In a pivotal moment for the field of artificial intelligence, Resmi Ramachandranpillai, a trailblazing researcher, has introduced a groundbreaking methodology titled “Fair Latent Deep Generative Models (FLDGMs).” This innovative approach addresses a crucial aspect often overshadowed in the realm of machine learning – fairness in synthetic data generation. Resmi’s work marks a significant step towards achieving ethical AI, revolutionizing how we approach the creation of synthetic data for machine learning models.
The motivation behind Resmi’s research stems from the pressing challenge of integrating fairness objectives into the training of Deep Generative Models (DGMs) without compromising the global convergence characteristics. Traditional fair generative models often face constraints, operating on pixel or attribute levels and demanding complex model architectures, leading to high computational requirements. Resmi’s FLDGMs, however, introduce a pioneering syntax-agnostic, model-agnostic fair latent representation, effectively untangling fairness optimization from the data generation process. This not only enhances stability and optimization performance but also operates in a low-dimensional space, increasing accessibility and computational efficiency.
The core contributions of Resmi’s work are manifold. FLDGMs offer a novel formulation of a fair latent generative framework applicable to both Generative Adversarial Networks (GANs) and Diffusion Models. This breakthrough eliminates the need for delicate weighting factors, ensuring zero regularization of the latent space, guaranteeing high-fidelity reconstructions, and achieving global convergence. The models also exhibit an unprecedented level of generalizability to various data categories, significantly reducing pre-processing and modeling overhead. Extensive experiments across tabular and image domains have validated FLDGMs’ superior performance in terms of fairness and data utility, solidifying their potential impact on the ethical landscape of AI.
Resmi Ramachandranpillai’s work not only represents a technological leap forward but also holds profound implications for society at large. By focusing on fairness in synthetic data generation, Resmi’s research addresses biases present in training data, thereby contributing to the creation of more equitable and unbiased machine learning models. The potential impacts of FLDGMs extend far beyond the realms of machine learning, influencing diverse fields such as healthcare and vision and fostering a new era of responsible AI.
As the ethical implications of AI continue to gain prominence, Resmi Ramachandranpillai’s work at the Department of Computer and Information science at Linkoping University, Sweden, under the guidance of Prof. Fredrik Heintz stands as a beacon, guiding the way towards a future where fairness and ethics are integral components of the AI landscape. The unveiling of Fair Latent Deep Generative Models marks a significant milestone in the ongoing quest for ethical advancements in artificial intelligence.
For more details and access to Resmi Ramachandranpillai’s groundbreaking work, please refer to the citation “Ramachandranpillai, R., Sikder, M.F. and Heintz, F., 2023. Fair Latent Deep Generative Models (FLDGMs) for Syntax-Agnostic and Fair Synthetic Data Generation. In ECAI 2023 (pp. 1938-1945). IOS Press.”
Resmi Ramachandranpillai’s pioneering contributions in the field of synthetic data generation have significantly advanced the development of Fair Latent Deep Generative Models (FLDGMs). These models, as detailed in the referenced paper titled “Fair Latent Deep Generative Models (FLDGMs) for Syntax-Agnostic and Fair Synthetic Data Generation,” co-authored by Ramachandranpillai, M.F. Sikder, and F. Heintz, were presented at the prestigious European Conference on Artificial Intelligence (ECAI) in 2023. The paper delves into the innovative techniques employed by FLDGMs to ensure fairness and syntax-agnosticism in the generation of synthetic data, catering to the evolving needs of diverse applications. For a comprehensive understanding of Ramachandranpillai’s groundbreaking work, readers are encouraged to explore the complete details and insights provided in the cited publication available through IOS Press.
Read More From Techbullion
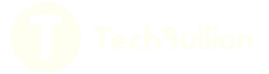