Quantum Machine Learning (QML) is pushing the boundaries of artificial intelligence, merging quantum mechanics with advanced algorithms to tackle complex problems with unmatched efficiency. Sunny Guntuka, an expert in the field, explores how tools like Quantum Support Vector Machines (QSVM) and Quantum Neural Networks (QNN) are unlocking new possibilities across fields such as material science, cryptography, and optimization—while also addressing the challenges to fully harnessing QML’s transformative power.
The Foundations of Quantum Computing in Machine Learning
At the heart of QML are quantum bits, or qubits, which are fundamentally different from classical bits. While classical bits exist in a binary state (0 or 1), qubits can exist in multiple states simultaneously due to superposition, vastly increasing the potential for data processing. Quantum gates and circuits further enhance this potential by enabling operations on qubits, creating entanglement and parallel processing capabilities. However, challenges such as decoherence—where quantum information is lost due to environmental interference—pose obstacles to maintaining qubit stability, requiring robust error correction mechanisms.
Quantum Support Vector Machines: A New Paradigm in Classification
QSVMs are a quantum-enhanced version of classical Support Vector Machines (SVMs), algorithms used for data classification and regression. By employing quantum algorithms, QSVMs handle computationally intensive processes like kernel evaluation more efficiently. This capability enables them to process high-dimensional data faster than classical SVMs, potentially improving accuracy for complex classification tasks. However, QSVMs face limitations, especially regarding hardware stability and scalability, which are crucial for tackling large datasets.
Quantum Neural Networks: Expanding the Depth of AI
Quantum Neural Networks (QNNs) extend neural networks into the quantum domain, combining quantum principles like superposition and entanglement with deep learning architectures. Unlike classical neural networks, QNNs can process information in parallel, exploring more complex data patterns. With architectures like variational quantum circuits and hybrid models, QNNs promise improved generalization capabilities, which are vital for complex tasks like image recognition and quantum simulations. Nevertheless, practical implementation of QNNs faces challenges due to hardware constraints and the need for further algorithmic development.
Material Science: Unlocking Molecular Simulations
QML offers transformative applications in material science, especially for molecular simulations. Quantum algorithms allow researchers to model complex quantum systems, like molecules and materials, at scales beyond classical computing. By predicting molecular properties, QML accelerates material discovery for energy storage and nanotechnology. In pharmaceuticals, QML’s predictive capabilities could expedite drug discovery, identifying effective compounds faster.
Cryptography: Strengthening Security for the Quantum Era
QML holds great promise in cryptography, especially in advancing Quantum Key Distribution (QKD) protocols. By optimizing quantum states for key generation, QML strengthens QKD’s resilience against noise and eavesdropping, enhancing security. It also aids post-quantum cryptography, developing algorithms resistant to both classical and quantum attacks, bolstering future communication systems against quantum threats.
Optimization: Solving Complex Problems More Efficiently
Optimization problems like the Traveling Salesman Problem (TSP) and portfolio optimization are critical across industries. QML, using algorithms such as the Quantum Approximate Optimization Algorithm (QAOA), offers more efficient solutions than classical methods. In finance, QML enhances portfolio optimization, helping investors explore diverse asset configurations for improved returns and risk management.
Challenges in Quantum Machine Learning
Despite QML’s promise, key challenges persist. Quantum hardware currently faces limitations in qubit counts and coherence times, complicating large-scale applications. Research in quantum error correction and noise mitigation is crucial for reliable computing. Additionally, efficient hybrid quantum-classical systems and interfaces are essential for integrating quantum tech into broader machine learning applications.
The Future of QML: Integration and Innovation
As quantum hardware advances, hybrid quantum-classical systems are expected to rise, allowing quantum processors to handle specific tasks within classical machine learning frameworks. This integration maximizes quantum benefits while retaining classical resources. Ongoing algorithm development and strong benchmarking will be essential to evaluate QML’s performance and identify areas with exponential advantages.
In conclusion, Sunny Guntuka highlights Quantum Machine Learning as a frontier in tech innovation, merging quantum computing with AI to address complex challenges. Despite hurdles like hardware limits and error correction, QML’s applications in material science, cryptography, and optimization show great promise. As research progresses, integrating quantum and classical systems will be crucial in unlocking QML’s full potential.
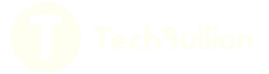