In the dynamic realm of radiology, the advent of artificial intelligence (AI) has ushered in a transformative era. Over 300 AI-powered tools have been greenlit by the FDA, marking a significant stride in the field. As Anant Madabhushi, a distinguished professor at the Emory University School of Medicine’s Department of Biomedical Engineering, points out, these tools primarily serve as a beacon for physicians in the labyrinth of cancer diagnosis and treatment. However, an innovative AI tool, christened ‘Sybil’, is carving a unique niche by forecasting future cancer risk.
Sybil’s raison d’être is to pinpoint potential hotspots where cancer may germinate, thus empowering physicians to detect it at the earliest possible juncture. This is of paramount importance in the case of lung cancer, which is most amenable to treatment when detected early, as per Dr. Kim Sandler, an Associate Professor of Radiology at Vanderbilt University Medical Center. Yet, the early detection of lung cancer is akin to finding a needle in a haystack due to the invisibility and intangibility of the lungs. The only feasible method for early detection is through a CT scan. By the time symptoms such as persistent coughing or difficulty breathing surface, the cancer usually has advanced to a stage where treatment becomes more challenging.
Research has illuminated that screening with low-dose CT scans can curtail lung cancer mortality by 24%, as it facilitates earlier detection when the disease is more susceptible to treatment. Sybil, the AI tool, could potentially amplify early detection rates and thereby enhance survival rates, Sandler suggests.
In a parallel development, recent studies have unveiled that deep learning, a subset of machine learning, can prognosticate the progression of preinvasive lesions in lung cancer, specifically squamous cell carcinomas (SCC). SCC, which has a stronger correlation with smoking, constitutes about 20% of lung cancers and often originates in the basal cells of the bronchial mucosa.
Managing SCC is a Herculean task as 30% of its preinvasive precursor lesions, known as squamous cell carcinoma in situ (SCIS), regress, thereby complicating clinical decisions. While low-dose helical CT screening has been increasingly employed to boost SCC patient survival, it may not always detect preinvasive tumors. Hence, the ability to predict which SCIS will metamorphose into SCC would be a boon for future monitoring and treatment.
Deep learning, leveraging artificial neural networks (ANN), can distill patterns from intricate data, making it particularly apt for histopathology and medical imaging. A sudy spearheaded by a team of scientists employed deep learning on H&E stained images to discern which SCIS lesions evolve into SCC and which regress. Despite being trained using a relatively small dataset by the standards of a deep convolutional neural network (DCNN), the model demonstrated remarkable efficacy in identifying the progression of SCIS into SCC.
This study, as noted by Eziokwu Fab-Emerenini MBBS in his article on Gilmore Health News, marks the first endeavor to predict the progression of a preinvasive lesion using deep learning on pathology images. It posits that deep learning could potentially serve as a cost-effective, high-throughput technique to provide patients and clinicians with more prognostic data, and could be integrated into the screening toolset. This pioneering study is anticipated to catalyze further research into the application of deep learning on whole slide images (WSI) to predict the progression of other preinvasive precursor lesions.
In summation, the horizon of lung cancer detection and treatment is bright, illuminated by AI tools like Sybil and the application of deep learning techniques. These advancements are not only augmenting the capabilities of radiologists but also paving the way for more precise, early detection and treatment of lung cancer. As these technologies continue to evolve and mature, they hold the potential to revolutionize our approach to lung cancer, ultimately leading to improved patient outcomes. As we stand on the cusp of this exciting new era in oncology, it is clear that the fusion of AI and deep learning will play a pivotal role in shaping the future of lung cancer detection and prognosis.
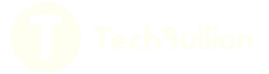