In today’s digital era, generative AI is revolutionizing credit risk assessment, offering a dynamic approach to financial decision-making. As Teena Choudhary explains, this technology surpasses traditional methods by generating synthetic data, enhancing predictive accuracy, and fostering financial inclusivity. By simulating complex scenarios and analyzing broader datasets, generative AI allows institutions to make better-informed decisions, ultimately transforming the credit evaluation process and improving access to financial services.
Redefining Credit Risk Assessment with Generative AI
The financial industry is experiencing a major shift as generative AI transforms credit risk assessment. Unlike traditional static models, generative AI, especially Generative Adversarial Networks (GANs), introduces dynamic simulations of diverse financial scenarios. This innovation enhances predictive accuracy, speeds up decision-making, and supports a more inclusive financial system. By generating realistic synthetic data, generative AI helps financial institutions fill gaps in real-world information, enabling them to analyze broader datasets and reduce uncertainty for new or underbanked borrowers.
Bridging Data Gaps with Synthetic Data
Generative AI’s ability to create synthetic data is a major breakthrough for credit risk assessment, addressing the challenge of data scarcity, particularly for underbanked populations. By generating realistic financial profiles, AI enhances predictive models’ robustness and helps fill data gaps. This leads to better-informed decisions, especially in emerging markets and for borrowers with limited credit histories. Additionally, synthetic data improves accuracy and prevents overfitting, ensuring more reliable and unbiased outcomes in financial assessments.
Predictive Power at Scale
Generative AI models excel at simulating complex economic conditions, enabling financial institutions to stress-test credit scenarios and assess borrower resilience in fluctuating markets. These advanced models analyze patterns and correlations in financial data with greater speed and accuracy than traditional systems. Their enhanced precision improves early warning systems for credit defaults by detecting anomalies in financial behavior, allowing institutions to identify potential risks early and take proactive measures, resulting in more agile risk management and deeper insights into borrower trends.
Personalized and Dynamic Credit Scoring
Generative AI enables personalized credit risk assessments, going beyond traditional static metrics like credit scores. It incorporates alternative financial behaviors, non-traditional data sources, and real-time economic changes, offering a more comprehensive view of each borrower’s risk profile. This adaptability leads to more accurate, fairer credit decisions and creates opportunities for individuals often overlooked by conventional models. By tailoring assessments to individual financial realities, AI plays a key role in promoting financial inclusion and expanding access to credit for underserved populations.
Automation and Efficiency in Credit Processes
Generative AI not only improves accuracy but also transforms the speed and efficiency of credit risk assessments. By automating data processing, it enables financial institutions to manage large volumes of data in real time. Tasks like risk assessment updates and report generation, once manual, are now automated, allowing teams to focus on strategic work. This automation reduces operational costs, accelerates decision-making, and provides a crucial advantage in rapidly changing financial conditions by ensuring real-time risk monitoring and updates.
Overcoming Implementation Challenges
Generative AI’s integration into credit risk assessment presents challenges, particularly in data privacy and security. Handling sensitive financial information requires advanced encryption and strict adherence to data protection regulations. Techniques like differential privacy are key to generating synthetic data while maintaining confidentiality. Another issue is model interpretability; AI models, though accurate, can be complex and difficult to explain, raising regulatory and trust concerns. Explainable AI (XAI) methods help make decision-making processes more transparent. Lastly, bias in AI models is a concern. Ensuring diverse and representative data during training, conducting regular bias audits, and adhering to ethical AI frameworks are essential for promoting fairness and avoiding harm to underserved populations.
The Road Ahead: A Transformative Financial Landscape
Generative AI’s impact on credit risk assessment is only the beginning of broader transformations in the financial sector. Its potential to democratize access to credit, reduce default rates, and optimize capital allocation promises a more inclusive and resilient financial ecosystem. With ongoing advancements, AI is poised to continue reshaping the financial landscape, enabling more dynamic, efficient, and fair decision-making processes.
As Teena Choudhary concludes, the future of generative AI in credit risk assessment is not just about technological advancement but also about responsibly harnessing this power for the betterment of financial services. The collaboration between technologists, regulators, and financial experts will be key in ensuring that AI serves as a force for good in the global economy.
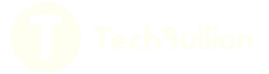