Nikhil Suryawanshi is a distinguished expert in machine learning, artificial intelligence, and data engineering. He has significantly contributed to advancing these fields. Throughout his career, Nikhil has been pivotal in the planning and design of systems that utilize machine learning algorithms and AI applications to tackle intricate challenges and enhance data processing workflows. His proficiency has enabled the organization to make better-informed decisions. Furthermore, Nikhil’s initiatives to optimize processes have minimized system downtime and led to enhanced data accuracy.
Nikhil received the ā2019 Decan Times Awards for Achievement in Technology – e-Commerce Solutionā from Decan Times publications in recognition of his research titled āPredicting Consumer Behavior in E-Commerce Using Recommendation Systemsā published in September 2019. His accomplishments and scholarly contributions have garnered recognition from Forbes tims magazine. The research examines the expansion of e-commerce platforms and emphasizes the necessity of understanding consumer behavior to enhance customer satisfaction and drive business success. Recommendation systems play a crucial role in predicting customer preferences and providing personalized product suggestions. The study offers a comprehensive review of existing literature on recommendation techniques, including content-based approaches, hybrid models, and collaborative filtering. It also highlights significant advancements such as the use of deep learning, trust-based filtering, and context-aware models. Building upon these foundational elements, the research proposes an innovative methodology that integrates consumer behavior analysis with advanced machine learning techniques to more accurately predict preferences. The expected results indicate that this model will improve recommendation accuracy, effectively address challenges such as data sparsity and evolving user preferences and enhance overall customer engagement within e-commerce environments.
Nikhil’s enthusiasm for the optimal use of technology motivated him to expand his research on the application of IoT devices for accurate air quality predictions in urban settings. The study titled āAir Quality Prediction in Urban Environment Using IoT Sensor Dataā published in May 2020, serves as a preliminary analysis demonstrating methodologies that can produce reliable Air Quality Index (AQI) predictions. These predictions are essential for aiding policymakers and public health officials in formulating effective air quality management strategies. As concerns regarding environmental health have intensified, the need for precise air quality monitoring and forecasting has surged. This study proposes a methodology for predicting AQI based on pre-existing data, rather than solely relying on real-time data from IoT sensors. The proposed system employs various machine learning techniques, including XGBoost, Neural Networks, and Linear Regression, to explore the relationships between air pollution indicators and AQI values. Key components of the methodology include data preprocessing, standardization, and the division of the dataset into training and testing sets. Although the system has yet to be implemented, preliminary evaluations indicate that these models could yield accurate AQI predictions, significantly benefiting public health authorities and policymakers in their efforts to establish effective air quality management strategies.
Nikhil Suryawanshi has consistently demonstrated a keen interest in and active engagement with research focused on the integration of technology within the healthcare sector. This passion led him to publish his study titled āPredicting Mental Health Outcomes Using Wearable Device Data and Machine Learningā in March 2021. The article introduces a machine learning-based framework intended to forecast mental health outcomes by utilizing data collected from wearable devices. This framework is designed to evaluate physiological and behavioral metrics, such as heart rate, sleep patterns, and activity levels, sourced from these devices. The core components of the framework include data preprocessing, feature extraction, and model training, employing a variety of machine learning algorithms, including Random Forest, Support Vector Machine, XGBoost, and Logistic Regression. These algorithms are combined through a voting-based ensemble classifier to improve the accuracy of predictions. While the system has yet to be implemented, the expected results suggest that this approach will enhance prediction reliability and offer real-time insights into mental health issues. The proposed system aspires to aid in the early detection of mental health disorders, thereby enabling timely interventions and tailored care.
Nikhil expanded his research on the application of machine learning to the material science domain and in March 2022 he successfully published the research titled āPredicting Material Properties Using Machine Learning for Accelerated Materials Discoveryā. The swift prediction of material properties has emerged as a crucial element in expediting the discovery and development of materials, propelled by innovations in machine learning and data-centric approaches. This paper introduces an innovative system designed for predicting material properties through machine learning techniques, providing a scalable and effective framework for exploring new materials with enhanced characteristics. The system utilizes extensive datasets, feature engineering, and a variety of machine learning models, including Kernel Ridge Regression, Random Forest, and Neural Networks, to forecast material properties such as thermal conductivity, elastic modulus, and electronic bandgap. By incorporating physics-based insights into the machine learning models, the proposed system improves the accuracy and interpretability of the predictions. The findings demonstrate that this system can substantially decrease the time and expenses associated with material discovery while achieving high levels of prediction accuracy. This approach can transform materials science by allowing researchers to identify promising material candidates in silico, thereby facilitating advancements in energy, electronics, and sustainable materials.
Neural is driven to persist in his research endeavors to discover solutions and innovative approaches that can facilitate substantial progress within a competitive environment. His objective extends beyond merely improving existing practices; he is also committed to embracing advanced technologies that enable both businesses and individuals to function more efficiently and maintain their competitive edge in the contemporary landscape.
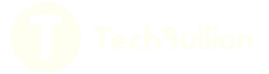