In the era of digital transformation, real-time data processing is revolutionizing how businesses harness information for strategic advantage. Santhosh Kumar Rai, an expert in cloud-based data analytics, explores this dynamic shift in computing paradigms. His research sheds light on the evolution from traditional batch processing to high-speed, cloud-powered real-time analytics, redefining enterprise decision-making.
The Shift from Batch to Stream Processing
The transition from batch to stream processing is revolutionizing data analytics. Traditional batch processing, which analyzes data at scheduled intervals, is giving way to real-time stream processing, driven by the demand for instant insights in finance, retail, and manufacturing.
By processing data as it is generated, real-time systems cut latency from hours to milliseconds, enabling swift responses to market fluctuations, security threats, and operational inefficiencies. This shift enhances decision-making, boosts efficiency, and provides enterprises with a competitive edge in dynamic environments.
Key Innovations in Cloud-Powered Real-Time Processing
Modern cloud platforms revolutionize real-time processing with scalable, distributed frameworks. Stream processing engines and serverless architectures remove on-premises constraints, enabling businesses to analyze millions of data points per second seamlessly. These innovations enhance agility, efficiency, and scalability, ensuring optimal resource utilization without infrastructure concerns. By leveraging cloud-native solutions, organizations gain instant insights, improve decision-making, and accelerate response times.
The shift from traditional architectures to cloud-powered real-time analytics fosters operational flexibility and cost efficiency. As data volumes grow, advanced cloud technologies empower businesses to harness real-time intelligence, driving innovation and competitive advantage in an increasingly data-driven landscape.
The Role of Edge Computing
Edge computing optimizes real-time processing by analyzing data closer to its source, reducing latency, conserving bandwidth, and improving system reliability. Instead of relying on centralized cloud servers, edge devices process information locally, enabling faster decision-making. This is especially valuable in IoT-driven industries, where sensor data must be processed instantly for predictive maintenance and autonomous operations. By minimizing data transmission delays and enhancing responsiveness, edge computing supports critical applications in manufacturing, healthcare, and smart cities, ensuring efficient and reliable system performance.
AI-Driven Real-Time Analytics
AI-driven real-time analytics is transforming data processing by embedding machine learning models into streaming pipelines. This enables businesses to detect patterns, predict trends, and automate decision-making with unparalleled speed and accuracy. In banking, AI swiftly identifies fraudulent transactions, reducing risks and enhancing security. In retail, real-time analytics optimizes inventory management, preventing stock shortages or overages.
By continuously analyzing incoming data, AI ensures rapid insights and adaptive responses, improving operational efficiency across industries. From predictive maintenance in manufacturing to personalized recommendations in eCommerce, AI-powered real-time analytics is revolutionizing how organizations leverage data for smarter, faster decision-making.
Overcoming Challenges in Real-Time Data Processing
Real-time data processing offers significant advantages but comes with challenges like scalability, data integrity, and cost efficiency. Handling high-speed data streams demands resilient architectures that ensure reliability without overloading resources. Organizations must implement optimized data storage solutions to efficiently manage growing data volumes while maintaining integrity. Additionally, real-time monitoring frameworks play a crucial role in detecting anomalies, preventing bottlenecks, and optimizing performance.
To balance efficiency and cost, businesses should leverage cloud-native solutions, stream processing technologies, and intelligent caching mechanisms. By integrating these strategies, organizations can enhance real-time data processing capabilities, ensuring seamless operations and actionable insights without unnecessary expenditures.
Industry Applications and Future Prospects
Real-time data processing is transforming industries by enabling proactive decision-making. In financial services, fraud detection systems analyze transactions within milliseconds, preventing unauthorized activities. In manufacturing, predictive maintenance powered by real-time data prevents costly downtime. Retailers leverage dynamic pricing and personalized recommendations based on real-time consumer behavior, enhancing customer experiences.
As the landscape evolves, advancements in serverless computing, AI integration, and edge analytics will further streamline real-time data processing. The continuous innovation in cloud technologies ensures that businesses of all sizes can leverage high-speed data analytics to drive growth and operational excellence.
In conclusion, Santhosh Kumar Rai‘s insights underscore the significance of real-time processing in shaping the future of enterprise intelligence. With organizations increasingly relying on instant data insights, cloud-based real-time analytics is set to become a cornerstone of digital transformation, enabling businesses to make faster, data-driven decisions and stay ahead in a competitive landscape.
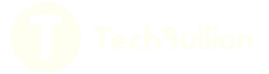