Baltimore, MD – In an era defined by decarbonization imperatives and digital disruption, renewable-energy specialist and data-engineering leader Venkata Narasareddy Annapareddy is charting a new course for how utilities, educators, and communities harness clean power and cloud intelligence. His latest peer-reviewed study—“Integrating AI, Machine Learning, and Cloud Computing to Drive Innovation in Renewable Energy Systems and Education Technology Solutions”—unveils an integrated blueprint that marries deep-learning models, hybrid Petri-net control, and elastic cloud services to create autonomous, cost-effective energy ecosystems and workforce-ready learning platforms .
Decarbonizing the Grid with Intelligent Hybrid Models
Most renewable sites still rely on rule-based logic or manual oversight, leaving turbines and solar arrays vulnerable to intermittency and weather shocks. Annapareddy’s research introduces Hybrid Petri Net architecture capable of modelling more than 1,000 discrete and continuous states within a smart city power network, then auto-synthesizing optimal control policies for wind, solar, and storage assets .
Key advances
- Portable micro-wind and mobile PV prototypes (5–10 kW fixed, 1.5–1.6 kW mobile) engineered for rapid deployment in remote communities
- Leakage-power–aware control that prolongs battery life in hybrid environments
- Early-fault detection that flags anomalies before catastrophic failure through multi-sensor data fusion
AI & Cloud: The New Backbone of Renewable Operations
By off-loading heavy analytics to Infrastructure-, Platform- and Software-as-a-Service layers, Annapareddy shows how utilities can slash capital costs while scaling real-time forecasting and edge decision-making . His framework calls for:
- Federated learning pipelines that safeguard data privacy yet improve wind-speed and solar-irradiance prediction accuracy
- Dynamic API orchestration for seamless device onboarding across global IoT fleets
- Self-healing cloud clusters that recover from faults autonomously, guaranteeing uptime for critical grid services
Predictive Analytics for Reliable Energy Production
Deep-neural-network ensembles, support-vector machines, and random-forest regressors deliver sub-hour forecasting granularity, enabling grid operators to synchronize generation with fluctuating demand . Annapareddy’s team further proposes robustness-assisted training to curb the notorious “prediction-drift” common in time-series RNNs .
Building a Future-Ready Workforce through EdTech
Recognizing the talent gap in AI-driven energy, the paper outlines a three-tier educational strategy:
- Tier 1: Core programming and engineering skills
- Tier 2: Domain projects on energy-management and distribution systems
- Tier 3: Curiosity-driven pathways that steer students toward autonomous systems and ML research
A cloud-multi-agent platform mines big-data textbooks, highlights knowledge gaps, and generates ChatGPT-powered study guides—bringing personalized STEM education to any connected classroom .
About Venkata Narsareddy Annapareddy
Currently a Enterprise Application Lead at SunPower, UKG, Capgemini, and DXC. His portfolio includes 5 articles on AI-enabled energy innovation .
“Integrating AI with renewable infrastructure is no longer optional—it is fundamental to achieving resilient, carbon-neutral grids and empowering the next generation of engineers,” Annapareddy states in his paper .
A Vision for Autonomous, Resilient Energy Ecosystems
From predictive-maintenance agents that alert field crews in seconds to data-driven microgrid controllers that balance prosumer loads in real time, Annapareddy’s holistic roadmap positions AI, ML, and cloud computing as the cornerstone of a sustainable energy future. Utilities, educators, and policymakers seeking to accelerate their net-zero agendas now have a pragmatic playbook—one that blends technical rigor with an unwavering commitment to societal impact.
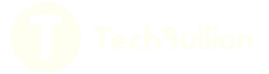