In the modern digital era, enterprises are navigating increasingly complex infrastructures, requiring more sophisticated approaches to reliability and system management. Madhu Sudhan Nanda, an expert in Site Reliability Engineering (SRE), highlights how predictive analytics is revolutionizing SRE practices. His research demonstrates how predictive analytics enables a shift from reactive maintenance to proactive system management, enhancing operational resilience and minimizing downtime.
The Shift from Reactive to Proactive SRE
Traditional SRE practices focused on incident resolution after failures occurred. Engineers spent a significant portion of their time addressing system downtimes and performance bottlenecks. However, with the integration of predictive analytics, organizations have significantly reduced manual interventions, improving efficiency and system resilience.
Predictive models now analyze historical data and detect patterns, allowing teams to anticipate failures before they disrupt operations. Organizations leveraging predictive analytics report a 76% reduction in system downtime and an 89% decrease in false positive alerts, streamlining operations while ensuring reliability.
Machine Learning Enhancing Pattern Recognition
Advanced machine learning models are at the core of predictive analytics in SRE. By analyzing millions of telemetry data points in real time, these models identify system anomalies with an accuracy rate of over 94%. This enhanced pattern recognition enables early detection of performance degradation and potential failures.
Moreover, modern systems integrate ensemble methods like Long Short-Term Memory (LSTM) networks and Gradient Boosting Decision Trees, improving anomaly detection accuracy to 96.7%. These advancements contribute to higher uptime and better incident prevention strategies.
Automating Incident Response for Faster Resolutions
Automation plays a crucial role in predictive analytics-driven SRE. Organizations implementing automated response mechanisms have achieved a 73% reduction in manual interventions. Dynamic scaling, traffic management, and configuration updates are now handled autonomously, reducing Mean Time to Resolution (MTTR) from 42 minutes to just 12 minutes.
Automated systems can dynamically allocate resources based on demand, reducing overprovisioning costs by 34% and optimizing cloud infrastructure expenses. This shift not only enhances operational efficiency but also minimizes financial overhead.
Capacity Planning and Resource Optimization
Predictive analytics has revolutionized capacity planning by providing accurate forecasts for resource utilization. Organizations using these models have reduced infrastructure costs by 31% while maintaining 99.99% service availability. Forecasting models predict CPU, memory, and storage needs with over 92% accuracy, helping enterprises optimize resource allocation and prevent bottlenecks.
These advancements have led to an 89% decrease in capacity-related incidents, ensuring that enterprises can scale efficiently without unnecessary resource expenditure.
Environmental and Economic Impact
Beyond improving operational efficiency, predictive analytics in SRE has substantial environmental and economic benefits. Data centers utilizing predictive analytics have reduced energy consumption by 43%, translating to an estimated 12.7 million metric tons of CO2 emissions saved annually. Moreover, optimized infrastructure planning has led to a 52% reduction in hardware waste, promoting sustainable technology practices.
From an economic perspective, organizations adopting predictive analytics have seen a 47% decrease in operational costs, making enterprise-level reliability more accessible to small and medium businesses. Additionally, predictive analytics-driven automation has contributed to the creation of 175,000 new jobs in the digital services sector.
The Future of Self-Healing Systems
The integration of AI-driven predictive analytics is paving the way for self-healing systems. Future advancements in AI models are expected to improve failure prediction accuracy by 23% while reducing computational resource demands by 68%. Enhanced automation will further decrease system downtime, with next-generation orchestration technologies achieving near-perfect decision-making accuracy.
Edge computing is also playing a critical role, reducing response latency by 82% and enabling real-time system adaptations. With these advancements, predictive analytics will continue to drive innovation in SRE, ensuring higher reliability and efficiency for digital services worldwide.
In conclusion, predictive analytics is transforming Site Reliability Engineering by enabling proactive failure prevention, resource optimization, and improved efficiency. Through machine learning, automation, and advanced forecasting, organizations can enhance system reliability and resilience. Despite implementation challenges, the long-term benefits justify the investment, making predictive analytics integral to modern SRE strategies. As businesses increasingly adopt these technologies, the vision of self-healing systems is becoming a reality. Madhu Sudhan Nanda’s research underscores its critical role in driving digital transformation and operational excellence in today’s dynamic IT landscape.
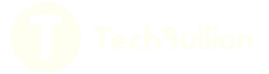