Today we will be discussing the future of artificial intelligence (AI) and machine learning (ML) and how that impacts businesses with Lucas Bonatto Miguel, founder and CEO of Elemeno AI.
What is Elemeno and what unique solutions do you provide at Elemeno?
Elemeno is a machine learning (ML) infrastructure provider. We offer a variety of cloud services that simplify the adoption of artificial intelligence (AI) across multiple industries. One example is our managed MLOps stack, which empowers companies with the best tools for developing AI in the most efficient way.
Could you give us an overview of the machine learning technology market and the most prevalent trends?
It is a market that is experiencing disruption. ML technology is not something new, however, it’s just recently being adopted by mainstream businesses. There are many opportunities to make the ML creation process easier within companies transitioning to the scientific field. Things happen at a different pace in research stage, and the urge for quick project turnarounds is a must for ML to become more popular.
How does your MLOps platform work, could you give us a walkthrough of the Elemeno ecosystem?
Our approach is to put the data scientist in the center. This guides how we develop our user experience and optimizes some aspects of how the teams are operating within companies. As much of the time spent in ML projects has to do with data preparation, we offer a variety of plugins and tools to make it easier to perform feature retrieval and engineering.
Once the user has built their data pipelines, we offer a managed development environment that helps the data scientist to adhere to the best engineering practices without necessarily needing to learn how to become a software engineer. We also give special attention to performance and simplifying complex use cases. ML usually implies using distributed computing and deploying high-performance microservices that can serve the model in high-throughput situations, and our tool makes both of these tasks less painful.
Why is MLOps becoming increasingly crucial to the successful implementation of data science projects?
I would not say it’s crucial, but it’s definitely something companies should ask themselves if they should be doing. MLOps is rooted in the DevOps techniques that are proven to make companies agile. Before mass adoption of DevOps techniques, most companies needed help to produce software. I like to think of it as a production line; if you’re running a car factory and you don’t have the right tools and processes in place, chances are that you will not be able to produce cars decently. You will not only move slower than your competitors but you will also be more prone to mistakes that can have significant implications in your customers experiences when driving the car. MLOps is crucial for any company who needs to constantly develop and maintain new ML models. If you’re only building an auxiliary model and you can predict your business will not make regular use of ML, you could do well without the overhead of MLOps.
What impact is Elemeno making and what makes it unique and special, any success stories from the community so far?
Our impact often has to do with the democratization of technology. Elemeno specializes in MLOps, but we are building solutions for companies at different levels of AI maturity. Our MLOps make it easier for us to build models that can solve problems shared by many companies. We assume that not every company may need a data science team, but in the near future every company will need data science. One of the most important features in our roadmap is a model marketplace platform. We’re aiming to connect ML developers worldwide with the company’s needs, and by providing the right infrastructure for development, production and monetization, we think we could amplify the reach of the experts in the field.
Who are the brains behind this platform, could you tell us about your team and customer support?
We’re a small team still, but we have been constantly delivering software and ML models. I founded Elemeno after several years of experience helping companies organize their ML development workflows. I’ve been exposed to solving these sorts of problems for multiple companies in the online retail space, which has offered me the opportunity to deploy models to serve web apps receiving hundreds of thousands of inference requests per minute. Our company was founded during the lockdowns of covid, so we have started fully distributed. I have a base in San Francisco – CA, but our Lead scientist, Ahmed Asadi, stays in Dallas – TX. His team is distributed in the US and Brazil.
Do you have any available opportunities for career investors for partnerships at Elemeno?
We currently have job openings for ML engineers and data engineers. The journey at Elemeno involves dealing with complex problems with, in most cases, unknown solutions. So it is a very good environment for those looking to challenge themselves technically. I like this kind of project because you not only help customers solve practical problems, but you learn lots of new technologies and enhance your problem-solving skills. I encourage anyone who identifies with these challenges to send us a message and start the conversation.
What are you currently working on and what is next on yoru roadmap, do you have more information for our readers today?
Our priority currently has been around adding new features to our MLOps cloud offering. It is a generic platform, so for every new customer we onboard we make sure that we adapt the product and make it attend new use cases. We have recently added support for NoSQL data sources in our feature store, such as Elasticsearch. Another upcoming feature that I am really excited about is the ability to handle semi-structured/binary files in the feature store. We noticed that many of our customers have pipelines that involve all sorts of different files, like image, video and PDF. And finding a feature store that allows you to have access to these files easily and, at the same time, ensure governance and organization is challenging. Once we launch the binary feature store, our users will be able to train these types of models with the minimum friction possible.
For more information, visit the website: https://www.elemeno.ai/
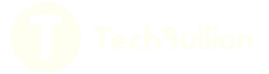