In today’s era of digital transformation, generative artificial intelligence is reshaping how machine learning models are developed beginning with feature engineering. Vineetha Sasikumar, a passionate data science researcher with a strong academic and research background, explores how AI innovations transform feature generation, transformation, and selection in predictive modeling. Her insights clarify this complex but essential aspect of data science, highlighting generative AI’s revolutionary role in modernizing traditional modeling workflows.
The Shift from Manual to Machine-Created Features
Traditional feature engineering heavily relied on manual techniques and domain expertise, limiting both the speed and scope of data modeling. These limitations often led to narrow exploration within high-dimensional datasets. In contrast, generative AI offers a scalable and systematic approach, using algorithms to uncover hidden patterns and produce creative combinations of variables. This transition has enabled more comprehensive modeling by automating non-linear interaction discovery and reducing time-to-market for data products.
Automation That Thinks Ahead
Automated feature generation is no longer just a time-saving tool; it’s an intelligence layer that identifies mathematically significant relationships far beyond human intuition. Modern systems can now evaluate hundreds of thousands of feature permutations in record time. These tools have shown measurable gains in prediction accuracy and efficiency, with interaction features—like combining demographic attributes with behavioral data—proving especially valuable in complex classification tasks.
Adapting Features to Their Domain
Generative AI frameworks now adapt strategies based on data types—like time series, text, or categorical variables—enhancing model effectiveness. Techniques such as rolling averages and frequency decomposition are automatically applied for time series. These domain-sensitive methods surpass traditional manual feature engineering in accuracy and contextual depth. By tailoring transformations to each data type, AI systems capture richer, more relevant information, resulting in models that are more precise and better aligned with the underlying structure of the data.
From Raw Input to Refined Insight
Feature transformation is essential for optimizing algorithm learning, and generative AI has made this process both intelligent and automated. Advanced systems analyze distribution patterns—like skewness and outlier presence—to choose the best normalization or scaling method. Once a challenge due to high dimensionality, categorical data is now efficiently encoded using techniques like binary encoding and contextual embeddings, greatly enhancing performance and reducing computational overhead.
Navigating Complex Data with Ease
Generative AI breaks barriers by extracting actionable insights from unstructured data sources like text and images. Using transformer-based models, these systems can interpret semantics and structure simultaneously, yielding features that are both meaningful and machine-readable. When combined across modalities—like merging text and sensor data—the result is a holistic, multifaceted view that significantly boosts model accuracy while minimizing false positives.
Smart Selection for Smarter Models
As feature sets expand, intelligent selection becomes vital for efficient modeling. Ensemble-based approaches integrate multiple evaluation methods, highlighting features that maximize both predictive power and clarity. Multi-objective optimization frameworks further balance performance with fairness and interpretability, aligning models with ethical and operational standards. These frameworks prioritize not just accuracy but responsible outcomes. Additionally, dynamic feature selection tools enable models to adapt in real time, automatically adjusting to changing data distributions. This adaptability minimizes the need for manual retraining, ensuring sustained relevance and robustness across evolving environments.
Building a Scalable and Responsible Framework
Deploying generative AI in feature engineering demands robust architecture. Microservices and asynchronous pipelines support scalable, high-throughput operations, while centralized feature stores ensure consistency and minimize duplication. Success extends beyond infrastructure—collaborative workflows involving domain experts refine feature relevance through iterative feedback. This synergy enhances model accuracy by blending algorithmic insight with human context. Ethical governance is critical: embedding fairness metrics, ensuring data privacy, and aligning with regulations builds trust and accountability. Together, these elements create a sustainable, intelligent system for real-world AI deployment in feature engineering.
In conclusion, Vineetha Sasikumar’s exploration into the evolving landscape of feature engineering illustrates how generative AI is not just augmenting but transforming data science. The fusion of algorithmic intelligence with human insight is shaping a new paradigm, one where models are faster, fairer, and more adaptive to the real world. As organizations embrace these innovations, they are better equipped to unlock deeper value from their data and stay ahead in a competitive digital economy.
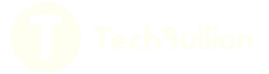