In this modern era, as credit card lines become a crucial aspect of financial institutions, optimizing these assignments has been a significant challenge. Pavan Rupanguntla presents a cutting-edge machine learning framework to refine the credit line assignment process, enhancing precision and operational efficiency. This innovative methodology addresses several inefficiencies in traditional underwriting methods, creating a more dynamic approach to credit risk management. This approach leverages sophisticated data analysis techniques to improve segmentation and adjust credit lines in real-time, ensuring better alignment with customer behaviors and market conditions.
Shifting from Traditional Models to Machine Learning
Traditional credit card line assignments have often relied on static segmentation, using income brackets and credit scores to determine credit limits. However, these conventional methods fail to adapt to rapidly changing consumer behavior and market conditions. With U.S. credit card balances reaching over $1 trillion, there is an increasing need for more sophisticated credit line management strategies. The shift towards machine learning addresses this need by better predicting and managing consumer behaviors, reducing risk while offering greater growth opportunities.
The Role of Machine Learning in Credit Risk Management
Machine learning models substantially improve segmentation accuracy by analyzing a broader range of customer data. The framework he proposes uses clustering algorithms to segment customers based on detailed behavioral data, including payment history, spending patterns, and trends in credit usage. By leveraging advanced data points, such as premium bureau variables, ML algorithms construct sophisticated behavioral profiles that improve decision-making, offering better risk and profitability predictions. This data-driven model enables more accurate credit decisions, significantly improving portfolio outcomes.
Data Engineering: Key to Optimized Credit Line Assignments
The success of the ML-driven credit line assignment framework relies heavily on data engineering. He emphasizes the importance of integrating both historical and real-time data. By incorporating variables like 24-month payment patterns and premium bureau metrics, financial institutions improve their risk models. Advanced trend analysis allows institutions to detect early warning signs of credit deterioration, reducing unexpected losses. This method also enhances credit line flexibility, allowing institutions to adapt more easily to sudden changes in the financial landscape.
Enhancing Customer Segmentation with Clustering Algorithms
At the heart of the proposed framework are clustering algorithms, particularly K-means and hierarchical clustering, that categorize customers based on their credit behavior. K-means clustering outperforms traditional segmentation techniques with an accuracy rate of 85.7%. This clustering methodology enables institutions to identify high-risk customers with greater accuracy, while hierarchical clustering helps spot unique risk behaviors. The flexibility of this approach also allows financial institutions to update and refine customer segments in real-time continuously.
Dynamic and Adaptive Optimization Framework
Unlike traditional methods that offer fixed credit tiers, the machine learning framework provides dynamic line assignments. The system continuously adjusts credit line assignment strategy based on evolving customer behaviors. Customers showing consistent payment patterns may receive better credit lines, while those with fluctuating balances experience conservative line assignments. This system leads to a 27.8% improvement in portfolio performance, offering financial institutions the agility needed to respond to shifting market trends and individual customer needs.
Real-Time Monitoring and Risk Management
A critical innovation of this framework is its ability to perform real-time analysis of credit line assignments. The system ensures credit lines align with the customer’s financial behavior, even as market conditions change. Continuous performance monitoring reduces the time needed to adjust credit line strategy, improving customer satisfaction and operational efficiency. This proactive approach allows institutions to mitigate risk faster and more efficiently than traditional methods, providing a significant competitive advantage in the market.
In conclusion, the shift to machine learning in credit card line assignments represents a significant leap forward in financial risk management. By enhancing customer segmentation, optimizing credit line assignments, and integrating real-time data analysis, this framework offers a more dynamic and precise approach to credit risk management. While challenges remain, the advantages of machine learning-based systems are clear. Pavan Rupanguntla’s work demonstrates how these technologies are reshaping the future of credit card underwriting, ensuring greater adaptability, efficiency, and long-term success in the financial services industry.
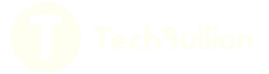