A New Era in Cloud Infrastructure Technology expert Vijayakumar Jayaseelan explores the transformative role of generative AI in optimizing hybrid cloud workloads. His insights shed light on how AI-driven innovations are reshaping cloud environments, enhancing security, and improving efficiency.
Predictive Resource Management: Moving Beyond Traditional Scaling
One of the most groundbreaking developments in hybrid cloud optimization is the use of generative AI for predictive resource management. Unlike traditional threshold-based scaling, AI-driven approaches analyze historical data, user behavior, and workload patterns to forecast demand. This allows real-time resource adjustments, ensuring that computing power is available when needed, reducing latency, and improving overall efficiency.
These advanced AI systems can identify subtle correlations between seemingly unrelated factors, such as time of day, geographic user distribution, and application-specific demands. By processing these complex variables, generative AI can proactively provision resources minutes or even hours before actual demand spikes occur. Furthermore, continuous learning algorithms refine prediction models over time, adapting to evolving business needs and technological changes.
Organizations implementing these solutions report up to 40% reduction in cloud spending while simultaneously enhancing performance metrics. The integration of these AI capabilities with existing cloud orchestration tools creates a self-optimizing infrastructure that can autonomously balance workloads across on-premises and multiple cloud environments, effectively eliminating the traditional trade-offs between cost, performance, and reliability that have long challenged IT operations teams.
Security and Risk Management: AI-Powered Threat Detection
Security remains a paramount concern in hybrid cloud deployments. AI-powered threat detection systems utilize machine learning to analyze vast amounts of security telemetry data. These intelligent models detect anomalies, identify potential vulnerabilities, and prevent cyber threats before they escalate. AI also plays a critical role in identity management, enforcing multi-factor authentication and zero-trust security models, thereby minimizing unauthorized access risks.
Network Optimization: Enhancing Performance with AI
Hybrid cloud environments rely on robust networking strategies to maintain performance across distributed systems. AI-driven software-defined networking (SDN) enables dynamic traffic routing, optimizing data flow between on-premises and cloud-based infrastructures. By analyzing real-time network conditions, AI ensures that latency-sensitive applications maintain high performance while minimizing unnecessary bandwidth consumption.
End-to-End Encryption: Securing Data Across Multiple Platforms
Data security in hybrid cloud environments is further strengthened through AI-driven encryption techniques. Advanced key management solutions separate encryption responsibilities between infrastructure providers and data owners, ensuring compliance with security standards while maintaining ease of access. AI enhances these processes by automating key rotations and securing data throughout its entire lifecycle.
Proximity-Based Workload Allocation: Improving Efficiency
AI algorithms now play a crucial role in workload placement by considering multiple factors beyond just geographic proximity. These systems analyze network congestion, compute availability, and application dependencies to dynamically adjust workload distribution. By intelligently positioning workloads closer to their data sources, AI reduces latency, improves response times, and enhances user experiences.
The Evolution of Autonomous Cloud Optimization
The shift towards self-optimizing cloud environments marks a major advancement in IT infrastructure. AI-driven systems continuously analyze performance metrics and automatically make adjustments to optimize workloads. This reduces manual intervention, allowing IT teams to focus on strategic initiatives rather than operational maintenance. Reinforcement learning further refines these capabilities, enabling autonomous systems to improve efficiency over time based on real-world data.
The Role of Quantum Computing in Hybrid Cloud Strategies
As quantum computing technology evolves, its integration with hybrid cloud infrastructures is expected to revolutionize computational capabilities. AI can help organizations prepare for quantum-readiness by identifying workloads that could benefit from quantum acceleration. AI-driven optimization frameworks will play a key role in bridging the gap between classical and quantum computing resources in future deployments.
Looking Ahead: Strategic AI Adoption in Cloud Environments
The integration of generative AI into hybrid cloud environments represents a significant step toward intelligent infrastructure management. Organizations adopting these technologies stand to benefit from increased efficiency, improved security, and reduced operational costs. As AI continues to advance, its role in optimizing cloud workloads will become even more integral.
In conclusion, Vijayakumar Jayaseelan emphasizes that organizations must strategically implement AI-driven cloud solutions to stay competitive. By leveraging AI-powered automation, security enhancements, and predictive analytics, businesses can build more resilient and efficient hybrid cloud ecosystems.
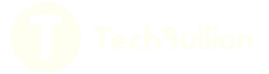