A Convergence of Two Powerful Technologies, Shashank Chaudhary, a researcher at the forefront of computational science, explores the dynamic fusion of quantum computing and artificial intelligence in his latest work. His insights shed light on how Quantum Machine Learning (QML) is redefining the way complex problems are approached, leveraging principles such as superposition and entanglement for computational efficiency.
Breaking Free from Classical Limitations
Traditional machine learning models have made significant strides in AI, yet they encounter growing computational constraints. Deep learning models require exponential processing power, particularly when dealing with large datasets. QML introduces a paradigm shift by utilizing quantum states to process multiple computations simultaneously, breaking through the bottlenecks that hinder classical systems. This revolutionary approach enables unprecedented computational efficiency, offering transformative potential for artificial intelligence applications while dramatically reducing resource requirements across diverse implementations.
The Mechanics of Quantum Neural Networks
Quantum Neural Networks (QNNs) function similarly to classical neural networks but harness quantum principles to enhance computational performance. Superposition allows QNNs to handle multiple states at once, vastly improving data processing efficiency. Furthermore, entanglement enables deeper connections between quantum units, allowing models to capture complex data relationships more effectively than their classical counterparts. These quantum advantages facilitate exponential speedups for specific computational tasks, particularly in optimization problems and pattern recognition scenarios. QNNs show remarkable promise for analyzing high-dimensional datasets where classical approaches struggle with dimensionality barriers, potentially revolutionizing fields from drug discovery to financial modeling.
Variational Quantum Circuits: A Hybrid Approach
Variational Quantum Circuits (VQCs) present a hybrid model that integrates quantum computation with classical optimization techniques. By using parameterized quantum gates, these circuits adjust model parameters dynamically, making them particularly suited for noisy intermediate-scale quantum (NISQ) devices.
Unlocking New Potential with Quantum Kernel Methods
Quantum Kernel Methods provide a robust way to enhance machine learning by mapping data into high-dimensional quantum feature spaces. Unlike classical kernel methods, which struggle with computational feasibility in high-dimensional scenarios, quantum-based approaches unlock exponentially larger feature spaces, enabling more effective pattern recognition and classification tasks.
Transforming Drug Discovery with Quantum Simulations
One of the most promising applications of QML lies in pharmaceutical research. Quantum-powered molecular simulations can model chemical interactions at an unprecedented level, accelerating the drug discovery process. By overcoming the computational limitations of classical simulations, QML has the potential to significantly reduce the time and cost associated with developing new treatments.
Reinventing Cryptography for the Quantum Age
The impact of QML extends beyond AI applications, reaching into the realm of cryptography. Quantum algorithms such as Shor’s algorithm threaten classical encryption methods, making post-quantum cryptography an urgent area of focus. By leveraging quantum key distribution (QKD), secure communication methods can be developed to withstand potential threats from quantum computers.
Optimizing Decision-Making in Finance and Logistics
Quantum optimization algorithms are transforming financial modeling and logistics. Portfolio optimization benefits from quantum approaches by processing vast amounts of financial data more efficiently. Similarly, supply chain logistics gain from quantum-enhanced routing algorithms, which can solve complex scheduling and distribution challenges beyond the capabilities of classical methods.
Overcoming the Challenges of QML Implementation
Despite its promise, QML faces several technical hurdles. Quantum decoherence, which leads to loss of quantum state integrity, remains a major obstacle. Limited qubit connectivity and error correction issues further complicate the deployment of quantum algorithms. Advances in quantum control systems and optimized qubit architectures are essential for ensuring stable and reliable quantum computations.
The Road Ahead: Bridging Theory and Practical Applications
Future research in QML is focused on improving quantum hardware scalability, designing more efficient quantum algorithms, and identifying practical use cases where quantum computing offers a definitive advantage. By developing hybrid models that integrate classical and quantum computing, researchers aim to create a smooth transition towards full-scale quantum AI applications.
In conclusion,As the boundaries of AI and quantum computing continue to expand, the possibilities for innovation grow exponentially. Shashank Chaudhary’s exploration of QML underscores its potential to reshape industries ranging from healthcare to finance. While challenges remain, the ongoing advancements in quantum technology promise a future where computational limitations are no longer a barrier to progress.
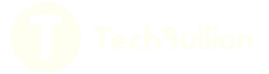