Data is a catalyst for business optimization, but it’s becoming a great problem to analyze large, different by nature and complex data sets.
Why Use Machine Learning for Big Data Analytics?
Just a few years ago, the ideas of big data and cloud services revolutionized the IT business, and then among other realms of business. Data scientists and executives from a wide variety of sectors grasped its opportunities and how AI-based big data collection and analysis are conducive to business success.
And it’s at this point where machine learning models come in handy. To thrive in the digital age, businesses need to arm their data analytics with powerful tools tailored to their needs. They can also try adopting artificial intelligence and machine learning for business success, and see how it goes.
What is Machine Learning?
Machine learning (ML) is a set of mathematical methods that allow you to get a solution to some problems using general algorithms that aren’t written specifically for the problem being solved.
Simply put, it’s a way to put big data analytics on stream. With the use of this technology, you teach a computer to identify certain patterns, facing which, it will perform certain actions – buying or selling securities, segmenting potential customers, or identifying defective products on the conveyor.
The major idea is that ML models are absolutely universal and are not at all tied to a specific business or branch of the economy. Monitoring the customer base and determining the churn rate, analyzing the traffic flow in the city and the likely places of congestion, the chance of a defect in the production industry, and more – all these tasks can be solved using the same mathematical system.
Applications of Big Data Analytics with Machine Learning
Leveraging ML to analyze large data sets can optimize your business operations in a number of ways. Let’s consider the major benefits that businesses reap from it:
Predictive Analytics
Slow decision-making is an aspect that handicaps the organization and allows competitors to have an upper hand. To solve it, AI technologies are capable of processing large amounts of data, identifying patterns, and making predictions swiftly. Knowing the expectable market trends or customer behavior, ML provides better data-driven business decisions.
Artificial intelligence performs well in forecasting due to its self-learning ability. Unlike traditional types of forecasting, predictive analytics can easily adapt to changes – the more information it gets, the better it functions.
Market Research
Predicting consumer behavior is one of the biggest challenges faced by specialists around the world. Companies conduct market research regularly to understand key customer attitudes, opinions, behavior, and solutions to provide a better customer experience and top-notch service.
However, traditional methods put limits on comprehensive research due to time, cost, and effort restrictions. ML combined with historical data analysis and accumulated experience can solve a huge variety of problems. It streamlines business operations since its algorithms conduct qualitative research in fewer steps, saving significant time, money, and research effort.
A vivid example – product recommendations by Netflix which analyzes customer behavior and offers personalized products. McKinsey estimates that 75% of movies watched on Netflix come from product recommendations making it conducive to the overall income.
Fraud Detection
According to the Association of Certified Fraud Examiners, an average fraud costs an organization more than $1.5 million. With most of the data stored in clouds and online, more companies are susceptible to cyber-attacks. Analyzing data with ML can become a go-to choice in this issue.
Implementing ML algorithms can speed up fraud analysis, increase fraud detection rate, and help identify and fix weaknesses in the system or business flow. In this way, fraud detection with InData Labs big data analytics implementing and machine learning allows companies to detect, prevent, predict, and remediate fraud quickly and more efficiently.
Keep In Mind
There are some prerequisites that determine how successful the integration of ML will be. Keep an eye on the following factors:
- Confirm the quality and completeness of your data to prevent losses from incorrect data analysis;
- Before embedding ML, experiment with real instead of computer-generated data;
- Make sure you know what you want to achieve by analyzing data with machine learning.
Wrapping Up
Data science has a significant impact on business efficiency. So embedding machine learning into big data analytics will be cost-effective for both large and small businesses to make the decision-making process more accurate.
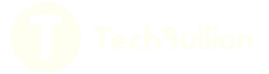