate of 99.4%, this approach sets a new benchmark in security technology.
Optimized Decision-Making with CODAS
The integration of CODAS (Combinative Distance-based Assessment) methodology further refines the fraud detection process. This multi-criteria decision-making framework enhances the system’s ability to evaluate transactions based on multiple risk factors. The result is a substantial reduction in false positives and improved accuracy in identifying fraudulent activities.
Addressing Challenges in Implementation
The transition to AI-driven fraud detection is not without its challenges. One key issue is data imbalance, where fraudulent transactions constitute a small fraction of total transactions. To overcome this, the system uses advanced synthetic sampling techniques, ensuring that machine learning models are trained on balanced datasets. Additionally, real-time processing demands significant computational power, which is addressed through distributed computing and optimized data structures.
The Future of AI in E-Commerce Security
As cybercriminals develop increasingly sophisticated fraud techniques, the need for advanced security solutions becomes more pressing. AI-driven fraud detection represents the future of e-commerce security, offering unparalleled accuracy and efficiency. By leveraging continuous learning mechanisms and real-time monitoring, businesses can stay ahead of cyber threats while ensuring a seamless shopping experience for customers.
In conclusion, Surendra Lakkaraju’s research presents a transformative approach to fraud detection in e-commerce. By integrating reinforcement learning, Bayesian networks, and real-time analytics, this AI-powered framework significantly enhances security, reduces false positives, and improves customer satisfaction. As digital transactions continue to grow, such innovations will play a crucial role in ensuring the safety and integrity of online commerce.
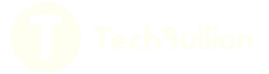