In 1997 when Deep Blue, a supercomputer, beat the then chess champion, Garry Kasparov, we all were taken aback. That was more than 20 years ago and most of us did not even know that computers like that even existed.
In late 2017, AlphaZero taught itself how to play chess under just four hours and beat the world’s then best chess-playing computer program. Remember, AlphaZero, the game-playing AI created by DeepMind, was not taught any domain knowledge but the rules of the game.
Such is the power of machines to learn and improvise and industries across the world are tapping a machine’s ability to learn and improve from its experience without being explicitly programmed. And the banking industry is at the forefront of embracing this innovation.
Understanding Machine Learning
Before discussing how any industry is making use of machine learning to combat fraud, let us understand the basics. As the name suggests, machine learning (ML) is an application that can learn from its experiences without being explicitly programmed. The applications can access data and use it to learn and improve themselves.
Various industries are using ML too for operations such as identifying email spam, providing a proper recommendation of the product to the customers, and offering an accurate medical diagnosis. For example, Coca Cola is using machine learning for product development. Using the data that they did collect from various soda fountain dispensaries, they were able to tell which flavor was preferred by most people. It helped them to launch the ‘Cherry Sprite’ nationally.
It is also instrumental in solving the most significant problem in any business – fraud. Fraud prevention has been a lot easier with the advent of machine learning and artificial intelligence (AI). There are so many transactions that people are doing online these days. Their money is at risk if proper security measures are not in place.
Here is another instance of how ML applications are being used to mitigate fraud. Huawei Technologies is using the translytical database to identify frauds in real-time. They are using a machine learning model that analyses the transactions that are both approved or declined. It is easy for the machine to figure out transactions that are fraudulent using this data.
How Machine Learning Applications Help Beat Fraud
Humans are not that great when it comes to checking a large quantity of data. Machines can do a better job than humans when it comes to this task. They are good at recognizing the user patterns. Companies can easily predict fraud in a massive volume of transactions using cognitive computing technology. The below example can validate the statement.
CitiBank is one of the first banks that have invested heavily on Feedzai, a machine learning product, to prevent fraud. It is quite helpful in identifying fraud in real-time. This particular software can do analysis on large scale. The best part is that it can identify and eradicate fraud not only in online banking but also offline banking.
Advantages of Using Machine Learning Applications
Increases Speed of Verification
In a system that bases primarily upon rules, things can get too complicated and verifying large data requires a lot of time. Merchants prefer to get their money faster and they will be super happy when there is a system in place that can check the enormous volumes of data in just a few milliseconds. Detecting fraud is going to be easy and simple when you pick this option. Real-time verification of a large number of transactions is only possible with the ML-based system
Easy to Maintain and Cost Effective
A machine learning application is going to perform a lot better when you feed in a lot of data. In systems that are rule-based, to maintain a fraud detection system, companies have to spend a lot of money. But, when it comes to ML, things are going to be a lot easy and cost-effective. The more data that you are going to feed to the systems is going to help the machines to function more efficiently. Differentiating good and bad transactions become a lot simpler when you do this thing.
Machines Are Efficient
Machines that receive proper training are going to perform a lot better than humans. They can do the repetitive job of data analysis with ease. Machines are going to escalate all the cases that need human intervention promptly. Preventing fraudulent transactions from happening is going to be easy because they are going to recognize non-intuitive and subtle patterns without any difficulty.
Machines Are the Future
Cybercriminals are smart and are using advanced tools and strategies to carry out their fraudulent activities. No matter how efficient your in-house fraud team is, they are not going to find fraudulent transactions easily as things are going to become more complicated.
AI and machine learning is the future, and hence, financial institutions and other industries should rely upon ML when it comes to preventing fraud. These systems can quickly learn the patterns and behavior of people doing frauds and shield your organization against such things.
Conclusion
Many companies are still wondering whether it is wise for them to invest in AI and ML. It is for sure going to be a huge investment. However, looking at all the benefits that an organization or an institution is going to get and the money they are going to save in the future using ML applications is unfathomable. Hence, it is time to embrace ML in order to safeguard the money and data of the customers and the brand’s reputation.
About Melissa
Customer data is the lifeblood of your organization. But it goes bad (up to 25% per year). Much of it is incorrect, outdated, or unstructured and fragmented across multiple systems – preventing operational efficiency, successful marketing and sales efforts, and a rewarding customer experience.
For more than thirty years, Melissa has been a leading provider of global identity verification and data quality solutions. Using cutting-edge technology and multi-sourced global reference data, we provide the solutions to support your Know Your Customer (KYC) initiatives, prevent fraud, reduce costs and improve fulfilment – at every point of the data chain.
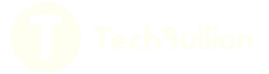