Multimodal AI is driving a new wave of innovation in artificial intelligence by integrating diverse data types into cohesive systems. In this article, Kiran Chitturi explores the breakthroughs that are shaping the future of technology, highlighting the transformative potential of multimodal AI across industries.
Bridging Modalities for Enhanced Intelligence
Multimodal AI integrates text, images, and audio, surpassing single-modal systems with 85%+ accuracy in tasks like visual question answering. Using neural embeddings and attention mechanisms, it enables real-time decision-making and cross-domain applications, revolutionizing personalization and autonomous navigation.
Revolutionizing Applications Across Domains
The impact of multimodal AI is evident in various practical applications. Cross-modal search systems, for instance, have transformed how users retrieve information by integrating text and image inputs. With a precision rate of over 82%, these systems excel in delivering relevant results, even for complex queries.
Multimodal AI excels in personalized recommendations, processing 8,500 interactions/second with 87% accuracy. It outperforms traditional models, solving the “cold-start” problem with 45% better relevance and significantly enhancing user engagement.
Healthcare has also seen significant advancements. By integrating visual scans and textual patient data, multimodal AI has improved diagnostic accuracy by 72%. These systems have the potential to enhance decision-making in critical environments, reducing errors and improving patient outcomes.
Advancing Model Architectures
Multimodal AI owes much of its success to breakthroughs in model architectures. The Large Language and Vision Assistant (LLaVA), for example, demonstrates exceptional performance in tasks that require both visual and textual reasoning. Using shared vector spaces, LLaVA ensures semantic consistency across modalities, enabling it to perform complex visual dialogues and multi-turn interactions effectively.
Another significant innovation is the adoption of contrastive learning techniques, which align large datasets of images and text. These models achieve outstanding results without extensive retraining, significantly reducing resource requirements. This approach has made multimodal AI more scalable and accessible, ensuring its adoption across a range of industries.
Real-time applications, in particular, have benefited from these advancements. With response latencies as low as 180 milliseconds, multimodal systems are well-suited for time-sensitive tasks like autonomous vehicle operations and dynamic search platforms.
Overcoming Current Challenges
Despite its remarkable progress, multimodal AI faces several challenges. The high computational costs associated with training and deploying large-scale models remain a significant barrier. Current systems often require millions of dollars in resources to achieve state-of-the-art performance. Furthermore, maintaining alignment across diverse data types introduces latency issues, especially in real-time applications.
Data preprocessing also presents a major obstacle. Multimodal systems must handle diverse formats while ensuring semantic consistency, which demands extensive computational resources. Studies indicate that these systems require significantly more preprocessing time and storage compared to unimodal approaches, limiting their scalability in resource-constrained environments.
Emerging Solutions and Future Directions
Efforts to address these challenges are showing promise. Advanced techniques like sparse attention mechanisms and model quantization have demonstrated the potential to reduce computational costs by up to 60%, without compromising performance. These innovations are paving the way for lightweight and efficient multimodal systems that can operate in diverse environments.
In the education sector, multimodal AI is enabling adaptive learning platforms that cater to individual student preferences. These systems achieve personalization accuracy rates of 87%, while reducing response times by 45%. Such advancements illustrate the potential of multimodal AI to create more inclusive and effective solutions for a wide range of applications.
A Transformative Vision for AI
Multimodal AI represents a fundamental shift in how machines interact with and understand the world. By integrating diverse data types, these systems provide smarter, more responsive technologies that address complex challenges in industries such as healthcare, education, and automation. Their ability to harmonize inputs across modalities is redefining human-machine interaction and enhancing decision-making processes.
The innovations discussed here highlight the profound potential of multimodal AI to transform industries and improve quality of life. As research continues, these systems will play an increasingly critical role in shaping the future of artificial intelligence.
In conclusion, Kiran Chitturi’s work in multimodal AI highlights its transformative potential, paving the way for smarter, more adaptive technologies that bridge data types and revolutionize human interaction with AI.
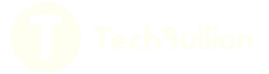