The University of Guelph (UoG) in Guelph, Ontario Canada has been awarded a prestigious grant from the Natural Sciences and Engineering Research Council (NSERC) to advance a cutting-edge carbon capture project. By blending quantum mechanics, atomistic simulation, thermodynamics, and Artificial Intelligence (AI), researchers at UoG aim to create a breakthrough predictive model for identifying new and effective Carbon Dioxide Removal solvents without the need for traditional trial-and-error experiments.
Why AI Matters
Artificial Intelligence, particularly machine learning (ML) algorithms like artificial neural networks (ANNs), is a game-changer in this project. It accelerates the discovery process by predicting crucial solvent properties based on molecular structure, sparing researchers from relying on slow, resource-intensive methods. AI models can sift through massive datasets to spot patterns linked to carbon capture capacity. In doing so, they streamline the search for promising solvents and complement quantum mechanical and atomistic simulations.
The Quantum Mechanics Advantage
Quantum mechanics offers precise insights into how CO₂ interacts at the molecular and atomic levels. This level of detail is vital to identifying solvents with the strongest CO₂ affinity. However, these calculations can be computationally heavy. Enter AI: machine learning narrows the field before quantum-based calculations begin, focusing resources on the most promising candidates first.
Atomistic Simulation for Deeper Insights
Atomistic simulations capture the nuanced behavior of individual atoms and molecules within solvents. By pairing these simulations with AI-generated predictions, the team can quickly evaluate an array of solvents, identifying molecular features that drive carbon capture. This method significantly reduces the time and computational power needed for exhaustive simulations.
The Role of Thermodynamics
The AI-aided quantum mechanics and atomistic simulation data are fed into a thermodynamic model as the final step in the theoretical framework. It predicts how solvents behave at different operating conditions such as temperatures, pressure and solvent composition.
A Predictive, AI-Enhanced Approach
Traditional carbon capture research often moves slowly because it relies on experimentally testing each potential solvent. This new predictive model, powered by AI, breaks that cycle. It can evaluate thousands of molecular structures without laboratory work, identifying top contenders for in-depth study—saving time, money, and effort.
Implications for Climate Goals
Innovative carbon capture methods are critical as governments, industries, and communities push to cut CO₂ emissions in line with net-zero targets. If successful, the University of Guelph’s approach could significantly reduce industrial emissions by making carbon capture technologies more efficient and affordable. Its AI-driven methods could even pinpoint novel solvent classes that existing techniques might never discover.
Industry and International Partners
Project collaborators include Delta Cleantech of Regina Saskatchewan and Natural Resources Canada laboratories in Ottawa ON and in Varennes Quebec. The project also involves international collaborators in Mexico, Germany and Switzerland. Critical to the Project’s success are its graduate students and postdoctoral fellow team members, shown below. Please contact us if you’re interested in joining this exciting venture!
Looking Ahead
Thanks to NSERC support, the University of Guelph is at the forefront of carbon capture innovation. By uniting quantum mechanics, atomistic simulation, thermodynamics, and AI, UoG researchers offer hope for a more sustainable future. Their work highlights the power of data-driven science to accelerate climate solutions and underscores the growing role AI will continue to play in one of the world’s most urgent challenges: mitigating climate change.
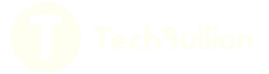