Solar energy has become one of the most promising renewable power sources globally. With increasing demand for clean energy, optimizing solar farm operations is critical. In this context, Robin Sarkar explores the revolutionary impact of predictive analytics and artificial intelligence (AI) on solar energy systems. Through innovative AI applications, solar farms can significantly improve efficiency, reduce operational costs, and boost energy production, accelerating the transition to sustainable energy.
Transforming Solar Energy Operations
The rapid growth of the solar energy market has made managing large-scale solar farms increasingly complex. Modern installations span vast areas with thousands of panels, presenting challenges in maintenance, performance, and grid integration. Predictive analytics powered by AI addresses these issues by leveraging IoT sensors and machine learning algorithms to analyze data. This enables solar operators to predict equipment failures, optimize performance, improve maintenance scheduling, and enhance energy production and grid integration, driving operational efficiency.
The Role of Data and Machine Learning
Predictive analytics for solar energy relies on continuous data collection from IoT sensors that monitor key metrics like power output, temperature, panel orientation, inverter performance, and environmental conditions. Large solar farms generate vast amounts of data daily, which is processed through cleaning, normalization, and feature extraction. Machine learning models, such as Random Forests and ANNs, analyze this data to forecast power output, detect degradation, and predict failures, enabling proactive maintenance and optimized energy production.
Proactive Maintenance and Performance Optimization
One of predictive analytics’ key advantages in solar energy is its ability to forecast equipment failures before they occur. Traditional solar farm maintenance relies on scheduled inspections, but this approach can result in unnecessary maintenance visits or missed opportunities to address equipment issues. Predictive models shift this paradigm by identifying early signs of potential failures, allowing maintenance teams to address problems before they lead to significant downtime.
For example, AI-powered systems can identify issues like inverter failures, power output reductions, or temperature fluctuations that signal maintenance needs. By intervening early, operators can reduce maintenance costs and improve equipment reliability. In addition to maintenance, predictive analytics also optimizes solar farm performance. Machine learning models forecast weather patterns and their impact on energy production, optimize the tilt angles of panels, and detect underperforming panels due to shading or dust accumulation, all of which contribute to maximizing energy yield.
Grid Integration and Energy Trading
Grid integration is a major challenge for solar energy providers due to the intermittent nature of solar power, which varies with weather. Predictive analytics addresses this by enhancing energy forecasting and grid stability. AI models analyze weather data and historical patterns to predict energy production more accurately, ensuring better alignment with grid demand. Additionally, AI systems optimize energy trading by forecasting market conditions and prices, enabling solar operators to make informed decisions, maximize revenue, and improve operational efficiency.
Overcoming Implementation Challenges
While predictive analytics offers clear benefits in solar energy, its implementation presents challenges. Data quality is a major hurdle, as sensor inaccuracies can lead to unreliable predictions. Additionally, integrating AI-driven systems with legacy infrastructure is complex, requiring significant investment in technology and training to ensure seamless deployment and optimal performance.
The solar energy sector also faces a skill gap in AI and data science, making it challenging to find qualified professionals. To address this, many organizations invest in cloud-based solutions, partner with academic institutions, and implement staff training programs, ensuring the successful adoption and integration of advanced technologies in solar operations.
The Future of Solar Energy
As the solar energy industry continues to expand, predictive analytics and AI will only become more critical. These technologies are not just improving current solar operations but also setting the stage for smarter, more efficient, and resilient solar power systems. With the potential to reduce costs, increase energy yield, and enhance grid stability, predictive analytics is a key driver of the future of solar energy.
In conclusion, Robin Sarkar’s research underscores the transformative role of predictive analytics and AI in optimizing solar energy systems. By overcoming operational challenges and unlocking new capabilities, these technologies are key to driving the global transition to a sustainable, clean energy future.
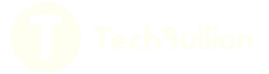