In this modern era, Venkata Krishna Pradeep Mattegunta, a seasoned analytics professional, explores the transformative power of predictive technologies in reshaping retail decision-making. With extensive experience in data-driven systems, his work highlights the convergence of AI, machine learning, and human strategy in the modern marketplace.
A New Era of Informed Merchandising
Retail is undergoing a quiet revolution. Today’s retail landscape is increasingly shaped by predictive analytics, a sophisticated blend of statistical modeling, machine learning, and AI integration. By converting vast volumes of data into actionable insights, predictive merchandising analytics enables precision in forecasting demand, managing inventory, setting prices, and customizing promotions. These tools empower retailers to proactively adapt to changing consumer behavior and economic conditions, allowing for more agile operations.
Forecasting with Foresight
Time-series forecasting models such as ARIMA allow retailers to predict demand with accuracy rates surpassing 90%. These systems rely on inputs like historical sales, weather forecasts, and social media sentiment, recalibrating projections every few hours. The result? Reduced stockouts, leaner inventories, and working capital efficiencies—often saving millions annually. Forecasting has also evolved to incorporate real-time signals, enabling more precise responses to market dynamics.
Pricing at the Speed of Thought
Dynamic pricing powered by real-time analytics has turned pricing strategy into a competitive advantage. By analyzing price elasticity across thousands of SKUs, retailers fine-tune prices based on demand and customer behavior. These adjustments deliver measurable financial results: 3–8% increases in revenue and 5–10% boosts in profit margins. Competitive price monitoring further ensures alignment with market expectations.
Smarter Shelves and Assortments
Predictive analytics determines not just what to sell, but where to place it. Product assortment tools integrate demographic and purchasing data to tailor mixes per store. Planograms—visual shelf layouts—are optimized using insights from in-store behavior, leading to higher sales per square foot and reduced unsold stock. Algorithms now factor in foot traffic patterns and product affinities to maximize placement effectiveness.
Promotions with Precision
Retailers spend up to 20% of revenue on promotions. Predictive models analyze timing, channel, and discount depth to forecast promotional lift with up to 90% accuracy. Personalization engines generate thousands of targeted offers daily, raising redemption rates and delivering stronger promotional ROI. Retailers also use historical performance to refine campaign strategies.
Knowing the Customer—Deeper and Smarter
Customer segmentation has evolved beyond demographics. Retailers leverage machine learning to categorize shoppers based on behavior and purchasing history. Techniques like k-means clustering identify nuanced segments, improving response rates by nearly 50% over traditional methods. Retailers can now tailor messaging, products, and offers to each segment’s specific needs.
Behavioral Insights: Anticipating Every Move
Predictive analytics anticipates shopper behavior—whether completing a purchase or abandoning a cart. Behavioral models track dozens of signals, enabling marketing teams to guide customer journeys. Churn models identify at-risk customers weeks before they leave, improving retention by up to 50%. These tools enhance lifetime value by ensuring proactive engagement.
The Human Side of Automation
Algorithms handle vast data, but human judgment remains crucial. Successful retailers balance automated systems for tactical decisions with human insight for strategic calls. Hybrid approaches outperform either extreme, particularly where historical data is scarce or context is critical. Teams trained in analytics contribute significantly to long-term adoption.
Ethical Footing in a Data-Driven World
Ethical considerations are rising in predictive merchandising. Fair pricing, transparency, and consumer consent are essential to trust. Only a third of retailers have formal ethics frameworks, but industry leaders are adopting explainable AI systems aligned with regulatory expectations. Responsible data governance is becoming a standard practice across competitive retailers.
What Lies Ahead
Future advancements promise more personalization and responsiveness. Real-time analytics platforms process tens of thousands of events per second. Edge computing enables rapid decision-making, while automated machine learning shortens model development cycles. Challenges remain, especially in using unstructured data and identifying causality. Visual AI and journey mapping are also expanding their role.
In conclusion, Predictive analytics is no longer a niche tool—it is the nerve center of modern retail strategy. By blending technical capability with organizational insight, it unlocks efficiencies and enhances customer engagement. As retailers evolve, the vision laid out by Venkata Krishna Pradeep Mattegunta illustrates what is possible when data meets purpose.
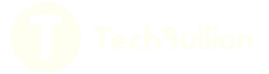