In this rapidly evolving digital landscape, modern data infrastructure serves as the cornerstone of today’s technological operations, demanding unparalleled reliability and operational excellence. Madhukar Dharavath presents an innovative framework that revolutionizes conventional maintenance approaches by shifting from reactive and preventive methods to sophisticated AI-powered predictive models. Through the implementation of state-of-the-art artificial intelligence, this groundbreaking system enables precise failure forecasting, optimizes maintenance planning, and enhances resource allocation. By identifying and addressing potential challenges before they materialize, the framework ensures continuous operational efficiency and strengthens the resilience of intricate data infrastructures, promoting sustainability and enduring performance.
The Evolution of Maintenance Approaches
Traditional maintenance methods, reactive or preventive, fall short for modern systems. Reactive approaches address issues post-failure, causing costly downtime, while preventive schedules risk unnecessary maintenance and wasted resources. AI-driven predictive maintenance, powered by real-time data and machine learning, anticipates failures, enabling timely interventions. This reduces costs, minimizes downtime, and optimizes resources, revolutionizing operational efficiency and reliability.
Multi-Modal Frameworks for Enhanced Insights
At the heart of this innovation lies a multi-modal framework that synergizes data streams from sensors, system logs, and historical records to provide a comprehensive view of system health. Advanced methodologies, including time-series analysis for tracking temporal patterns, natural language processing (NLP) for extracting insights from unstructured data, and reinforcement learning for adaptive decision-making, seamlessly integrate to detect potential failure points. This approach optimizes maintenance schedules dynamically, ensuring system reliability, reducing downtime, and enhancing operational efficiency through intelligent automation.
Leveraging AI for Optimal Decision-Making
AI technologies like deep learning and natural language processing (NLP) revolutionize predictive maintenance by enhancing accuracy and efficiency. Deep learning identifies intricate non-linear patterns, enabling the precise detection of anomalies in complex manufacturing systems. Simultaneously, NLP processes unstructured data such as maintenance logs, extracting valuable insights that were once overlooked. Together, these advanced tools optimize maintenance workflows, minimizing false positives, uncovering root causes, and reducing downtime. This synergy not only boosts operational reliability but also lowers costs and improves overall productivity.
Integration of IoT and Edge Computing
The framework effectively combines Internet of Things (IoT) devices with edge computing to facilitate seamless, real-time data collection and processing. By integrating IoT sensors, it enables the capture of detailed performance metrics from diverse systems, offering comprehensive insights. Edge computing processes this data locally, reducing reliance on centralized systems, which minimizes latency and ensures faster, more efficient decision-making. This synergy between IoT and edge computing enhances system monitoring, enabling timely responses to critical events and driving operational efficiency and performance.
The Role of Swarm Intelligence
Inspired by the principles of swarm intelligence, this approach employs collective optimization techniques. For instance, distributed sensing networks adjust data collection priorities dynamically, ensuring optimal resource allocation. Additionally, anomaly detection mechanisms collaborate across nodes to pinpoint potential issues, reducing false alarms and enhancing reliability.
Challenges in Implementation
Despite its promise, the adoption of predictive maintenance systems is not without hurdles. Integrating these frameworks with legacy systems, ensuring data quality, and managing resource constraints are significant challenges. Addressing these requires robust planning, organizational readiness, and ongoing refinement of AI models to adapt to new failure modes and data patterns.
Impact on System Reliability
The framework’s deployment has demonstrated remarkable results. From reducing unplanned downtime to enhancing equipment lifespan, AI-driven predictive maintenance redefines operational efficiency. The ability to predict failures with 90-98% accuracy underscores its transformative potential, offering a compelling alternative to traditional strategies.
Future Horizons
As technology evolves, the integration of blockchain for secure maintenance records and explainable AI for transparent decision-making promises to further enhance predictive maintenance systems. These advancements will pave the way for more trustworthy and autonomous maintenance ecosystems, ensuring robust infrastructure reliability.
In conclusion, Madhukar Dharavath‘s groundbreaking contributions emphasize the transformative potential of AI in redefining maintenance practices, seamlessly integrating technological sophistication with operational excellence. His innovative approaches provide organizations with the tools to achieve unparalleled reliability, optimize efficiency, and proactively manage data infrastructures. This research not only addresses critical challenges in intelligent infrastructure management but also sets a visionary framework for future innovations, paving the way for continued advancements and elevating standards across the field of data-driven maintenance and infrastructure optimization.
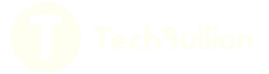