In the ever-evolving world of artificial intelligence (AI), the ability to make effective decisions is a cornerstone of innovation. AI-driven decision optimization is becoming increasingly crucial across industries, from marketing to automated systems. Huzaifa Fahad Syed, an expert in AI-driven decision strategies, explores the comparative strengths of Uplift Modeling and Reinforcement Learning (RL) in refining decision-making frameworks.
Measuring True Impact: The Essence of Uplift Modeling
Uplift Modeling is a predictive analytics technique that measures the causal impact of interventions, rather than just forecasting outcomes. It segments individuals based on their likelihood of response, enabling precise targeting. The process includes data collection, segmentation, model building, validation, and implementation. Widely used in marketing, it ensures promotional efforts reach those genuinely influenced, optimizing budgets and maximizing impact through data-driven decision-making.
Continuous Learning with Reinforcement Learning
Unlike Uplift Modeling, which provides a one-time analysis, Reinforcement Learning (RL) excels in dynamic environments through continuous adaptation. RL involves an agent interacting with its environment, refining strategies via a reward-based system, and learning iteratively. This makes it ideal for applications like real-time advertising, automated trading, and personalized recommendations. By dynamically adjusting suggestions based on user behavior, RL enhances engagement and satisfaction, making it a powerful tool for evolving, fast-paced decision-making scenarios.
Static vs. Dynamic: Choosing the Right Approach
One fundamental difference between Uplift Modeling and RL lies in the nature of their decision-making environments. Uplift Modeling is best suited for static environments where historical data provides reliable insights. It is particularly effective when evaluating the impact of a single intervention, making it ideal for controlled experiments and marketing strategies that require precise measurement.
In contrast, RL is built for dynamic and continuously evolving environments. It excels in scenarios where decisions need to be adjusted in real-time based on incoming data. For instance, in programmatic advertising, RL optimizes bidding strategies by learning from previous auction outcomes, ensuring maximum return on ad spend.
Data Requirements and Computational Complexity
Uplift Modeling requires large, structured datasets with clear treatment and control groups, relying on extensive historical data for accurate intervention assessment. Though computationally less intensive than RL, its effectiveness hinges on data quality and model assumptions. In contrast, RL can begin with minimal data but requires extensive environmental interactions to optimize decisions. Deep RL, integrating neural networks, handles complex scenarios but demands high computational power, making it ideal for applications prioritizing long-term adaptability over immediate precision.
Scalability and Adaptability in AI Systems
Uplift Modeling excels in scalability, efficiently processing large customer datasets for marketing and segmentation. However, its adaptability is limited, requiring re-modeling and additional data collection for each new intervention. In contrast, Reinforcement Learning (RL) offers exceptional adaptability, continuously refining decisions without new datasets. Yet, scaling RL to large environments is computationally intensive, demanding advanced optimization techniques. While Uplift Modeling handles scale well, RL’s flexibility comes at a higher computational cost.
The Future: Hybrid Approaches for Enhanced Decision Optimization
Although Uplift Modeling and RL serve different purposes, they can complement each other in AI-driven decision-making. Uplift Modeling can be used to identify initial target segments, which can then be fine-tuned by RL systems for ongoing optimization. Similarly, RL’s reward mechanisms can be informed by Uplift Modeling’s causal insights, creating a more efficient decision-making framework.
Future research in AI-driven decision-making is likely to explore hybrid models that combine the precision of Uplift Modeling with the adaptability of RL. Potential advancements include Causal Reinforcement Learning, which integrates causal inference principles into RL, and multi-armed bandit approaches that blend targeted intervention analysis with continuous learning.
In conclusion, as AI-driven decision optimization continues to evolve, the integration of Uplift Modeling and Reinforcement Learning presents a compelling approach for businesses seeking smarter and more adaptive systems. Uplift Modeling remains essential for accurately measuring the impact of interventions, while Reinforcement Learning’s ability to autonomously refine decision-making makes it well-suited for dynamic environments. As Huzaifa Fahad Syed’s research suggests, RL may eventually surpass traditional Uplift Modeling in many applications, offering greater adaptability and efficiency. The future of AI-driven decision-making lies in striking the right balance between measurement and continuous learning, unlocking new possibilities for intelligent automation.
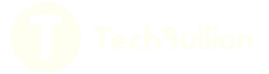