The world of data processing is undergoing a seismic transformation, shifting from batch-oriented workflows to highly dynamic real-time AI systems. In his latest work, Himanshu Adhwaryu spotlights how artificial intelligence and advanced stream processing pipelines reshape modern enterprises by delivering instant insights and automated decision-making. His research illustrates innovative breakthroughs in AI-driven real-time analytics that raise the bar for efficiency, accuracy, and responsiveness.
From Batch Operations to Real-Time Intelligence
For decades, organizations depended on batch processing, where data was gathered and analyzed in scheduled intervals—sometimes taking hours or even days. In contrast, today’s digital landscape demands responses in milliseconds. Real-time AI solutions now allow businesses to process staggering volumes of data in near-zero latency. Advanced platforms handle more than a million events per second, ensuring data-driven intelligence arrives just in time for critical decisions.
Stream Processing Frameworks: The Backbone of Real-Time AI
At the heart of real-time AI are high-throughput stream processing frameworks that continuously ingest and analyze data. Leveraging distributed computing paradigms, these frameworks sustain enormous event rates—exceeding 850,000 per second—while keeping processing delays under 50 milliseconds. This ultralow latency proves indispensable in fields like algorithmic trading, healthcare monitoring, and cybersecurity, where microseconds can differentiate success from failure.
Integrating AI and ML into Streaming Pipelines
A significant leap in real-time analytics is the seamless fusion of machine learning models with streaming pipelines. Intelligent algorithms, embedded directly into data flows, deliver instantaneous recommendations and alerts. Optimized inference engines have pushed prediction latencies to under 10 milliseconds while retaining 98% or higher accuracy. These capabilities are revolutionizing fraud detection—identifying anomalies in financial transactions—and powering predictive maintenance by spotting equipment failures before they escalate.
Overcoming Model Drift and Adaptability
Despite its many advantages, real-time AI must address the ongoing challenge of model drift. Traditional models degrade over time as they encounter evolving data patterns. Continuous learning and online model update mechanisms have emerged to counter this, ensuring AI remains current and self-correcting. Modern solutions achieve update latencies under 100 milliseconds, preventing performance erosion in ever-changing data streams.
Edge Computing: Localized Real-Time Intelligence
Beyond centralized data centers, edge computing has introduced a new milestone for real-time AI. By processing data on or near local devices, edge-based AI drastically reduces dependence on remote cloud resources, slashing latency and bandwidth consumption. Current deployments demonstrate inference times as low as 18 milliseconds, vastly improving IoT responsiveness, autonomous vehicle controls, and on-site industrial analytics and security monitoring.
Federated Learning: Privacy-Preserving AI
With data privacy concerns on the rise, federated learning has gained traction as a vital innovation. Rather than consolidating sensitive information in a single repository, federated learning trains models across multiple decentralized nodes without exposing raw data. This strategy cuts data transfer overhead by up to 82%, preserving model accuracy within 18% of centralized approaches. Sectors like healthcare and finance benefit particularly from this method, given the stringent confidentiality requirements they face.
Quantum Horizons for Real-Time AI
As data volumes grow and model complexity intensifies, quantum computing offers a potential leap forward. Emerging hybrid quantum-classical architectures hint at speedups of 75× for intricate optimization tasks. Early results suggest that quantum-driven AI could slash feature computation from minutes to mere milliseconds, opening unprecedented possibilities in large-scale simulations, high-frequency risk analytics, and cutting-edge scientific research.
Transforming Industry Operations
Real-time AI is already reshaping business fundamentals across numerous sectors. Financial institutions use AI-driven fraud detection systems that process upward of 18,000 transactions per second, slashing false positives by nearly 38%. Healthcare providers lean on AI-based monitoring to identify critical patient conditions up to 4.5 hours earlier than legacy solutions. In insurance, real-time AI streamlines claim workflows, shrinking response times by 65% and bolstering fraud detection rates.
In conclusion,the fusion of AI, edge computing, federated learning, and quantum hardware signifies a historic leap in real-time data processing. Organizations that embrace these breakthroughs gain a decisive edge in operational agility, data security, and user experiences. As Himanshu Adhwaryu demonstrates, real-time AI is not merely the next frontier—it is actively transforming the present, shaping a faster, more intelligent, and more adaptable digital world.
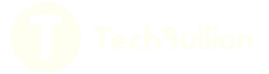