The digital era has witnessed unprecedented technological advancements, with artificial intelligence emerging as one of the most transformative forces. Within this rapidly evolving landscape, researchers like Venkata Bharathula Siva Prasad Bharathula have made notable contributions to the development and refinement of Large Language Models (LLMs).
Understanding Reward Models
Reward models serve as a bridge between human expectations and machine-generated responses. These models are trained on extensive datasets containing human feedback, where evaluators rank responses based on their quality, relevance, and ethical considerations. By systematically incorporating human preferences, reward models refine AI decision-making, ensuring outputs that align with user intent while minimizing misinformation or biased responses.
Training Reward Models for Accuracy and Alignment
Developing effective reward models involves multiple training techniques. Traditional methods rely on direct human feedback, where raters assess model-generated responses based on predefined criteria. More advanced techniques incorporate Constitutional AI, which embeds ethical principles into training data, ensuring that AI systems maintain integrity while delivering accurate information. The integration of implicit user interactions, such as analyzing engagement metrics, further enhances reward model performance. However, ensuring that these models do not reinforce unintended biases remains a challenge.
Reinforcement Learning: Enhancing AI Adaptability
Reinforcement Learning from Human Feedback (RLHF) has emerged as a groundbreaking approach in AI fine-tuning. This method enables LLMs to evolve based on human responses, adapting dynamically to deliver more contextually relevant outputs. RLHF follows a structured process that begins with a pre-trained language model, followed by the incorporation of reward models, and concludes with reinforcement learning techniques that optimize AI behavior over time.
The Evolution of Reinforcement Learning Strategies
Recent advancements in reinforcement learning have introduced more robust algorithms for training AI models. Techniques such as Proximal Policy Optimization (PPO) help fine-tune AI systems, allowing them to balance accuracy with ethical considerations. The optimization process includes continuous feedback loops, where AI-generated responses are evaluated and adjusted accordingly. This iterative refinement ensures that models do not just provide factually correct answers but also align with human expectations of fairness and clarity.
Constitutional AI: A New Paradigm for Ethical AI
One of the most notable innovations in AI training is the introduction of Constitutional AI. This approach embeds explicit behavioral guidelines into reward models, ensuring that AI responses adhere to ethical boundaries while maintaining high accuracy levels. Research has demonstrated that models trained with constitutional constraints achieve a remarkable 95% alignment with predefined ethical guidelines, reducing instances of harmful or misleading outputs. By structuring reward signals to reinforce positive behaviors, AI models can maintain consistency across diverse applications.
Addressing the Challenges in AI Fine-Tuning
Despite these advancements, challenges persist in reward model robustness and scalability. One major issue is the degradation of model performance when faced with unfamiliar data. Studies indicate that even the most sophisticated reward models experience up to a 40% drop in accuracy when handling out-of-distribution inputs. Additionally, training large-scale language models demands significant computational resources, making optimization a key area of focus for future research.
Optimizing AI for Future Applications
Efforts to refine AI fine-tuning methodologies have led to the exploration of novel optimization techniques. Researchers have proposed distributed training frameworks that can reduce processing time by up to 60%, making large-scale AI systems more efficient. Moreover, advancements in preference learning methodologies suggest that integrating uncertainty estimates can improve model robustness, ensuring that AI systems better understand nuanced human expectations.
The Future of AI Alignment
As AI technology continues to evolve, the synergy between reward models and reinforcement learning will play a crucial role in shaping its trajectory. The ongoing refinement of Constitutional AI, optimization algorithms, and preference learning techniques will be instrumental in developing AI models that are both powerful and ethically aligned.
In conclusion, Venkata Bharathula Siva Prasad Bharathula’s contributions provide a roadmap for future research, emphasizing the need for continuous innovation in AI training methodologies. With these advancements, AI systems are poised to become more reliable, transparent, and aligned with human values, paving the way for a future where artificial intelligence serves as a responsible and trustworthy tool for society.
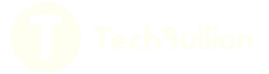