In today’s rapidly evolving digital landscape, edge computing is revolutionizing the way data is processed, offering a significant shift from traditional cloud-based architectures. This evolution addresses key challenges such as latency, bandwidth, and data security by moving processing closer to where data is generated. In this article, Santhosh Reddy Thuraga explores how advancements in hardware, software, and AI integration are driving the adoption of edge computing across industries. From healthcare to manufacturing, edge computing is transforming real-time decision-making and operational efficiency, while also presenting challenges that must be addressed for widespread implementation.
Bringing Processing to the Edge
Traditional cloud-based architectures, while robust, have shown limitations in latency and bandwidth for real-time applications. Edge computing addresses these challenges by shifting data processing closer to the point where it is generated. This paradigm reduces lag time, conserves bandwidth, and enhances data security. By processing data locally, edge computing allows for faster decision-making and more responsive systems, which is critical in industries where real-time action is essential.
Unlocking Hardware Potential for Edge Computing
Advancements in hardware are driving the growth of edge computing. Power-efficient processors now enable edge devices to handle complex tasks with minimal energy use. Specialized AI chips, like NVIDIA’s Jetson series, boost machine learning performance directly on edge devices, reducing reliance on central servers and improving real-time processing. Additionally, 5G networks provide the high-speed, low-latency connections needed for real-time applications, which is crucial for industries like autonomous vehicles that require fast data processing for safe navigation.
Software Innovation at the Edge
New software tools and frameworks tailored for edge computing are emerging, optimizing data handling with minimal resources. Containerized systems and distributed databases are designed to improve efficiency at the edge. Lightweight operating systems like Azure Sphere enable secure and efficient edge device operations. Another key innovation is distributed machine learning, such as Federated Learning, which trains models across multiple edge devices without centralizing data. This enhances privacy and speeds up learning in critical environments like healthcare.
AI and Machine Learning: The Edge Advantage
The integration of AI and machine learning into edge computing is a major advancement, enabling real-time data analysis at the source. This is particularly impactful in healthcare, where wearables can monitor patient vitals and respond instantly without relying on central servers. Lightweight machine learning models, designed for edge devices, maintain efficiency by using techniques like model quantization and transfer learning. These methods optimize large AI models for resource-limited environments, ensuring fast responses and minimizing latency in critical applications.
Transforming Industry Applications
Edge computing is transforming industries such as healthcare, manufacturing, and automotive. In healthcare, edge devices enable instant processing of patient data, allowing for immediate diagnoses while enhancing data security. Wearable devices provide real-time health insights, which can be life-saving in critical situations. In manufacturing, edge computing revolutionizes production by analyzing IoT sensor data to predict maintenance needs, optimize processes, and prevent costly downtimes. The automotive industry also benefits, with edge computing powering autonomous vehicles that rely on real-time, low-latency data from multiple sensors to make split-second driving decisions, ensuring safety and efficiency.
Challenges and the Road Ahead
Despite the promise of edge computing, several challenges must be addressed for widespread adoption. Security is a major concern, as the distributed nature of edge systems increases vulnerability to attacks, making encryption and secure boot processes essential for safe operation. Interoperability is another issue, as diverse edge devices and systems need seamless communication, requiring standardized protocols and open frameworks. Additionally, resource constraints on edge devices limit complex task handling, a challenge best addressed by hybrid edge-cloud architectures, where tasks are dynamically distributed based on complexity for optimal resource utilization.
As businesses continue to generate ever-increasing amounts of data, edge computing offers a solution that addresses the limitations of traditional cloud architectures. By processing data closer to the source, edge computing enhances efficiency, security, and real-time decision-making across various sectors. Santhosh Reddy Thuraga‘s insights into the technological advancements driving this innovation highlight the transformative power of edge computing. As these technologies continue to evolve, we can expect even greater integration of AI and machine learning at the edge, unlocking new opportunities for industries worldwide.
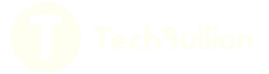