Expert analysis by Maksim Kariagin
***
Transportation is standing at the threshold of a sea change: driven by data science and machine learning. Among the active uses of these state-of-the-art technologies for servicing both vehicles and infrastructure, predictive maintenance plays a great role. Predictive maintenance works on real-time data and machine learning models with rich analytics to provide estimates of failures that might occur in the equipment. It reduces downtime, improves safety, and lowers operation costs.
Predictive maintenance is already in use by several firms like Siemens, which is using it to maintain railway systems continuously. This has reduced the incidences of sudden failure of rail and enhances efficiency. BMW applies predictive maintenance to its line of vehicles by analyzing real-time data from sensors to predict and rectify issues that may cause costly repairs. UPS uses it to maintain fleets of trucks, just as Delta Airlines does for aircraft to maintain high performances with minimum downtime. Below, we take a look at how machine learning is revolutionizing predictive maintenance in transportation and reduces the risk on all levels.
The Importance of Predictive Maintenance in Transportation
In transportation, whether it be logistics, public transit, railways, or aviation, maintenance is the key to continued smooth operations of both the vehicle and infrastructure. Traditional maintenance strategies, such as reactive and preventive maintenance, have been effective, yet more often than not they lead to unnecessary downtime or, even worse, unexpected breakdowns, which may seriously affect safety and operations.
With condition-based maintenance, the problem is solved by providing companies with the ability to anticipate equipment failures even before they occur. With machine learning algorithms and data from sensors, historical performance, and environmental conditions, transportation providers are able to achieve patterns predictive of potential issues long in advance. This method, however, has indicated significant advantages throughout the sector.
According to Deloitte’s report, predictive maintenance will lower breakdowns of equipment by as much as 70% and reduce maintenance costs by up to 25%. Besides, McKinsey adds that predictive maintenance can increase asset availability by 20%, while reducing maintenance labor time by 50% to bring improvement in operations efficiency with minimal downtime. These indicators and metrics can give good insight into predictive maintenance that avoids costly breakdowns while strengthening safety and efficiency in the transport systems.
How Machine Learning Powers Predictive Maintenance
These may include but are not limited to the following data sources for machine learning models in predictive maintenance:
IoT Devices and Sensors Data: Continuous flow of data from sensors and IoT devices integrated into the vehicle or infrastructure pertaining to parameters such as temperature, vibration, pressure, and fluid level.
Historical Maintenance Records: Previous data from maintenance activities can be used to train machine learning models to identify patterns in recognitions for future failures.
Environmental Data: External factors, such as weather conditions, road quality, and traffic patterns, can also be fed into the predictive models for a more accurate assessment of potential risks.
Collected data is analyzed using machine learning algorithms-such as regression models, decision trees, or neural networks to identify complex patterns. After that, such models can make predictions regarding when and where repairs may be needed. Such predictions allow maintenance scheduling whereby repair or replacement of parts by transportation companies could be done before failures actually take place.
Benefits of Machine Learning to Predictive Maintenance
Reduced Downtime
Probably the most important benefit of predictive maintenance is that it reduces unplanned downtime. The ability to anticipate failures before they actually occur helps schedule transportation enterprise maintenance during planned periods of time, minimizing chances of operational disruption. This is important in industries like logistics and public transportation, where delays may have a cascading effect on supply chains and commuters.
Improved Safety
Predictive maintenance has enormous potential to improve safety by mitigating risks before accidents happen. For example, in any mode of transportation, brake, engine, or control system failure would have disastrous implications. It is now possible to detect health deterioration through a machine learning model of the critical systems in question and appropriate preventive action on the part of transportation providers in order to avoid accidents and ensure safety on the part of both passengers and operators.
Several companies and organizations utilize the full capability of machine learning models to ascertain the deterioration in system health and, correspondingly, take appropriate preventive action with a view to ensuring the safety of passengers and operators. General Electric uses its platform “Predix” to monitor locomotive systems in its fleet of locomotives; monitoring the output from thousands of sensors that predict potential failures in train operations. Similarly, Hitachi Rail uses AI-powered predictive maintenance in monitoring the components of trains, such as brakes and engines, to ascertain whether they are worn out before an accident happens. Moreover, Toyota deploys the technology to monitor early signs of failure in manufacturing equipment to ensure that vehicles are safe by preventing such malfunctions from ever reaching the consumer. These examples really drive home how vital a role machine learning is playing in improving safety via predictive maintenance in many transportation fields.
Cost Savings
Traditional maintenance strategies are often driven to over-maintenance, where components are replaced or repaired too early, or under-maintenance, where issues are allowed to progress to the point of failure. Predictive maintenance will have repairs done only when required, thus optimizing maintenance cost. It also serves to extend the life of key assets, further reducing long-term costs.
Greater Efficiency
By nature, machine learning models learn continuously with new data coming into them, and hence, they become more accurate with time. All these elements enable the transportation companies to fine-tune their effective maintenance strategy with a view to raising the efficiency of operations. Minimizing downtime and averting expensive breakdowns are the ways transportation providers make sure that their fleet and infrastructure operate at peak performance.
Applications of Predictive Maintenance in Transportation
Automotive and Fleet Management: Equipped with machine learning, fleet operators such as UPS and FedEx are able to monitor in real time the health of their respective fleets of moving vehicles. By running predictive maintenance, companies can optimize vehicle uptime while reducing fuel consumption and improving safety on the road. For instance, UPS reported a 10% decrease in labor hours in line with the 9.9% decline in U.S. average daily package volume because of its predictive maintenance efforts.
Aviation: Boeing and Airbus are using machine learning technology in monitoring critical components’ health like an engine and landing gears in aviation. Airlines analyze data from sensors to forecast the time of failure of parts so that they can schedule maintenance in advance. Such an approach has been able to reduce in-flight failures drastically, which means lesser delays and improvement in passenger safety. Predictive maintenance, for example, has been implemented by Delta Airlines, allowing them to reduce aircraft maintenance costs.
Rail: Predictive maintenance is applied by operators such as Amtrak and CSX in rail infrastructure monitoring, signaling systems, and rolling stock. They predict the failures in the rail infrastructure by integrating advanced analytics with machine learning, hence decreasing the risk of accidents.
These various applications illustrate how predictive maintenance will affect changes in the transportation sector, from improving safety and reliability to enhancing operational efficiencies in all types of travel.
AWS and Predictive Maintenance Solutions
Among the most popular platforms for building machine learning models regarding predictive maintenance is Amazon Web Services-AWS.
AWS IoT Analytics-this service will build the ability to collect, process, and analyze IoT data on the following use case: sensor data from vehicles and infrastructure.
Amazon Kinesis: Allows ingesting and analyzing in real time sensor data generated by transport companies. This is pretty important for some applications related to real-time predictive maintenance, when immediate action might be justified.
AWS Lambda: Serverless computing-performs machine learning workflows anytime certain events occur, such as detecting abnormal behavior by a sensor.
Predictive maintenance in transportation will continue to expand with the ongoing evolution of machine learning models and data analytics tools. A few critical patterns to watch out for include:
Edge Computing: Edge computing will enable data coming from sensors to be processed locally, enabling real-time predictions without needing to send data to the cloud. This will be specifically important for autonomous vehicles and other time-sensitive applications.
AI-Powered Diagnostics: The next generation predictive maintenance solution will include full-functioning AI capable of diagnosing the root cause of a pending failure and providing recommendations on repairs or replacements in specifics.
Integrated Platforms: As the industry matures, we expect to see more predictive maintenance platforms integrated-tie IoT, machine learning, and cloud services into unified solutions and making the adoption of these technologies seamless for transportation providers.
Conclusion
This will turn predictive maintenance in transportation into new ways of mitigating risks, improving safety, and cutting costs. As companies increasingly exploit real-time data and predictive algorithms, they will advance in better management of assets and infrastructure to ensure that vehicles remain operational and safe for passengers and operators alike.
Predictive maintenance in the future will grow notably. According to the grand view research report, the Global predictive maintenance market size was valued at USD 7.85 billion in 2022 and is expected to grow at a compound annual growth rate (CAGR) of 29.5% from 2023 to 2030 since IoT and AI technologies may be increasingly adopted in several industries, transportation included. Other sources such as the Artesis report also cement this by establishing the expected growth to reach USD 28.2 billion by 2026.
By embracing such solutions, it means the future of transportation stands at the threshold of a much safer, highly efficient, and reliable future in which predictive maintenance becomes integral to operations, thereby enhancing service delivery and customer satisfaction.
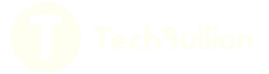