AI has had a spectacular year in 2023 and its affecting many industries. While much of the focus is on consumer applications (B2C), AI’s potential in business-to-business (B2B) contexts is underestimated and relatively unexplored.
This article explores AI’s readiness for enterprise development, looking into its transformative potential for businesses, as well as its risks.
B2C vs. B2B AI Developments
AI development has significantly impacted both B2C and B2B sectors, but with notable differences. B2C applications, such as virtual assistants and recommendation systems, are highly visible and directly enhance consumer experiences. Language Learning Models (LLMs) like ChatGPT and Claude have essentially been marketed towards customers. We know this because ChatGPT was the fastest company ever to reach 5 million users (in 5 days) – that’s hardly the behavior of an enterprise-focused company.
As a result, both workers and businesses alike have begun figuring out how to use this B2C product to their advantage (and without breaking data privacy laws). Some of the more common B2B solutions have been focused on customer service, such as chatbots, which existed prior to the AI renaissance that we saw last year.
Current State of AI in Enterprises
In finance, AI helps predict market trends and manage risks – it’s very good at recognizing patterns, so it’s also being used for fraud detection. Healthcare uses AI for diagnostic tools and personalized medicine – but one only needs to take a visit to Bangkok hospital to see self-driving robots delivering items all around the building. Manufacturing benefits combine these, as they use AI for predictive maintenance supply chain optimization (with the increase in IoT, there’s much more data to explore), but equally it’s used for on-floor operations.
Despite these advances, the full potential of AI in enterprises is yet to be realized. Sometimes, there’s a fine line between algorithms that have touched on Machine Learning, and full-blown neural networks and LLMs, which indicates room for growth.
Customizability and Potential of AI for Enterprises
Customizing AI solutions is crucial for enterprises. Generic AI tools may not address specific business needs, whereas tailored solutions can significantly enhance efficiency and competitiveness. For instance, Harry Potter’s Wizarding World recently outsourced AI development services to create bespoke gesture-recognition so visitors could wave their arms around and cast spells. Of course, such a thing requires bespoke computer vision and image processing – it’s something that cannot be off-the-shelf.
For more ordinary enterprise solutions, these can simply be automating routine tasks, provide predictive analytics, or even supporting strategic decisions. For IT departments, it may be finding a customised AI assistant to run tests faster and debug, but with the context of their current infrastructure. Ultimately, though, it has the potential to be a point of differentiation to the customers.
This level of customization ensures that AI tools align closely with business objectives, thus maximizing their impact. Off-the-shelf AI tools are still useful, but they’re not going to provide a competitive advantage (because competitors are using them too, and with identical results), and they’re not going to fit perfectly to your needs.
Enterprise-level AI solutions will also become more important regarding data privacy. One of the biggest hurdles is handing over sensitive data to companies like OpenAI, where in theory, it’s possible (and known to) have spit such information back out to other customers. Therefore, enterprise solutions will be focused on local models, or obscuring current ones enough to ensure you’re fully compliant.
Challenges in Enterprise AI Adoption
Adopting AI in enterprises presents several challenges. Technically, integrating AI with existing systems can be complex, requiring significant expertise and quality data engineering. It’s also a matter of repeatability – an LLM may help a programmer with some tasks, but can this be reliably replicated to work on its own, every time, with the same results? By nature, LLMs are not very repeatable, meaning further iterations and frameworks are required.
Organizational challenges include resistance to change, as employees may be wary of new technologies, or are simply unsure of what they’re capable of.
Emerging Trends and Solutions
Several emerging trends in AI are poised to benefit enterprises. One is that AI tools are being used to help speed up the building of IT infrastructure and systems, even for beginners. For example, Zapier is a drag-and-drop workflow automation tool; you can build complex processes. But now, with the integration of a text field, you can write down roughly what you want to build in plain, non-technical language, and it will then organize for itself what the order of logic is and self-build. This democratization of AI technology allows more enterprises to adopt and benefit from AI, whether they build it themselves, or pay less money for the same solution due to the gains in productivity. Therefore, even if indirectly, all companies may benefit from lower prices for evergreen solutions.
Conclusion
AI is ready for enterprise development, but it’s presenting some compliance obstacles. As B2B companies figure out how to remain compliant, as well as build reliable systems that produce repeatable results, more sophisticated solutions can be provided to companies. Alternatively, non-technical owners can access customer-facing AI solutions to cut costs and improve upon productivity.
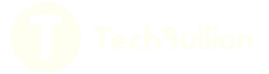