When your dashboard warns of a failing water pump weeks before it seizes, or rush-hour lights retime themselves moments before gridlock, you’re seeing predictive analytics at work inside today’s connected-vehicle networks. A new paper by mobility researcher Utham Kumar traces how machine-learning models are moving from lab demos to real- world fleets, slicing accidents, downtime and emissions in the process.
From data exhaust to early warnings
Modern vehicles generate gigabytes of telemetry every hour. Supervised learning tools mine that stream for patterns—abnormal vibrations, erratic fuel burn—that once hid in plain sight. According to Kumar’s survey of European logistics operators, predictive maintenance algorithms cut on-road breakdowns 40 percent and saved one firm $1.5 million a year in repair bills.
Safer streets through behavioral AI
Inside the cabin, cameras and lidar feed models that watch for fatigue and aggressive maneuvers. Systems trained in millions of driving hours now flag risky lane changes or distracted texting seconds before they escalate. One commercial fleet reported a 25 percent crash reduction in its first year of deployment; highway pilots integrating similar tech into emergency-braking stacks have halved rear-end collisions in testing.
City planners join the loop
Predictive analytics aren’t confined to the car. In Los Angeles and Singapore, AI traffic brains ingest signal data, ride-share pings and weather feeds to forecast jams and tweak lights in real time—knocking 20-plus minutes off commutes and trimming urban CO₂ by
double digits. Stockholm’s pilot program even pairs the models with dynamic tolling: when congestion looms, peak-hour prices rise automatically and traffic drops 12 percent.
The fleet-management dividend
For logistics chiefs, predictive routing delivers the next leap. Algorithms juggle live traffic, customer windows and weather to compress mileage; one MIT-backed study cited by Kumar records fuel savings of 12 percent and an 18 percent cut in delivery-route length.
Add vehicle-health forecasts, and operators not only arrive faster but spend 30 percent less time parked in repair bays.
Ethical code and legal code
Big wins bring big questions. Bias in training data can misjudge driving styles; privacy advocates worry about the constant upload of location and biometrics. Regulators are
scrambling to pin liability when an algorithm’s “best guess” goes wrong. Kumar argues that transparent, auditable models—plus GDPR-style safeguards—are non-negotiable if public trust is to keep pace with technical horsepower.
What’s next: talking asphalt and AI copilots
Research labs are already fusing predictive engines with natural-language interfaces so drivers—or autonomous stacks—can ask why a system chose to slow or reroute. The next wave links cars directly to pavement sensors and 5G curbside nodes, letting asphalt itself whisper warnings about black ice two blocks ahead. Deep-learning ensembles processing that mesh of data could, Kumar predicts, cut urban fatalities another 15 percent within a decade.
Bottom line: predictive analytics is transforming connected vehicles from reactive
machines into proactive partners. For city halls chasing cleaner air, fleets hunting margin, and drivers wanting safer roads, the message is identical: the smartest mile is the one your AI already saw coming.
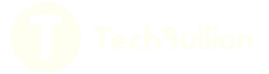