In this rapidly growing digital era Athul Ramkumar, a researcher from a leading US university, has published groundbreaking research that explores innovative techniques for deploying large language models (LLMs) on mobile and edge devices. His comprehensive study examines the delicate balance between computational demands and hardware constraints, opening new possibilities in mobile AI technology.
Revolutionary Compression Methods
The transition of powerful language models from cloud servers to personal devices marks a significant shift in AI technology. Advanced compression techniques, including pruning and knowledge distillation, are making it possible to reduce model sizes by up to 75% while maintaining over 90% accuracy. These methods carefully remove redundant parameters and transfer essential knowledge to smaller, more efficient models without significantly compromising performance. Through careful calibration and optimization, these compression techniques achieve substantial memory savings while preserving model capabilities, enabling sophisticated AI functions on everyday devices.
Smart Hardware Solutions
Specialized hardware accelerators are revolutionizing how AI models operate on mobile devices. Neural Processing Units (NPUs) integrated into modern smartphones can achieve several trillion operations per second while maintaining low power consumption. Field-Programmable Gate Arrays (FPGAs) offer flexible solutions that can be customized for specific model architectures, while Application-Specific Integrated Circuits (ASICs) provide unparalleled efficiency for AI computations. These hardware innovations are crucial for enabling real-time inference on resource-constrained devices.
Memory Management Innovation
Novel approaches to memory management are addressing one of the biggest challenges in mobile AI deployment. Advanced techniques like memory pooling and cache optimization are significantly reducing resource requirements. Dynamic memory management algorithms adapt to specific patterns of language models, while gradient checkpointing allows for efficient recomputation of intermediate results instead of storing them, dramatically reducing memory needs. These optimizations enable smooth operation of complex language models on devices with limited RAM, making sophisticated AI applications accessible to a broader range of mobile devices.
Privacy-First Processing
On-device inference is transforming how AI applications handle sensitive data. By processing information locally, these systems eliminate the need to transmit personal data to remote servers, addressing critical privacy concerns. This approach enables applications like real-time language translation and voice assistance while keeping user data secure and private. The shift to on-device processing also reduces latency and eliminates dependency on network connectivity, ensuring consistent performance across various usage scenarios. This privacy-centric approach is particularly valuable in scenarios involving sensitive personal or business information.
Efficient Architecture Design
Lightweight variants of transformer architectures are making LLMs more accessible for mobile use. These modified designs incorporate innovations like factorized embedding layers and reduced attention heads, significantly decreasing computational requirements while maintaining robust language understanding capabilities. Sparse attention mechanisms allow each token to focus on relevant information only, reducing unnecessary computations. These architectural improvements are complemented by advanced quantization techniques that further optimize model performance, enabling more efficient processing of complex language tasks.
Real-World Applications
The practical applications of these innovations are far-reaching. From real-time language translation for travelers to sophisticated text analysis for healthcare professionals, on-device LLMs are enabling new possibilities across various sectors. Smart home devices can now process complex voice commands locally, and industrial equipment can understand natural language instructions without relying on cloud connectivity. The technology also enables privacy-preserving text analysis for sensitive applications in healthcare, finance, and legal domains, revolutionizing how these industries interact with AI technology.
Future Outlook
The field continues to evolve with research focusing on improving energy efficiency and model adaptability.The development of hardware-aware neural architecture search techniques and automated optimization frameworks promises to make these systems even more efficient and accessible. Security measures are being enhanced to protect both the models and user data, ensuring responsible deployment of AI capabilities. These developments are paving the way for more sophisticated and secure AI applications on mobile devices.
In his concluding remarks, Athul Ramkumar emphasizes that these advancements represent just the beginning of a new era in mobile computing, where sophisticated AI capabilities become an integral part of our daily interactions with technology, all while maintaining privacy and efficiency.
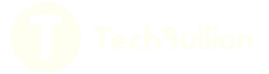