With over two decades of experience leading large-scale IT initiatives and developing growth strategies for Fortune 500 clients, Ganesh Masti has established himself as a thought leader in enterprise digital transformation. As a Senior Project Manager, he has spearheaded successful automation and data product solutions, while leading large teams and driving strategic decisions alongside C-level executives. His recent completion of an Executive MBA from the Stephen M. Ross School of Business, where he developed an innovative AI Blueprint for workforce development, has added fresh perspective to his technical leadership.
In this in-depth interview, Ganesh shares critical insights on enterprise AI adoption, drawing from his experience in building global teams and implementing transformative technologies. He addresses the hidden challenges organizations face, introduces frameworks for successful AI implementation, and offers a strategic vision for building AI literacy across organizational levels. His unique perspective, combining technical expertise with business acumen, provides valuable guidance for organizations navigating the complex landscape of AI transformation.
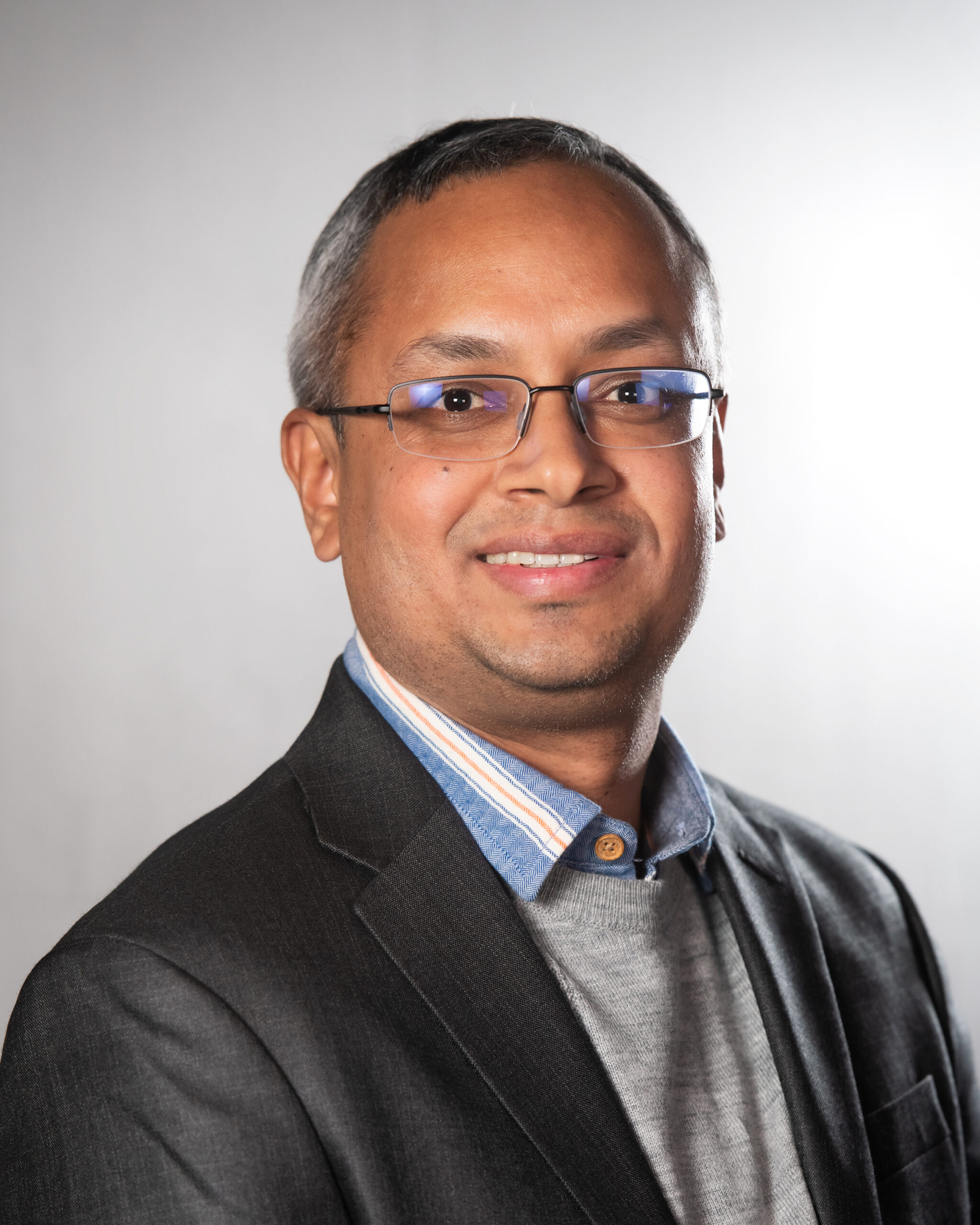
Ganesh Masti
Ganesh, you developed an innovative AI upskilling blueprint combining both academic and industrial insights. What hidden challenges did you uncover in enterprise AI adoption that most organizations overlook?
Both the kind of work we do and how we do that work have undergone significant changes. This is not new. Right now we are in an age, where AI represents the newest catalyst for change. To put it in perspective with a quote based on my discussions with academia & industry leaders:“Dating once relied on personal connections, but now it’s all driven by algorithms!”. We have seen this massive & rapid transformation in AI technologies that is giving rise to Industry transformation which is leading to workforce transformation. So as AI advances, the challenges of adoption evolve as well; for instance, responsibly implementing AI becomes crucial. Beyond ethical considerations, organizations must also navigate data privacy concerns, workforce upskilling, and integration with legacy systems to fully realize AI’s potential.
I’d summarize the hidden challenges as STAR: Strategy alignment, Tailored evaluation, Agile customization, and Risk management. First, one of the primary hurdles to start with that enterprises face, that is often-overlooked, that goes beyond mere technical implementation is the lack of cohesive AI strategy and alignment with business goals. Many organizations rush into AI adoption without fully assessing feasibility or return on investment (ROI), leading to disjointed implementations that fail to deliver value. Secondly, with the vast array of models and tools available, the challenge lies in identifying what works best for specific needs, which requires careful valuation and custom tailoring. However, this step is often overlooked due to the need for speed, limited skill sets, leading many to rely on out-of-the-box models that may not fully meet their unique requirements. Third is the need for essential skill sets and infrastructure that enable organizations to adapt swiftly and operate with agility. Although AI tools are widely accessible, the real challenge lies in bridging the skill gap essential for organizations to adapt quickly and effectively. Lastly, and the most important aspect that tends to get overlooked, is the risk associated with AI adoption (ASI): safeguarding the Security and Integrity of outcomes without compromising ethics or safety. In summary, the most significant hidden challenges lie in ensuring AI adoption is not just technically sound but also strategically aligned and ethically grounded, with a strong focus on managing risks to maintain the integrity and security of outcomes — elements often overlooked but essential for sustainable success.
Your experience spans both technical implementation and strategic planning. How do you approach building AI literacy across different organizational levels, from technical teams to executive leadership?
As AI continues to advance, it unveils a diverse range of roles, spanning from data scientists to business practitioners, with everyone requiring AI literacy at different levels. Building AI literacy across an organization is like constructing a resilient ecosystem, where each level plays an interconnected role in supporting AI-driven growth. To build AI literacy across an organization, I use a layered approach, ensuring that each level has the knowledge and skills necessary to contribute to AI-driven growth.
At the Strategic Layer – for Executive Leadership and Governance Teams, the focus is on the strategic implications of AI. This includes training on aligning AI initiatives with business objectives, understanding ROI, and establishing governance frameworks that prioritize ethics and security.This layer sets the vision and ethical standards that guide the organization.
For the Application Layer—comprising Mid-Level Managers and Business Practitioners—the training focuses on the practical application of AI within business processes. Here, I emphasize understanding AI’s role in enhancing operations, decision-making, and customer insights. Training includes identifying potential AI use cases in their functional areas, interpreting data-driven insights, and collaborating effectively with technical teams to ensure AI initiatives align with strategic objectives. This equips them to act as informed advocates and implementers of AI within their respective domains.
At the Execution Layer, technical teams get trained on developing expertise in machine learning, NLP, and robotics, enabling them to turn strategic goals into actionable AI solutions. This practical focus empowers them to drive innovation and execute AI initiatives effectively.
Finally, the Support Layer where we have foundational teams in traditional tech roles such as infrastructure, cloud, and cybersecurity learn how AI integrates with existing systems. They adapt their roles to accommodate and enhance AI’s impact, ensuring stability across the organization. By building AI literacy in these layers, I create a cohesive, well-prepared organization ready to harness AI’s potential sustainably.
Having led digital transformation initiatives generating multi-million dollar impacts, what are the most common misconceptions about enterprise AI adoption that you’ve encountered?
Misconceptions about AI adoption are indeed REAL. Let me break down what that means. REAL stands for Readiness,Enhancement vs Replacement, Alignment, Longevity
Readiness is often underestimated; many organizations think AI is plug-and-play, expecting immediate results. But truly successful AI requires solid infrastructure, skilled teams, and a mindset prepared for continuous learning and adaptation.Readiness is often underestimated; many organizations think AI is plug-and-play, expecting immediate results. But truly successful AI requires solid infrastructure, skilled teams, and a mindset prepared for continuous learning and adaptation.Then there’s Enhancement versus Replacement. There’s a misconception that AI will fully replace human decision-making. In reality, AI’s real strength is in enhancing human abilities by managing data-heavy tasks and providing insights, especially in complex scenarios. AI should work alongside humans, not replace them. In fact, as we continue to advance, real-time data streams from human interactions will increasingly feed into Large Language Models, making them some of the most reliable and valuable sources of data, offering the highest potential benefits. We then look at Alignment. Too often, alignment with strategic business objectives is seen as an afterthought, pursued only to enhance productivity after AI has been implemented. This leads to isolated, ineffective implementations that don’t deliver real value. For AI to truly benefit an organization, it needs to be integrated into core business strategies of these enterprises. And,Longevity is often misunderstood. Many organizations mistakenly view AI adoption as a one-time implementation, neglecting the fact that it requires ongoing commitment and evolution. To ensure lasting impact, organizations must prioritize continuous learning, scalability, and the iterative enhancement of their AI initiatives.
You’ve achieved significant cost reductions through strategic automation. How do you identify which processes are truly ready for AI enhancement versus those that need a more measured approach?
At the heart of identifying processes ready for AI lies there are many factors that need consideration. It’s essential to be really SMART when assessing processes for AI readiness. Here’s what I mean by SMART.
- S – Scalability: We evaluate whether the process can effectively scale with AI integration, processes that need and can handle increased demand or volume are ideal candidates for enhancement.
- M – Measurable Impact: It’s important to consider the potential for measurable improvements. We should prioritize processes that can demonstrate clear, quantifiable benefits from AI implementation.
- A – Alignment with Strategy: Ensuring that the process aligns with our organization’s strategic objectives is crucial. AI initiatives should support broader business goals to drive meaningful value.
- R – Readiness of Data: We must assess the quality and volume of data associated with the process. High-quality, abundant data is essential for effective AI training and outcomes.
- T – Technology Integration: Finally, we must assess how well the process can integrate with our existing systems and technologies. Seamless integration is vital for successful AI implementation.
In my view, when it comes to processes, scalability comes first.It’s essential to ensure that a process can effectively adapt to AI implementation and has the necessary prerequisites (such as data, standardization,compatibility with other processes etc.) to facilitate this transition successfully. This is due to the fact that even if a process is impactful but not scalable, then neither alignment nor measurable impact truly matters; without the ability to grow and adapt, any benefits derived from AI integration will be limited and unsustainable. Hence my point of view is that scalability must be the first consideration when it comes to processes to ensure that the organization can fully leverage AI’s potential over time. In the event that a process is not scalable, then in such cases, the process may need to be redefined to ensure it can grow and adapt.
As organizations rush to adopt AI, how do you balance the drive for innovation with the need for responsible AI implementation and governance?
To effectively balance the drive for innovation with the need for responsible AI implementation and governance, first adhere to the main principles of Responsible AI. These principles align closely with the Executive Order on Artificial Intelligence signed by President Biden, which emphasizes the importance of promoting safety, equity, and accountability in AI development. These principles include ensuring transparency and explainability of AI systems, prioritizing ethics and fairness to address biases, maintaining compliance with regulations, and implementing robust privacy and security measures. Additionally, organizations must establish accountability for AI outcomes and engage in continuous monitoring of AI systems to enhance safety and reliability. Responsibility precedes Speed when it comes to AI implementations. The English dictionary places R before S, and it’s essential for us to follow suit in AI; prioritizing Responsibility before Speed ensures our innovations are ethical, sustainable and don’t lead to unforeseen pitfalls.
Your recent completion of an Executive MBA brings fresh perspective to technical leadership. How has this business education influenced your approach to AI strategy and implementation?
My recent completion of an Executive MBA included an action-based learning project known as the Executive Map (ExecMAP). This project significantly enriched my perspective on technical leadership, particularly in shaping AI strategy and implementation. In this project, I had the opportunity to collaborate with a leading tech giant alongside my team to develop an AI Blueprint and introduce the LEADERS Framework, aimed at bridging the skills gap that persists in the industry today. This educational experience taught me the importance of aligning AI projects with broader business objectives, ensuring that our technology investments deliver measurable value. It also helped me with a practical application of the theoretical concepts learned in class, specifically with respect to, strategy and innovation management. It honed my leadership, strategic thinking, and project management skills.I gained invaluable insights into problem-solving and innovation in workforce development..The experience of collaborating with a leading tech giant on AI initiatives has sharpened my strategic planning, stakeholder engagement, and innovation skills. This unique exposure, particularly regarding how AI should be implemented across diverse industries such as healthcare, high-tech etc, has allowed me to consider different perspectives for shaping the future of AI implementations in these critical sectors.
Ganesh, you’ve successfully scaled teams and solutions in multicultural environments. What unique challenges arise when implementing AI initiatives across diverse global teams?
Implementing AI initiatives across diverse global teams brings unique challenges that extend beyond the technical aspects. From my experience,this shift will require organizations to solve some the following key challenges like:
- Investment in Upskilling workforce to ensure they have the necessary skills to leverage AI technologies effectively.
- Navigating cultural nuances and ensuring that AI systems are designed to be culturally sensitive and inclusive to ensure linguistic biases do not creep into AI models. This requires a careful examination of training data and the implementation of guidelines that promote fairness and equity in AI outputs.
- Ensuring Accountability: In a globally distributed environment, maintaining accountability across teams can be challenging. Organizations need to establish clear guidelines for AI implementation that align with compliance standards and ensure all team members understand their roles and responsibilities.
- Keeping Pace with Technological Change: The rapid pace of technological advancement in AI often outstrips the learning curve of teams. As a professor from Columbia University noted, “Things are moving too rapidly in the world of AI; to remain relevant, we must teach our students how to learn and fail faster.” This represents one of the most significant challenges organizations will encounter: upskilling their global workforce to adapt to new roles that arise as routine tasks become AI-driven. This is one of the most formidable challenges that organizations would face to upskill their global workforce to adapt to new roles that emerge as routine tasks become AI oriented.
With your expertise in enterprise automation, how do you see generative AI reshaping organizational workflows and decision-making processes over the next few years?
With my expertise in enterprise automation, I see generative AI significantly reshaping organizational workflows and decision-making processes in several key ways over the next few years. We’re entering a new era where, traditionally, we have trained Large Language Models (LLMs) using tokens. Moving forward, while tokens will still play a crucial role, the quality of these tokens and the mechanism to acquire these tokens & train models will also evolve and would be in real time through human interactions via our devices or sensors enabled through these devices. This shift will enhance the models’ capabilities by integrating immediate human insights and examples, leading to more dynamic and responsive AI systems that will lead to reshaping organizational workflows & lead to faster and improved decision-making. In healthcare, clinicians will harness AI-powered diagnostic systems that analyze patient data in real-time, delivering personalized treatment plans and enhancing patient outcomes. In the oil and gas industry, analysts will use AI to assess seismic data instantaneously, leading to greater accuracy in resource extraction decisions. Meanwhile, in chip manufacturing, engineers will implement AI-driven automation to improve precision during the fabrication process, significantly reducing defects and increasing yield rates. Process engineers will also employ AI for real-time monitoring of production lines, enabling swift adjustments that optimize performance and minimize waste. The potential applications are limitless.
Data quality often poses significant challenges in AI adoption. How do you approach building robust data foundations while maintaining momentum in digital transformation?
When discussing data in the industry, we often reference the 5 V’s: Variety, Velocity, Veracity, Volume, and Value. These elements are crucial in understanding the complexities and challenges of data management, especially in the context of AI adoption. Each “V” plays a significant role in shaping how organizations approach their data foundations, and addressing these factors is essential for unlocking the true potential of data-driven insights.I approach building data quality by establishing robust data governance policies and fostering a culture of accountability where all stakeholders are responsible for maintaining data integrity. To ensure effective data quality for AI implementation, I prioritize discovering and cleansing data early in the process. By identifying inaccuracies and irrelevant information from the outset, I set a solid foundation for my AI models. I also establish and enforce stringent data quality standards, defining metrics for accuracy, completeness, consistency, and timeliness. Customizing these standards specifically for AI initiatives allows me to align my data strategies with the unique demands of AI technologies. Continuous monitoring is essential, as it helps me assess data quality over time and address any issues promptly. Lastly, I foster a data-driven culture within my team, empowering everyone to take ownership of data quality and understand its importance in driving effective AI outcomes.
Looking ahead, what skills and competencies should organizations prioritize to stay competitive in an AI-driven future?
It’s important to acknowledge that for organizations to succeed in an AI-driven future, they must prioritize developing key skills and competencies across the various layers of their workforce, namely Strategic, Application, Execution, and Support. In the Strategic Layer, it is essential to cultivate AI-enabled executives who can guide the organization in leveraging AI for strategic advantage. This includes having AI Translators who bridge the gap between technical capabilities and business needs, as well as establishing expertise in AI Governance and Ethical Oversight to ensure compliance and uphold ethical standards. In the Application Layer the focus is on enhancing skills in AI Model and Prompt Engineering to effectively design and optimize AI systems, particularly large language models (LLMs). In the Execution Layer, proficiency in Data Science is vital for extracting meaningful insights from data, alongside strong Design and Coding skills for implementing and managing AI solutions in daily operations.Additionally, prioritizing Data Quality and Bias Analysis is crucial for ensuring that AI outputs are reliable and free from bias.
Finally, the Support Layer plays a critical role in maintaining the overall effectiveness of AI initiatives. This includes providing ongoing training and resources to ensure that employees are equipped to setup AI infrastructure , use AI tools effectively, as well as establishing robust support systems for troubleshooting and enhancing AI applications. By addressing all these skills across different layers, organizations can create a comprehensive framework that maximizes the benefits of AI and drives innovation.
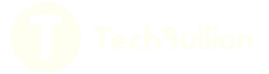