The rapid evolution of cybersecurity necessitates innovative solutions to combat sophisticated threats. In this landscape, the work of Tuhin Banerjee provides a critical exploration of how artificial intelligence, particularly Large Language Models (LLMs), is reshaping Identity and Access Management (IAM). Banerjee’s insights highlight the transformative potential of AI-driven security frameworks that go beyond traditional defenses.
The Shift from Static to Dynamic Security Models
Traditional IAM frameworks rely on static, rule-based access control systems, which often struggle to adapt to the ever-changing cyber threat landscape. AI-driven IAM systems introduce a dynamic approach by continuously analyzing user behavior and contextual data. This shift enables organizations to proactively detect and mitigate threats before they materialize, fundamentally altering cybersecurity strategies.
Advanced Identity Verification Through AI
One of the most significant innovations in AI-enhanced IAM is linguistic-based authentication. LLMs analyze user communication patterns, syntax preferences, and keystroke dynamics to develop probabilistic identity confidence scores. This continuous verification approach makes impersonation significantly more difficult, reinforcing security beyond conventional password-based systems.
Moreover, behavioral biometrics—such as tracking keystroke rhythms and command sequences—adds an additional layer of security. These AI-driven techniques can differentiate between legitimate user activity and potential cyber threats, offering an adaptive authentication process that evolves with user behavior.
Contextual Access Control and the Zero-Trust Paradigm
The zero-trust security model, which mandates continuous verification rather than implicit trust, has gained prominence in modern cybersecurity strategies. AI enhances this approach by integrating contextual awareness into access control decisions. Instead of relying solely on user credentials, AI systems assess multiple factors, including device health, network conditions, and User activity patterns are indeed valuable for determining dynamic access permissions. By implementing AI-driven risk-scoring mechanisms, organizations can move beyond binary access decisions, allowing for nuanced access controls that adjust to real-time threats. This ensures that only the right individuals gain access to sensitive data under the right conditions, significantly reducing the risk of unauthorized breaches.
Additionally, these intelligent systems continuously learn from behavioral analytics, incorporating contextual factors such as time, location, device health and unpredictable behaviors feeds into access decisions.
AI-Powered Policy Management and Autonomous Threat Detection
AI’s ability to autonomously generate and manage security policies represents a groundbreaking development in cybersecurity governance. By analyzing large datasets—such as threat intelligence reports, compliance frameworks, and historical security incidents—LLMs can create adaptive security policies that evolve with emerging threats.
Predictive analytics further enhances security by identifying attack precursors before they materialize. AI-driven systems process vast amounts of unstructured security data to detect subtle correlations that might indicate an impending cyber attack. This anticipatory approach shifts organizational security postures from reactive to proactive, minimizing potential vulnerabilities.
Challenges and Ethical Considerations
Despite these advancements, integrating AI into IAM systems presents several challenges. The computational requirements for AI-driven security models are significant, requiring organizations to balance performance, cost, and scalability. Additionally, AI models are susceptible to adversarial attacks, where malicious actors manipulate AI outputs by introducing deceptive prompt data.
Governance and compliance also pose challenges. AI-driven security decisions must be transparent and explainable to ensure regulatory adherence and build user trust. Organizations must implement oversight mechanisms that ensure fairness and prevent biases in AI decision-making processes.
Future Directions: Quantum-Resistant Security and Self-Healing Systems
Looking ahead, the integration of AI with quantum-resistant cryptographic techniques will be crucial in preparing for future cyber threats. AI can dynamically manage cryptographic challenges based on real-time risk assessments, ensuring resilience against both conventional and quantum-enabled cyber attacks.
Additionally, self-healing security frameworks—where AI systems autonomously detect, respond to, and rectify vulnerabilities—are expected to redefine cybersecurity resilience. These systems will continuously monitor security configurations, making automatic adjustments to counter emerging threats with minimal human intervention.
In conclusion,the fusion of AI and IAM is revolutionizing cybersecurity by introducing adaptive, intelligent security ecosystems. Through continuous learning, contextual decision-making, and predictive threat detection, AI-powered IAM systems provide organizations with unparalleled security capabilities. As Tuhin Banerjee’s research highlights, this transformation is not merely an incremental improvement but a fundamental shift in how digital identities are protected. By embracing AI-driven security innovations, organizations can stay ahead of evolving threats and establish a more secure digital future.
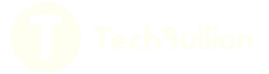